Augmenting Implicit Neural Shape Representations with Explicit Deformation Fields
arxiv(2021)
摘要
Implicit neural representation is a recent approach to learn shape collections as zero level-sets of neural networks, where each shape is represented by a latent code. So far, the focus has been shape reconstruction, while shape generalization was mostly left to generic encoder-decoder or auto-decoder regularization. In this paper we advocate deformation-aware regularization for implicit neural representations, aiming at producing plausible deformations as latent code changes. The challenge is that implicit representations do not capture correspondences between different shapes, which makes it difficult to represent and regularize their deformations. Thus, we propose to pair the implicit representation of the shapes with an explicit, piecewise linear deformation field, learned as an auxiliary function. We demonstrate that, by regularizing these deformation fields, we can encourage the implicit neural representation to induce natural deformations in the learned shape space, such as as-rigid-as-possible deformations.
更多查看译文
关键词
implicit neural shape representations,explicit deformation fields
AI 理解论文
溯源树
样例
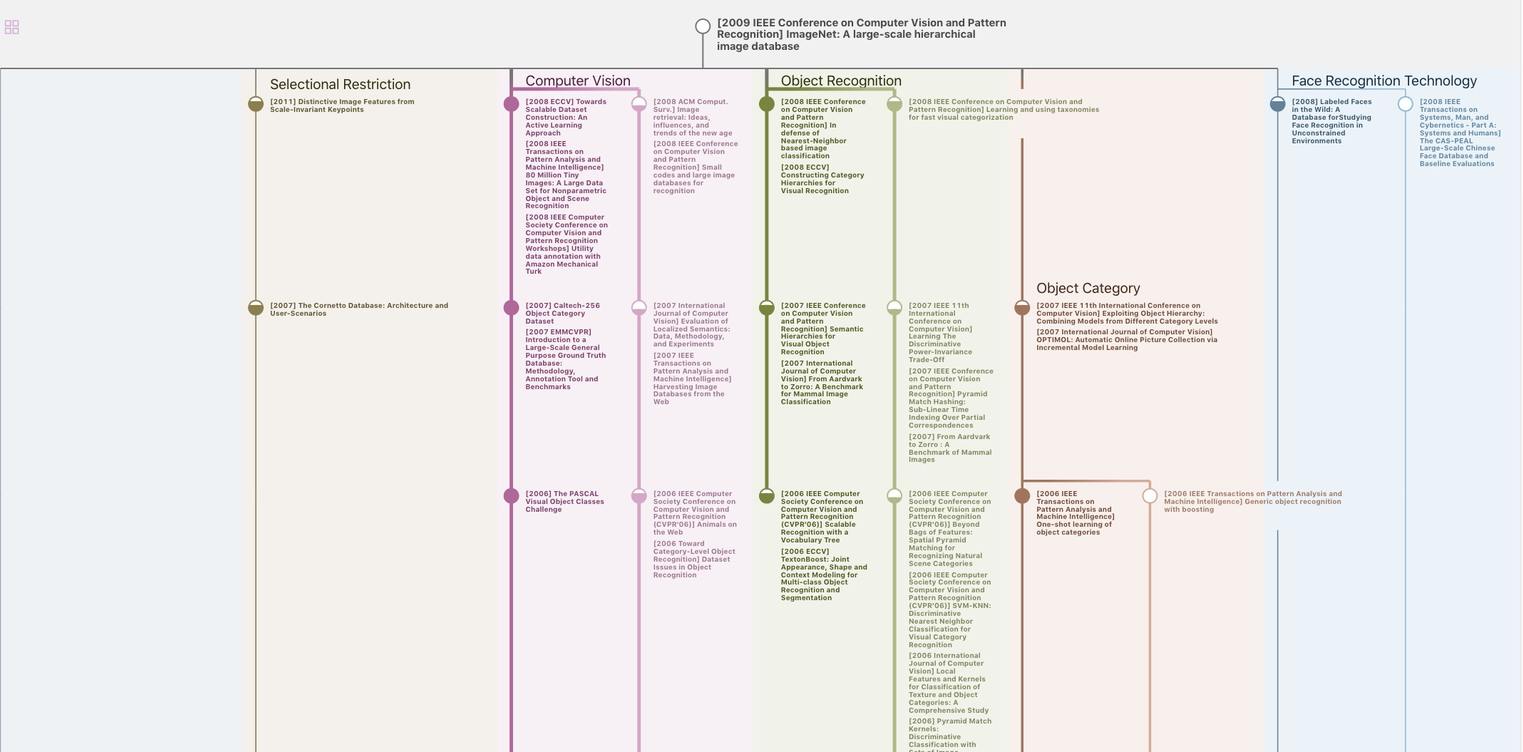
生成溯源树,研究论文发展脉络
Chat Paper
正在生成论文摘要