How to Compare Summarizers without Target Length? Pitfalls, Solutions and Re-Examination of the Neural Summarization Literature
user-5edf3a5a4c775e09d87cc848(2019)
摘要
We show that plain ROUGE F1 scores are not ideal for comparing current neural systems which on average produce different lengths. This is due to a non-linear pattern between ROUGE F1 and summary length. To alleviate the effect of length during evaluation, we have proposed a new method which normalizes the ROUGE F1 scores of a system by that of a random system with same average output length. A pilot human evaluation has shown that humans prefer short summaries in terms of the verbosity of a summary but overall consider longer summaries to be of higher quality. While human evaluations are more expensive in time and resources, it is clear that normalization, such as the one we proposed for automatic evaluation, will make human evaluations more meaningful.
更多查看译文
关键词
Automatic summarization,Normalization (statistics),Verbosity,Natural language processing,Computer science,Artificial intelligence,Neural system,Random systems
AI 理解论文
溯源树
样例
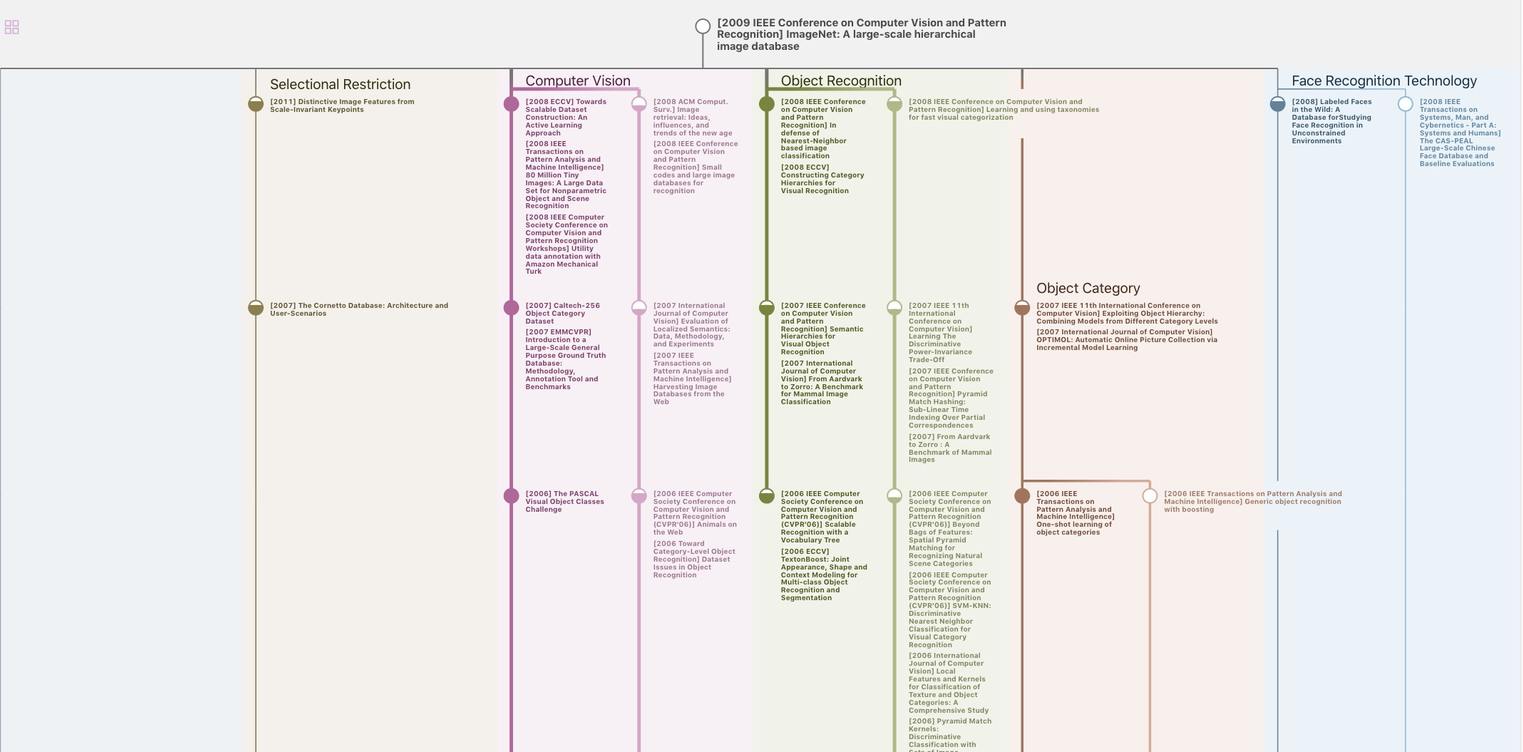
生成溯源树,研究论文发展脉络
Chat Paper
正在生成论文摘要