Mobility-Aware Cluster Federated Learning in Hierarchical Wireless Networks
IEEE Transactions on Wireless Communications(2022)
摘要
Implementing federated learning (FL) algorithms in wireless networks has garnered a wide range of attention. However, few works have considered the impact of user mobility on the learning performance. To fill this research gap, we develop a theoretical model to characterize the hierarchical federated learning (HFL) algorithm in wireless networks where the mobile users may roam across edge access points (APs), leading to incompletion of inconsistent FL training. We provide the convergence analysis of conventional HFL with user mobility. Our analysis proves that the learning performance of conventional HFL deteriorates drastically with highly-mobile users. And such a decline in the learning performance will be exacerbated with small number of participants and large data distribution divergences among users’ local data. To circumvent these issues, we propose a mobility-aware cluster federated learning (MACFL) algorithm by redesigning the access mechanism, local update rule, and model aggregation scheme. We also conduct experiments to evaluate the learning performance of conventional HFL, a cluster federated learning (CFL) with simple averaging, and our proposed MACFL. The results show that our MACFL can enhance the learning performance, especially for three different cases: (
$i$
) the case of users with non-independent and identically distributed (non-IID) data, (
$ii$
) the case of users with high mobility, and (
$iii$
) the case with a small number of users.
更多查看译文
关键词
Hierarchical federated learning,user mobility,data heterogeneity,convergence analysis
AI 理解论文
溯源树
样例
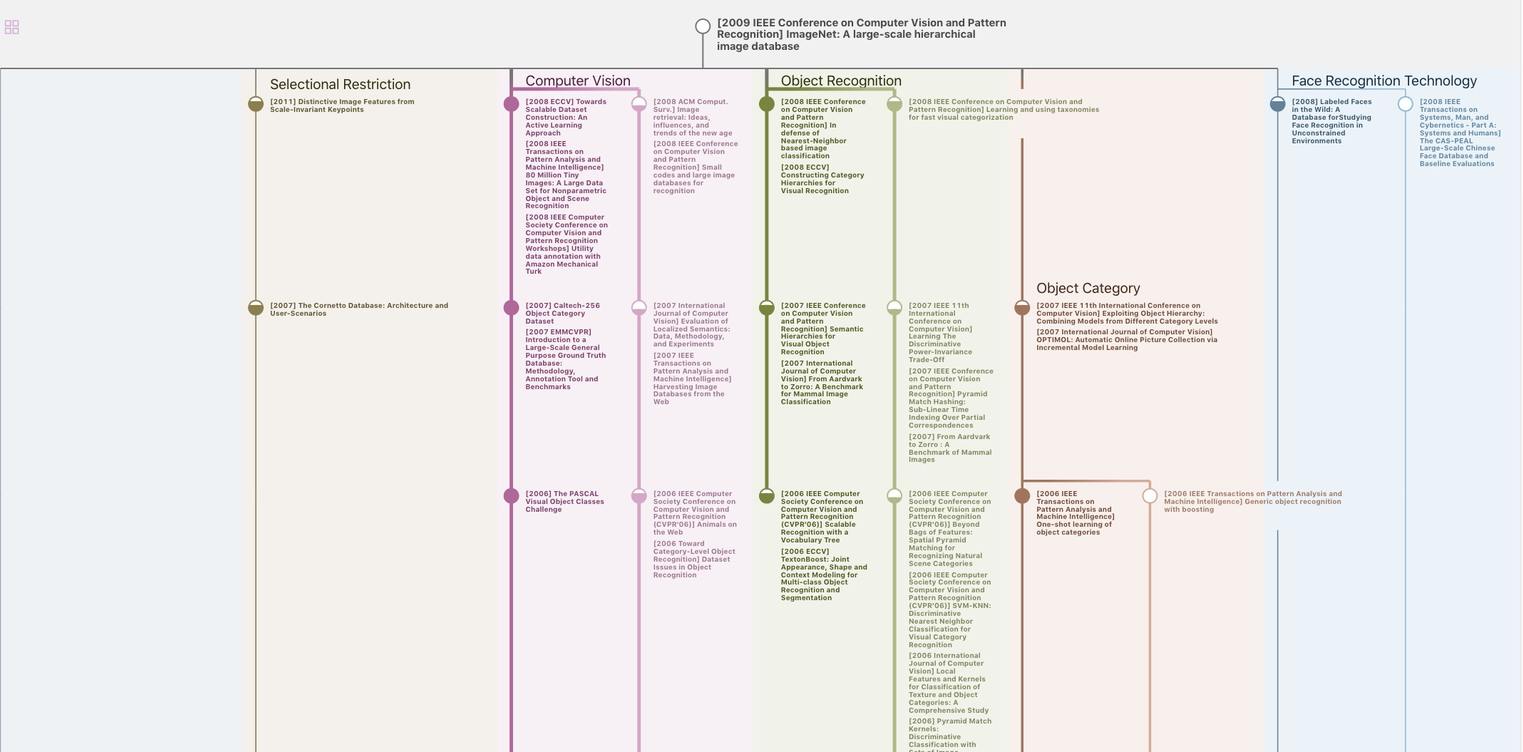
生成溯源树,研究论文发展脉络
Chat Paper
正在生成论文摘要