Talos: A Weighted Speedup-Aware Device Placement of Deep Learning Models
2021 IEEE 32nd International Conference on Application-specific Systems, Architectures and Processors (ASAP)(2021)
摘要
Efficient device placement of deep learning (DL) models, which consist of many operations, is a big challenge when heterogeneous devices (e.g., CPU, GPU) are considered. Existing average speedup and transient speedup approaches do not make full use of operation-level speedups, and the Total Operation Completion Time (TOCT) cannot be optimized efficiently. To address this challenge, we present Talos, a weighted speedup-awareness approach to optimize device placement of multiple DL models. Talos reveals operations within or across DL models have diverse speedups (from 10(-1) to 10(2)) on heterogeneous devices. In addition, the execution time of operations are widely ranged (from 0.1ms to 100ms). Talos considers the two features simultaneously as weighted speedups, and treats them as costs in an incremental minimum-cost flow. Compared with state-of-the-art efforts, experiment results show that Talos can reduce TOCT by up to 50%.
更多查看译文
关键词
deep learning models,device placement,heterogeneous devices,minimum-cost flow
AI 理解论文
溯源树
样例
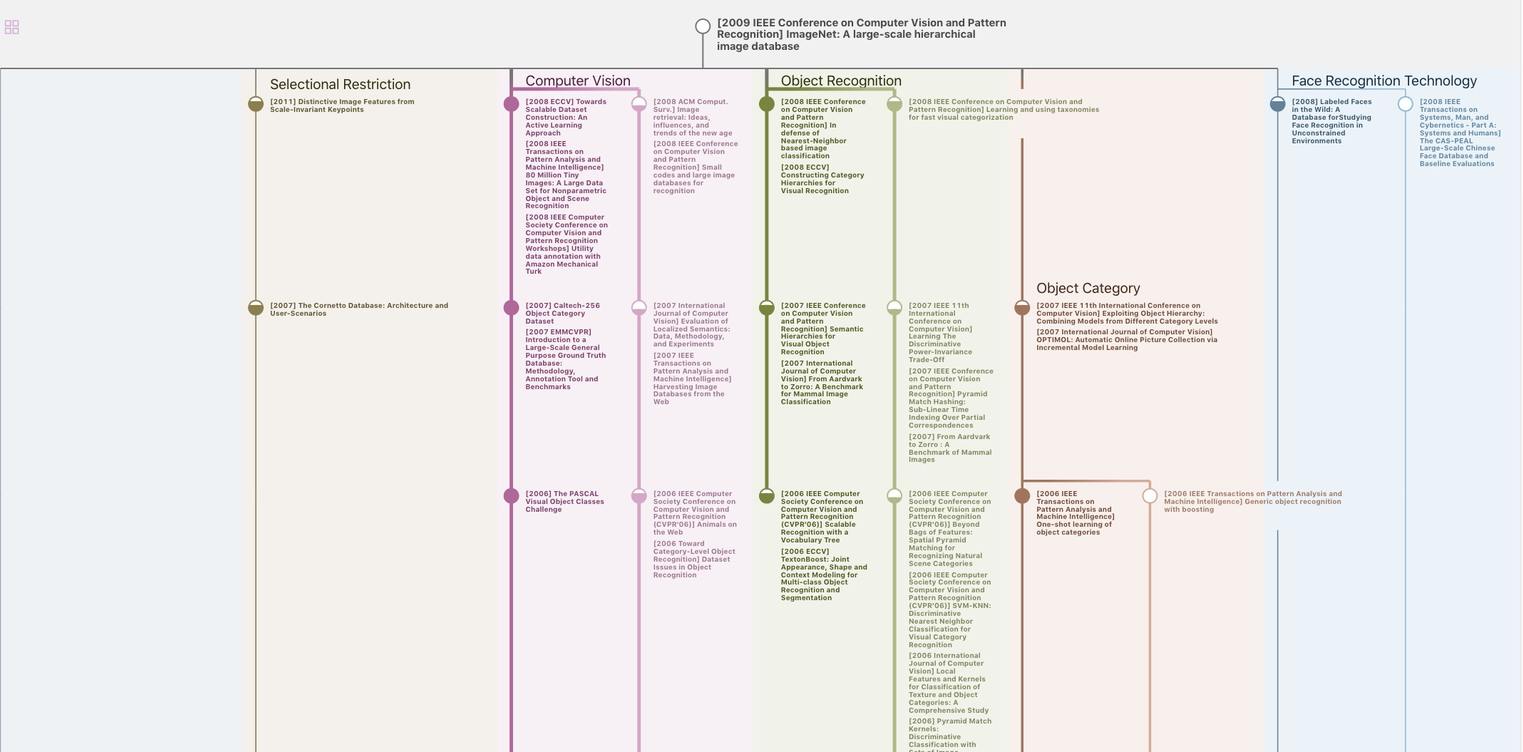
生成溯源树,研究论文发展脉络
Chat Paper
正在生成论文摘要