Aggregation Delayed Federated Learning
2022 IEEE International Conference on Big Data (Big Data)(2022)
摘要
Federated learning is a distributed machine learning paradigm where multiple data owners (clients) collaboratively train one machine learning model while keeping data on their own devices. The heterogeneity of client datasets is one of the most important challenges of federated learning algorithms. Studies have found performance reduction with standard federated algorithms, such as FedAvg, on non-IID data. Many existing works on handling non-IID data adopt the same aggregation framework as FedAvg and focus on improving model updates either on the server side or on clients. In this work, we tackle this challenge in a different view by introducing redistribution rounds that delay the aggregation. With delayed aggregations, local models are trained on data that are more representative to the global distribution. The proposed algorithm can also be used as a federated learning paradigm, as an alternative to FedAvg, where other methods can be plugged in. We perform experiments on multiple tasks and show that the proposed framework significantly improves the performance on non-IID data.
更多查看译文
关键词
federated learning,non-IID,heterogeneous data
AI 理解论文
溯源树
样例
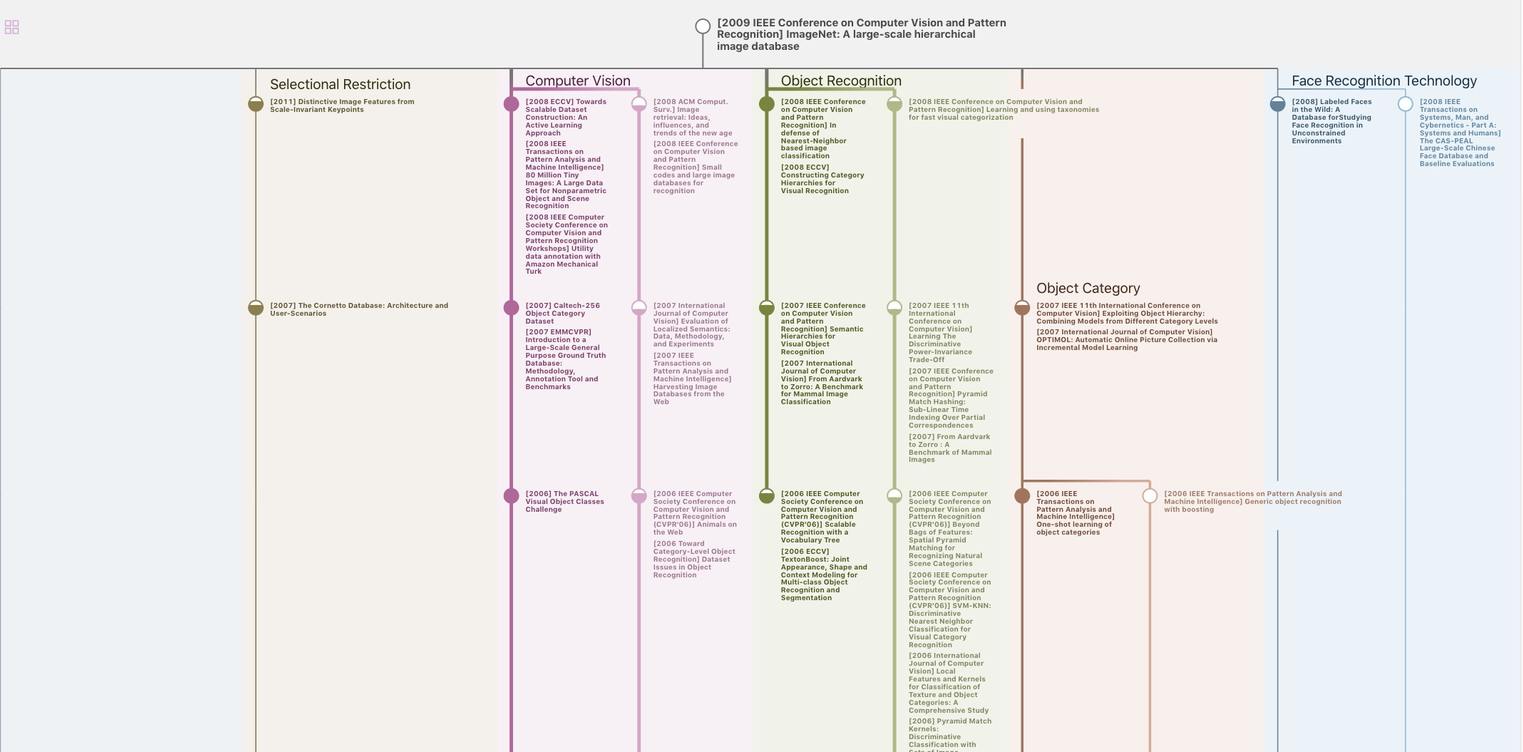
生成溯源树,研究论文发展脉络
Chat Paper
正在生成论文摘要