ALiBERT: improved automated list inspection (ALI) with BERT
DOCENG(2021)
摘要
ABSTRACTWe consider Automated List Inspection (ALI), a content-based text recommendation system that assists auditors in matching relevant text passages from notes in financial statements to specific law regulations. ALI follows a ranking paradigm in which a fixed number of requirements per textual passage are shown to the user. Despite achieving impressive ranking performance, the user experience can still be improved by showing a dynamic number of recommendations. Besides, existing models rely on a feature-based language model that needs to be pre-trained on a large corpus of domain-specific datasets. Moreover, they cannot be trained in an end-to-end fashion by jointly optimizing with language model parameters. In this work, we alleviate these concerns by considering a multi-label classification approach that predicts dynamic requirement sequences. We base our model on pre-trained BERT that allows us to fine-tune the whole model in an end-to-end fashion, thereby avoiding the need for training a language representation model. We conclude by presenting a detailed evaluation of the proposed model on two German financial datasets.
更多查看译文
关键词
Neural Networks, Text Classification, Natural Language Processing
AI 理解论文
溯源树
样例
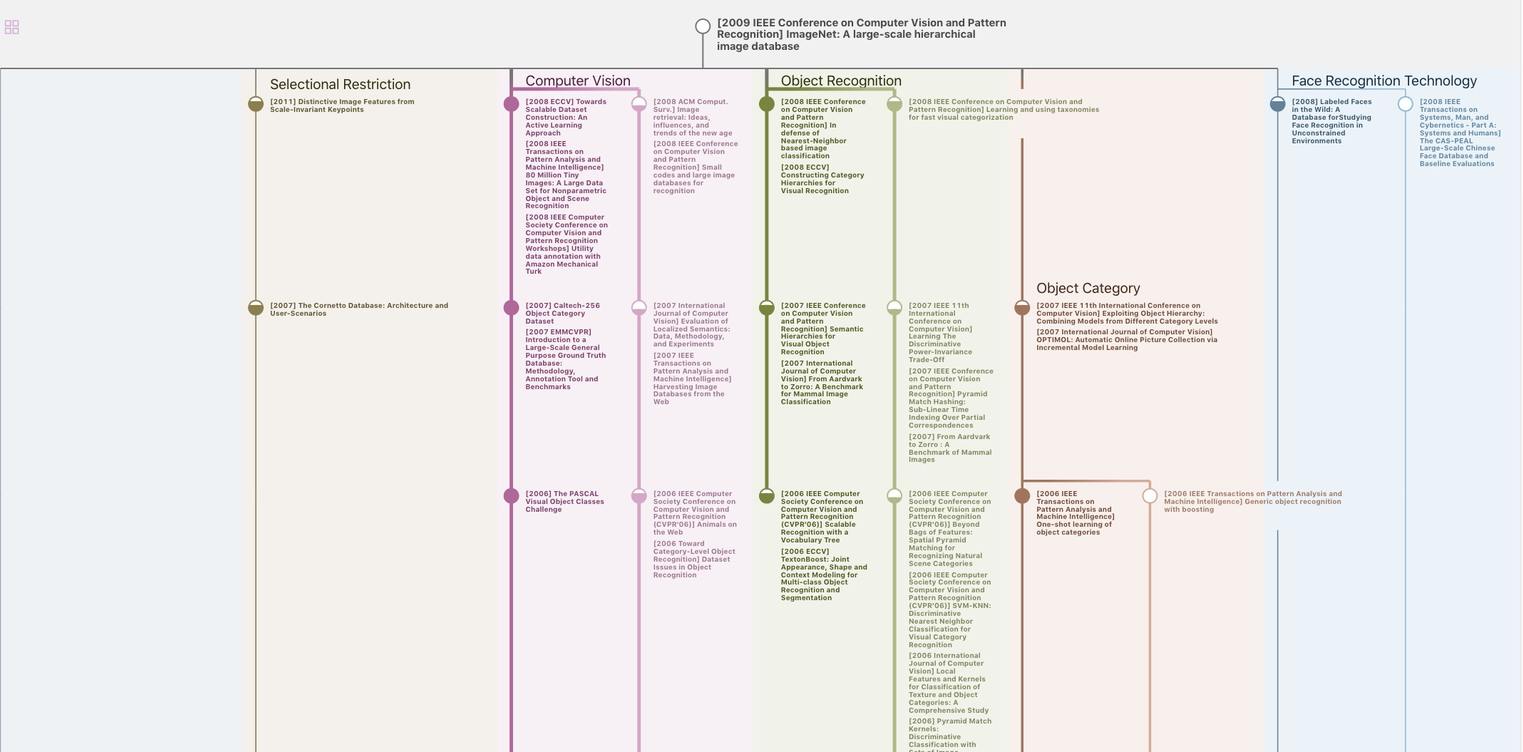
生成溯源树,研究论文发展脉络
Chat Paper
正在生成论文摘要