Improved datasets and evaluation methods for the automatic prediction of DNA-binding proteins
BIOINFORMATICS(2021)
摘要
Motivation: Accurate automatic annotation of protein function relies on both innovative models and robust datasets. Due to their importance in biological processes, the identification of DNA-binding proteins directly from protein sequence has been the focus of many studies. However, the datasets used to train and evaluate these methods have suffered from substantial flaws. We describe some of the weaknesses of the datasets used in previous DNA-binding protein literature and provide several new datasets addressing these problems. We suggest new evaluative benchmark tasks that more realistically assess real-world performance for protein annotation models. We propose a simple new model for the prediction of DNA-binding proteins and compare its performance on the improved datasets to two previously published models. In addition, we provide extensive tests showing how the best models predict across taxa. Results: Our new gradient boosting model, which uses features derived from a published protein language model, outperforms the earlier models. Perhaps surprisingly, so does a baseline nearest neighbor model using BLAST percent identity. We evaluate the sensitivity of these models to perturbations of DNA-binding regions and control regions of protein sequences. The successful data-driven models learn to focus on DNA-binding regions. When predicting across taxa, the best models are highly accurate across species in the same kingdom and can provide some information when predicting across kingdoms.
更多查看译文
关键词
automatic prediction,proteins,improved data sets,dna-binding
AI 理解论文
溯源树
样例
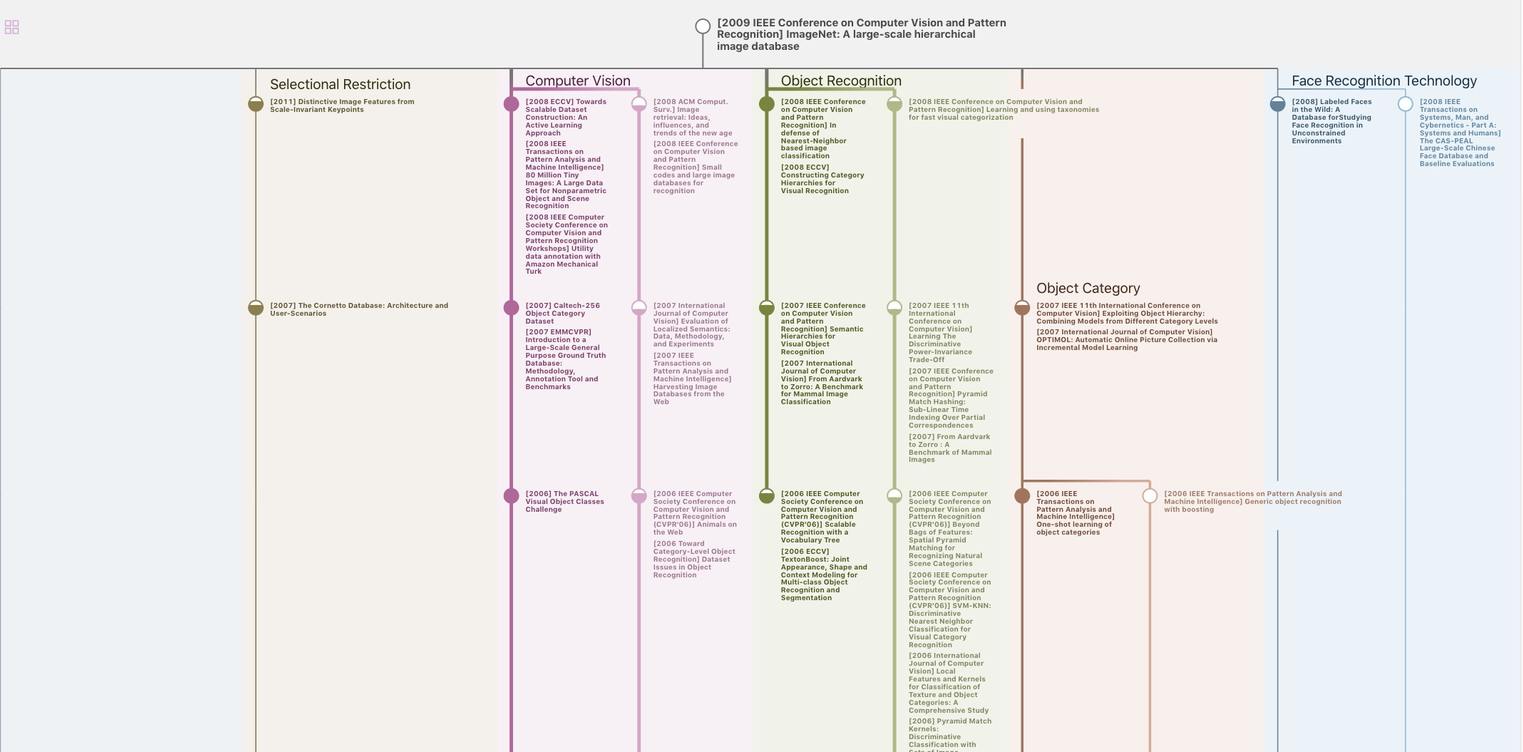
生成溯源树,研究论文发展脉络
Chat Paper
正在生成论文摘要