Decentralized Knowledge Transfer on Edge Networks for Detecting Cancer in Images
2021 IEEE EMBS International Conference on Biomedical and Health Informatics (BHI)(2021)
摘要
The proliferation of edge networks creates an opportunity to utilize extremely low latency servers for offloading computation. In the healthcare domain, these will allow for more significant opportunities for personalized medicine and health monitoring solutions that will be directly connected to a health-care provider where patient data will be analyzed. Optimally, a model fitted to local data could be shared between providers to benefit from data that is not available locally, whether to debias a model or to gain better insight on a rarely seen condition. Since patient data privacy is of utmost importance, we require a method for transferring knowledge from sources containing data of interest without exposing or sharing local data. We propose such a method based on knowledge distillation that can transfer knowledge between models in real-time. Our approach results in lower training and converging time in addition to the ability to classify targets that are rarely or never seen locally accurately. Our method emphasizes transferring knowledge without sacrificing the insights gained from local data. We show our method works on skin cancer classification, detecting tumors in brain MRI scans, and other image datasets. All assuming data is non-i.i.d.
更多查看译文
关键词
Machine learning,Knowledge transfer,Edge computing
AI 理解论文
溯源树
样例
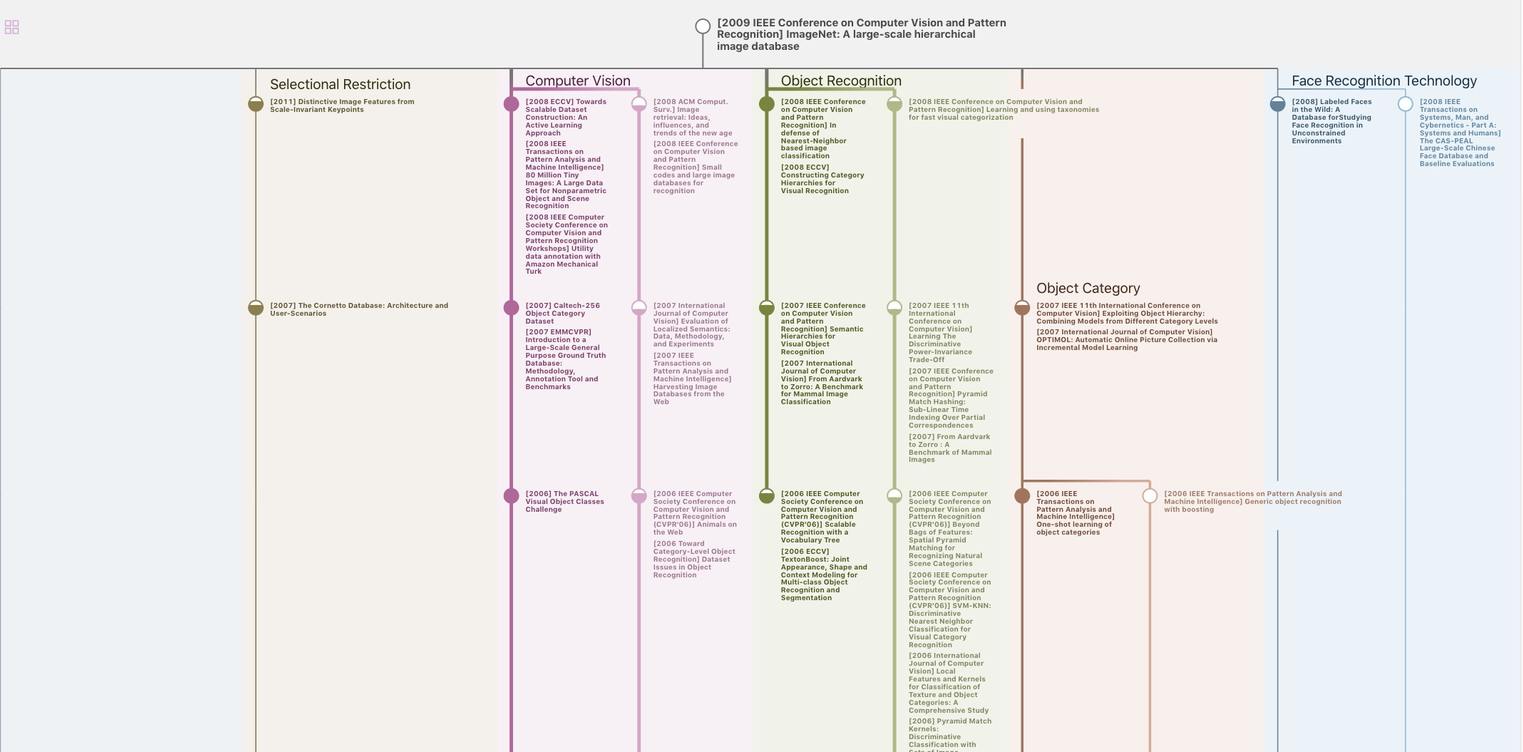
生成溯源树,研究论文发展脉络
Chat Paper
正在生成论文摘要