Collision Avoidance Using Deep Learning-Based Monocular Vision
SN Comput. Sci.(2021)
摘要
Autonomous driving technologies, including monocular vision-based approaches, are in the forefront of industrial and research communities, since they are expected to have a significant impact on economy and society. However, they have limitations in terms of crash avoidance because of the rarity of labeled data for collisions in everyday traffic, as well as due to the complexity of driving situations. In this work, we propose a simple method based solely on monocular vision to overcome the data scarcity problem and to promote forward collision avoidance systems. We exploit state-of-the-art deep learning-based optical flow and monocular depth estimation methods, as well as object detection to estimate the speed of the ego-vehicle and to identify the lead vehicle, respectively. The proposed method utilizes car stop situations as collision surrogates to obtain data for time to collision estimation. We evaluate this approach on our own driving videos, collected using a spherical camera and smart glasses. Our results indicate that similar accuracy can be achieved on both video sources: the external road view from the car’s, and the ego-centric view from the driver’s perspective. Additionally, we set forth the possibility of using spherical cameras as opposed to traditional cameras for vision-based automotive sensing.
更多查看译文
关键词
Monocular vision,Time to collision,Deep learning,Spherical camera,Ego-centric video
AI 理解论文
溯源树
样例
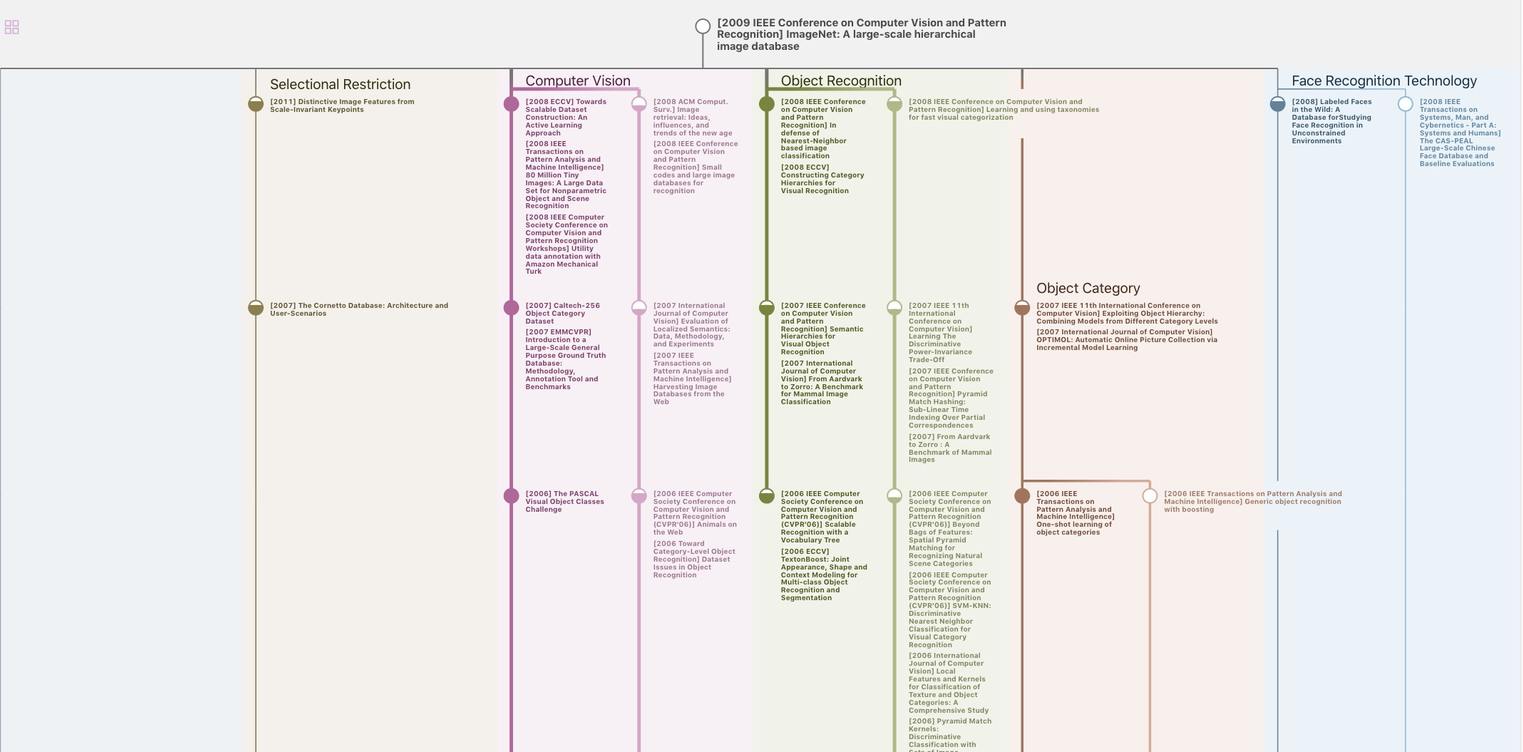
生成溯源树,研究论文发展脉络
Chat Paper
正在生成论文摘要