Case Study on Gap Selection for Automated Vehicles Based on Deep Q-Learning
2021 International Conference on Artificial Intelligence and Computer Science Technology (ICAICST)(2021)
摘要
Autonomous driving agents are often faced with decisions which human drivers seem to solve by their intuition. Many of these decisions can be quite complex without a straightforward way to derive e.g. a rule-based model and make the intuition available for artificial agents. This paper discusses a setup for learning decisions by applying reinforcement learning. It further exemplifies this with a purposefully not overly complex use case, the merging onto a road before the end of the ego vehicle's lane. To showcase the approach a simulation scenario is created which is used as a training and evaluation environment for the autonomous driving agent. The result shows that the agent can keep up with an exemplary implemented rule-based heuristic. The performance is analyzed and evaluated on the basis of specific instances of the traffic merger. Potential for improvement for the specific experiment is addressed along with an outlook on further research.
更多查看译文
关键词
gap selection,automated vehicles,deep q-learning,autonomous driving agent,human drivers,rule-based model,artificial agents,learning decisions,reinforcement learning,purposefully not overly complex use case,ego vehicle,evaluation environment,exemplary implemented rule-based
AI 理解论文
溯源树
样例
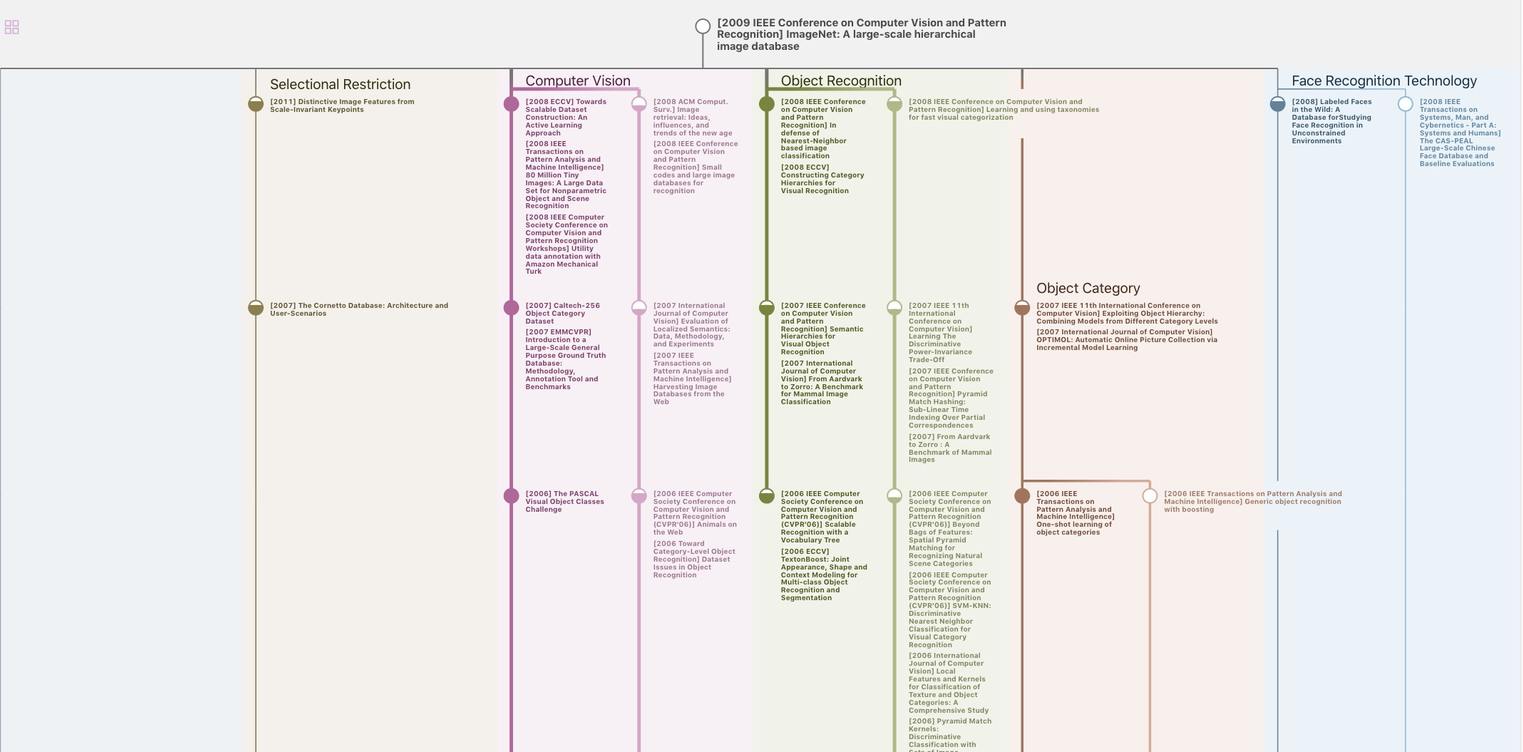
生成溯源树,研究论文发展脉络
Chat Paper
正在生成论文摘要