Two-stage sampling in the estimation of growth parameters and percentile norms: sample weights versus auxiliary variable estimation.
BMC medical research methodology(2021)
摘要
BACKGROUND:The use of auxiliary variables with maximum likelihood parameter estimation for surveys that miss data by design is not a widespread approach, despite its documented improved efficiency over traditional approaches that deploy sampling weights. Although efficiency gains from the use of Normally distributed auxiliary variables in a model have been recorded in the literature, little is known about the effects of non-Normal auxiliary variables in the parameter estimation.
METHODS:We simulate growth data to mimic SCALES, a two-stage survey of language development with a screening phase (stage one) for which data are observed for the whole sample and an intensive assessments phase (stage two), for which data are observed for a sub-sample, selected using stratified random sampling. In the simulation, we allow a fully observed Poisson distributed stratification criterion to be correlated with the partially observed model responses and develop five generalised structural equation growth models that host the auxiliary information from this criterion. We compare these models with each other and with a weighted growth model in terms of bias, efficiency, and coverage. We finally apply our best performing model to SCALES data and show how to obtain growth parameters and population norms.
RESULTS:Parameter estimation from a model that incorporates a non-Normal auxiliary variable is unbiased and more efficient than its weighted counterpart. The auxiliary variable method is capable of producing efficient population percentile norms and velocities.
CONCLUSIONS:The deployment of a fully observed variable that dominates the selection of the sample and correlates strongly with the incomplete variable of interest appears beneficial for the estimation process.
更多查看译文
AI 理解论文
溯源树
样例
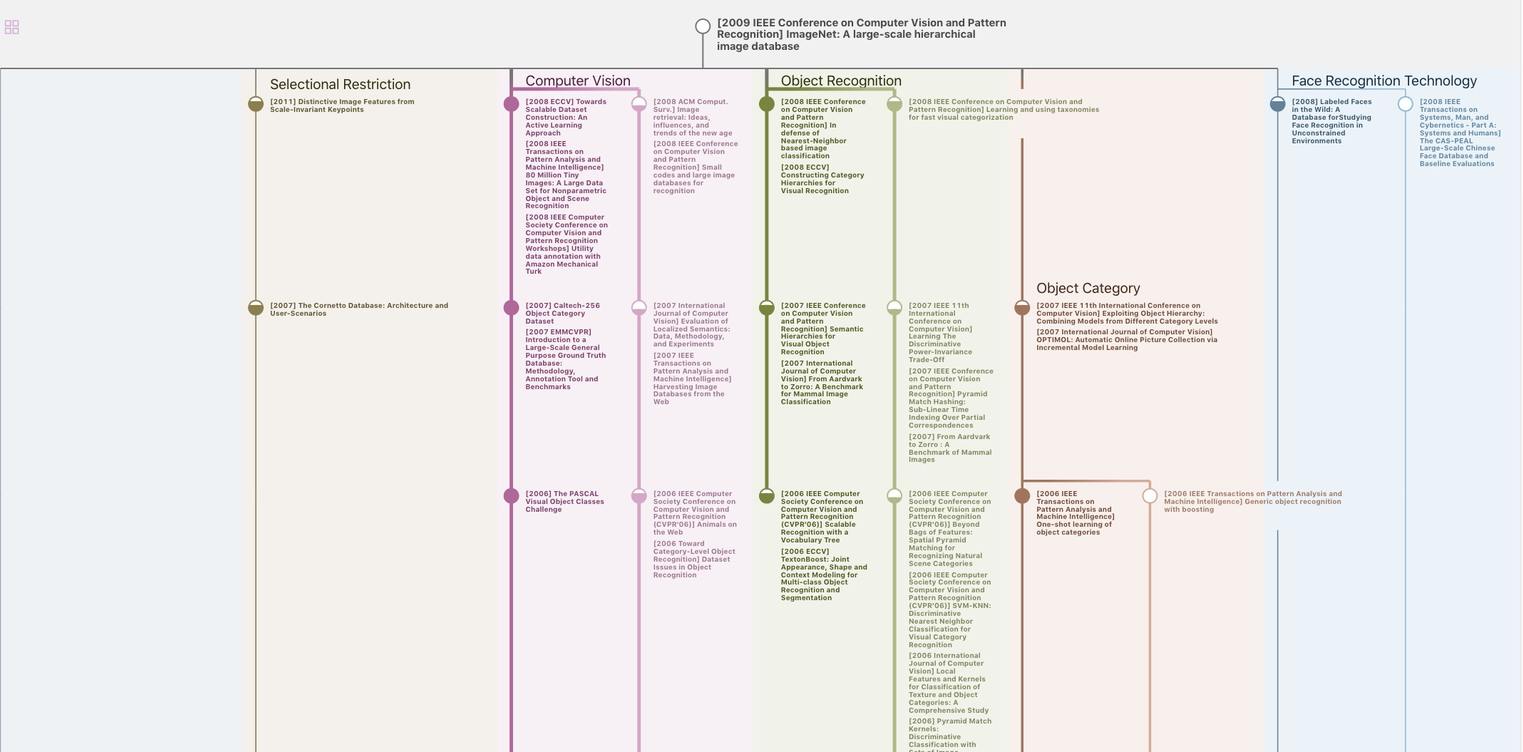
生成溯源树,研究论文发展脉络
Chat Paper
正在生成论文摘要