Region-level Active Learning for Cluttered Scenes
arxiv(2021)
摘要
Active learning for object detection is conventionally achieved by applying techniques developed for classification in a way that aggregates individual detections into image-level selection criteria. This is typically coupled with the costly assumption that every image selected for labelling must be exhaustively annotated. This yields incremental improvements on well-curated vision datasets and struggles in the presence of data imbalance and visual clutter that occurs in real-world imagery. Alternatives to the image-level approach are surprisingly under-explored in the literature. In this work, we introduce a new strategy that subsumes previous Image-level and Object-level approaches into a generalized, Region-level approach that promotes spatial-diversity by avoiding nearby redundant queries from the same image and minimizes context-switching for the labeler. We show that this approach significantly decreases labeling effort and improves rare object search on realistic data with inherent class-imbalance and cluttered scenes.
更多查看译文
关键词
cluttered scenes,learning,region-level
AI 理解论文
溯源树
样例
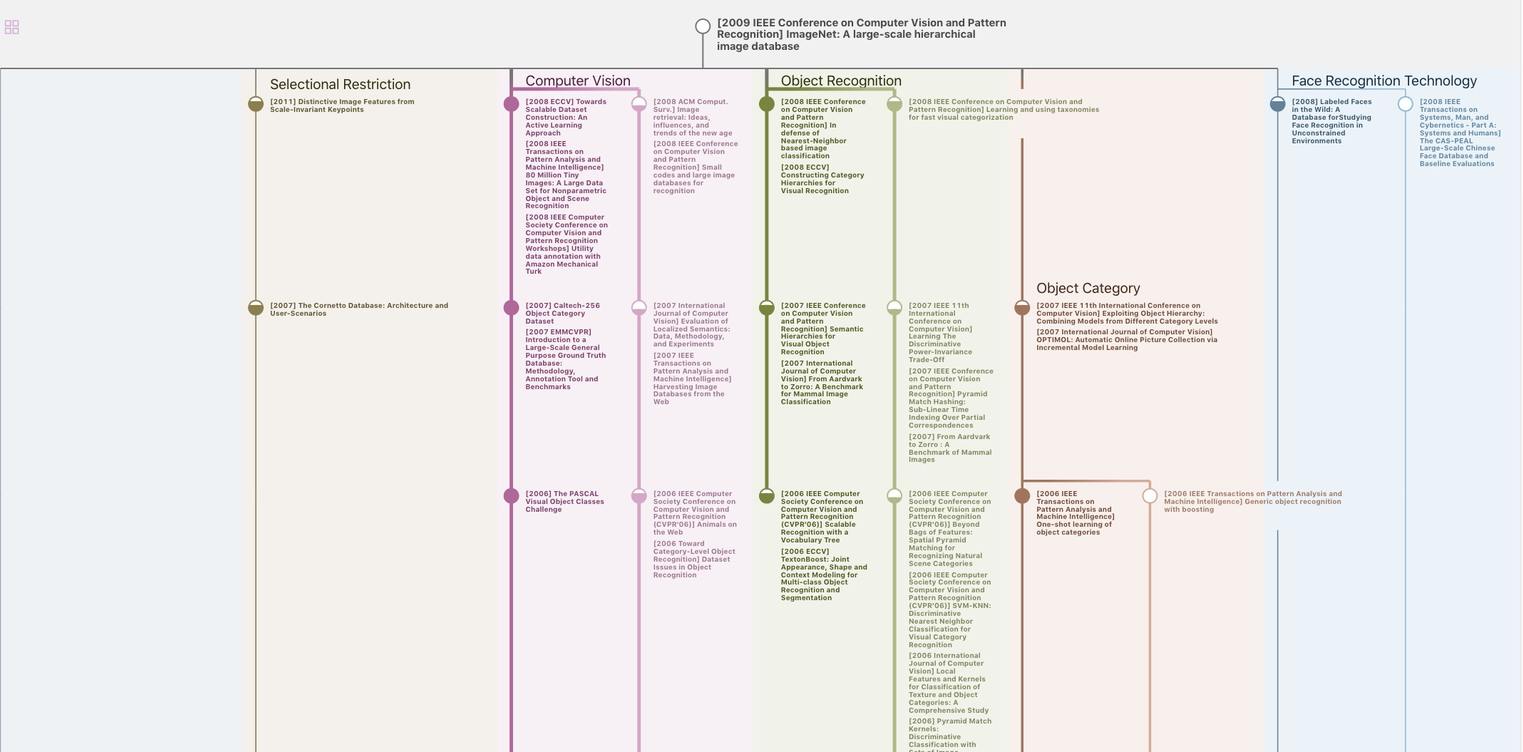
生成溯源树,研究论文发展脉络
Chat Paper
正在生成论文摘要