Cold-Start Hospital Length of Stay Prediction Using Positive-Unlabeled Learning
2021 IEEE EMBS International Conference on Biomedical and Health Informatics (BHI)(2021)
摘要
Accurately predicting the in-hospital length of stay (LOS) at the time of admission can positively impact healthcare metrics. Machine learning (ML) techniques have been used to predict hospital patients’ LOS based on their demographic and clinical characteristics. During the regular steady-state operation, traditional supervised-learning classification algorithms can be used for this purpose to inform hospital planning. However, when there are sudden changes to the admission and patient statistics, such as during the onset of a pandemic or the establishment of a new hospital, these approaches break down because reliable data for training ML models becomes available only gradually over time. This paper shows how LOS predictions can be improved during such a cold-start transition period by utilizing the positive-unlabelled (PU) learning techniques. Experimental results from using two PU learning algorithms with inpatient data from New York state hospitals support the proposed approach.
更多查看译文
关键词
healthcare,length of stay,machine learning,classification,semi-supervised learning,positive-unlabeled learning
AI 理解论文
溯源树
样例
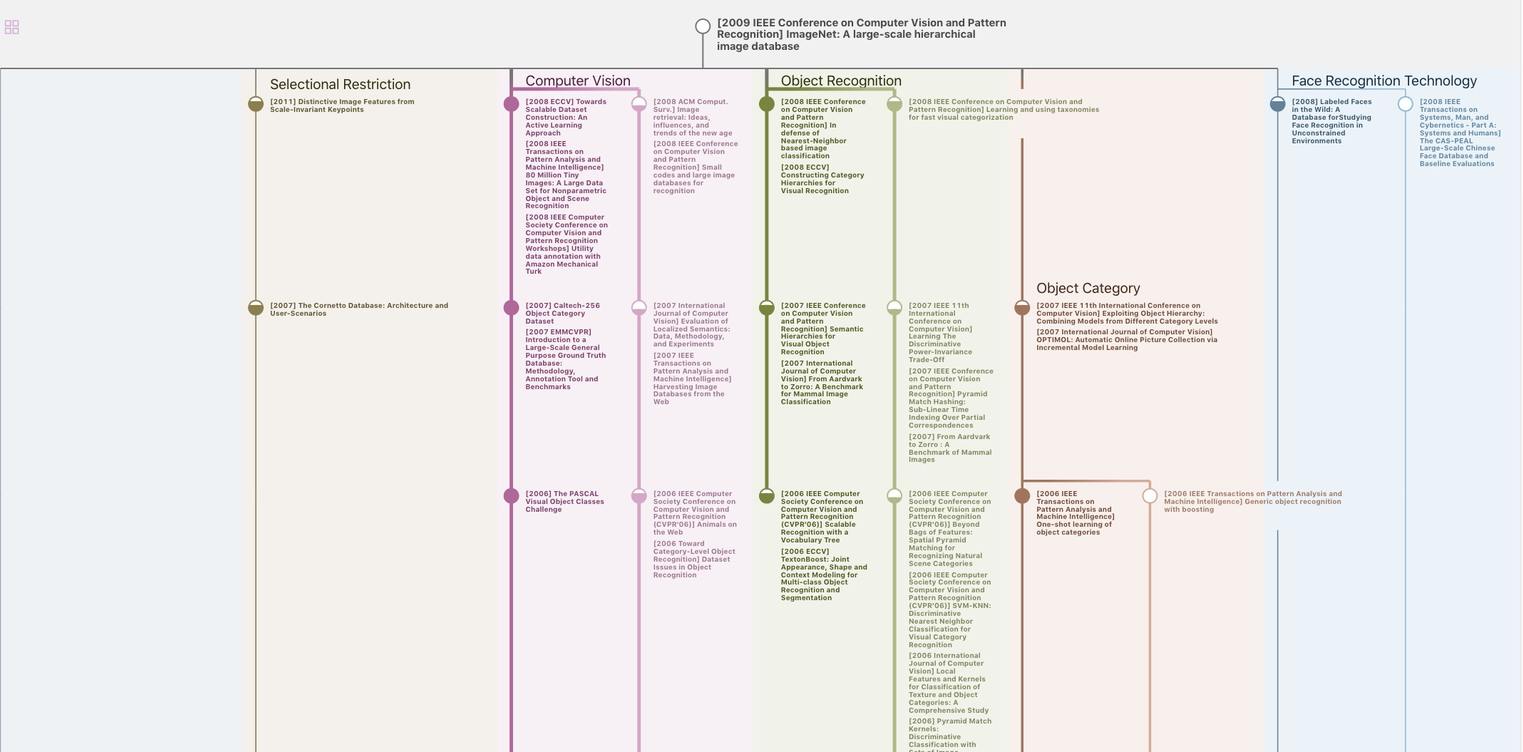
生成溯源树,研究论文发展脉络
Chat Paper
正在生成论文摘要