Comparison Of Traveling-Subject And Combat Harmonization Methods For Assessing Structural Brain Characteristics
HUMAN BRAIN MAPPING(2021)
摘要
Multisite magnetic resonance imaging (MRI) is increasingly used in clinical research and development. Measurement biases-caused by site differences in scanner/image-acquisition protocols-negatively influence the reliability and reproducibility of image-analysis methods. Harmonization can reduce bias and improve the reproducibility of multisite datasets. Herein, a traveling-subject (TS) dataset including 56 T1-weighted MRI scans of 20 healthy participants in three different MRI procedures-20, 19, and 17 subjects in Procedures 1, 2, and 3, respectively-was considered to compare the reproducibility of TS-GLM, ComBat, and TS-ComBat harmonization methods. The minimum participant count required for harmonization was determined, and the Cohen's d between different MRI procedures was evaluated as a measurement-bias indicator. The measurement-bias reduction realized with different methods was evaluated by comparing test-retest scans for 20 healthy participants. Moreover, the minimum subject count for harmonization was determined by comparing test-retest datasets. The results revealed that TS-GLM and TS-ComBat reduced measurement bias by up to 85 and 81.3%, respectively. Meanwhile, ComBat showed a reduction of only 59.0%. At least 6 TSs were required to harmonize data obtained from different MRI scanners, complying with the imaging protocol predetermined for multisite investigations and operated with similar scan parameters. The results indicate that TS-based harmonization outperforms ComBat for measurement-bias reduction and is optimal for MRI data in well-prepared multisite investigations. One drawback is the small sample size used, potentially limiting the applicability of ComBat. Investigation on the number of subjects needed for a large-scale study is an interesting future problem.
更多查看译文
关键词
ComBat, FreeSurfer, harmonization, MRI, multisite, traveling subject
AI 理解论文
溯源树
样例
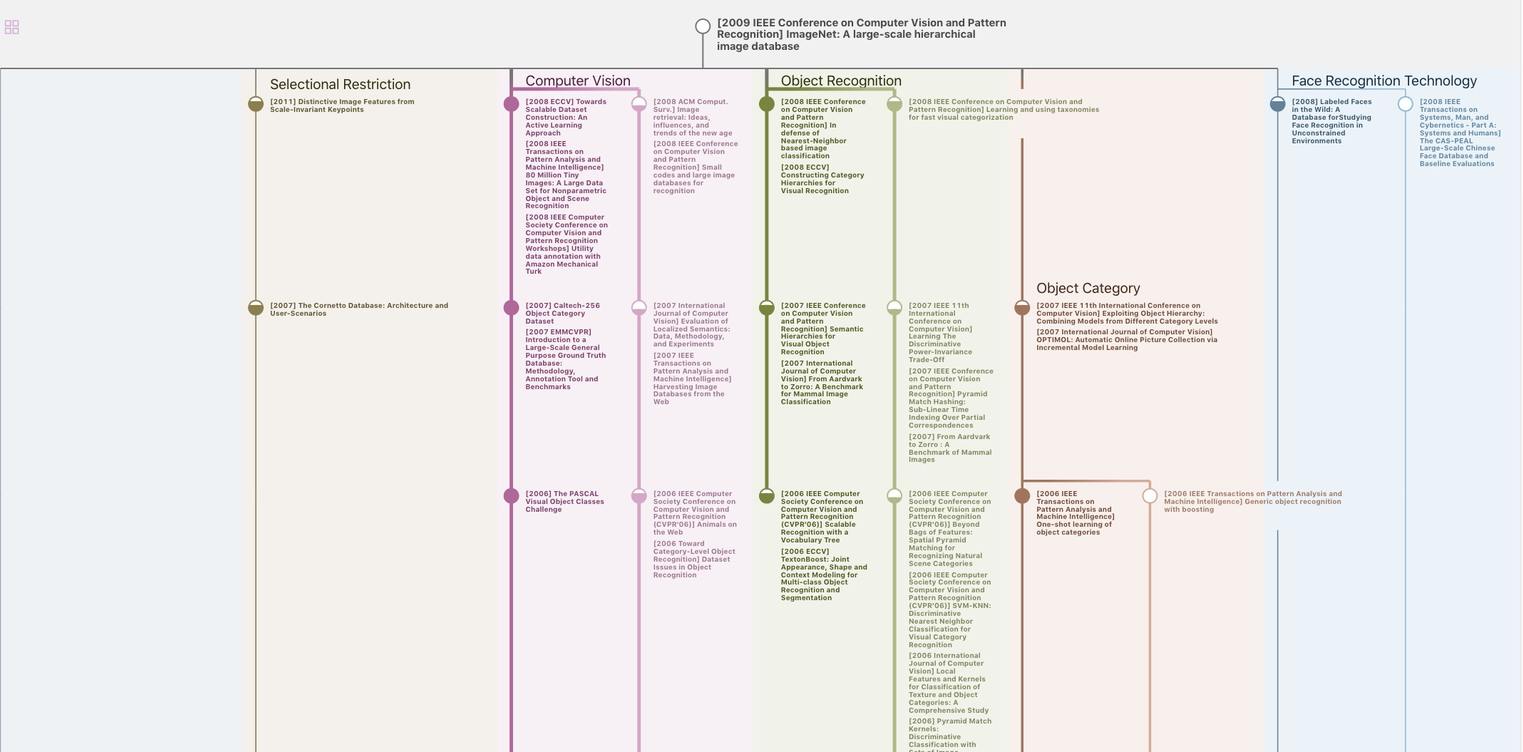
生成溯源树,研究论文发展脉络
Chat Paper
正在生成论文摘要