A Neural Network Estimation Of Ankle Torques From Electromyography And Accelerometry
IEEE TRANSACTIONS ON NEURAL SYSTEMS AND REHABILITATION ENGINEERING(2021)
摘要
Estimations of human joint torques can provide clinically valuable information to inform patient care, plan therapy, and assess the design of wearable robotic devices. Predicting joint torques into the future can also be useful for anticipatory robot control design. In this work, we present a method of mapping joint torque estimates and sequences of torque predictions from motion capture and ground reaction forces to wearable sensor data using several modern types of neural networks. We use dense feedforward, convolutional, neural ordinary differential equation, and long short-term memory neural networks to learn the mapping for ankle plantarflexion and dorsiflexion torque during standing, walking, running, and sprinting, and consider both single-point torque estimation, as well as the prediction of a sequence of future torques. Our results show that long short-term memory neural networks, which consider incoming data sequentially, outperform dense feedforward, neural ordinary differential equation networks, and convolutional neural networks. Predictions of future ankle torques up to 0.4 s ahead also showed strong positive correlations with the actual torques. The proposed method relies on learning from a motion capture dataset, but once the model is built, the method uses wearable sensors that enable torque estimation without the motion capture data.
更多查看译文
关键词
Torque, Wearable sensors, Estimation, Recurrent neural networks, Legged locomotion, Feedforward neural networks, Dynamics, Accelerometers, biomechanics, electromyography, neural networks, wearable sensors
AI 理解论文
溯源树
样例
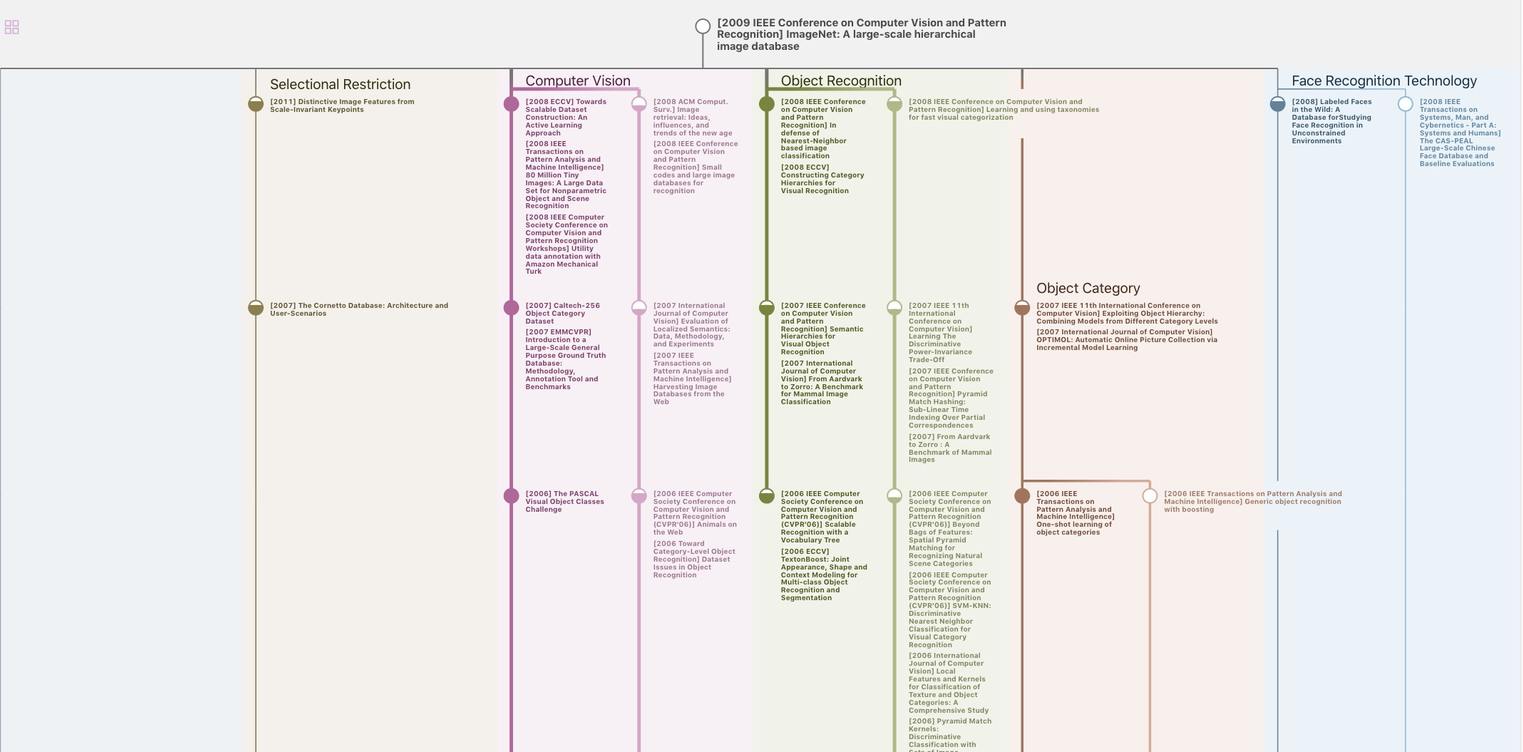
生成溯源树,研究论文发展脉络
Chat Paper
正在生成论文摘要