HiTab: A Hierarchical Table Dataset for Question Answering and Natural Language Generation
PROCEEDINGS OF THE 60TH ANNUAL MEETING OF THE ASSOCIATION FOR COMPUTATIONAL LINGUISTICS (ACL 2022), VOL 1: (LONG PAPERS)(2022)
摘要
Tables are often created with hierarchies, but existing works on table reasoning mainly focus on flat tables and neglect hierarchical tables. Hierarchical tables challenge table reasoning by complex hierarchical indexing, as well as implicit relationships of calculation and semantics. We present a new dataset, HiTab, to study question answering (QA) and natural language generation (NLG) over hierarchical tables. HiTab is a cross-domain dataset constructed from a wealth of statistical reports and Wikipedia pages, and has unique characteristics: (1) nearly all tables are hierarchical, and (2) questions are not proposed by annotators from scratch, but are revised from real and meaningful sentences authored by analysts. (3) To reveal complex numerical reasoning in analysis, we provide fine-grained annotations of quantity and entity alignment. Experimental results show that HiTab presents a strong challenge for existing baselines and a valuable benchmark for future research. Targeting hierarchical structure, we devise an effective hierarchy-aware logical form for symbolic reasoning over tables. Furthermore, we leverage entity and quantity alignment to explore partially supervised training in QA and conditional generation in NLG, which largely reduces spurious predictions in QA and meaningless descriptions in NLG. The dataset and code are available at https://github.com/microsoft/HiTab.
更多查看译文
关键词
language generation,question answering,hierarchical table dataset
AI 理解论文
溯源树
样例
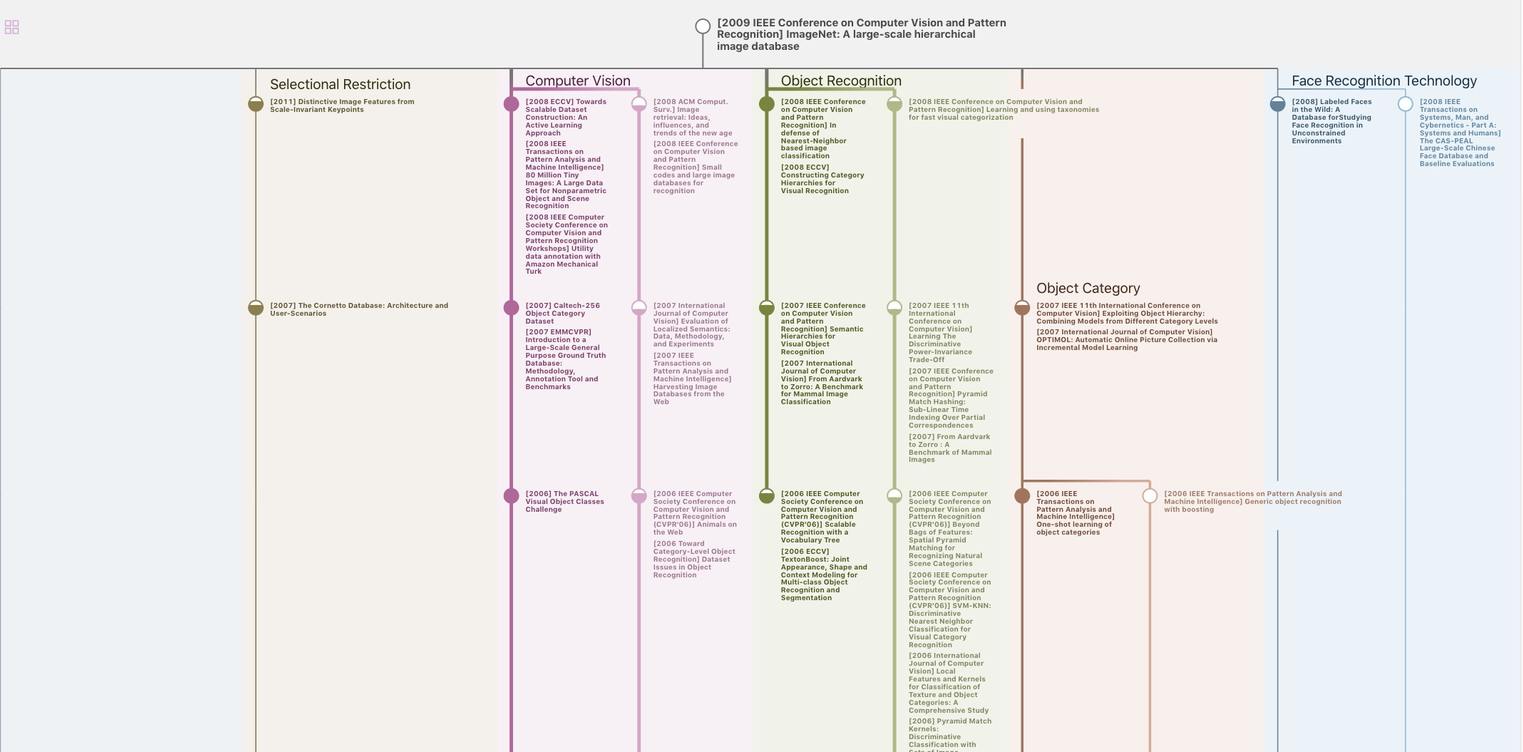
生成溯源树,研究论文发展脉络
Chat Paper
正在生成论文摘要