Amazon SageMaker Automatic Model Tuning: Scalable Gradient-Free Optimization
Knowledge Discovery and Data Mining(2021)
摘要
ABSTRACTTuning complex machine learning systems is challenging. Machine learning typically requires to set hyperparameters, be it regularization, architecture, or optimization parameters, whose tuning is critical to achieve good predictive performance. To democratize access to machine learning systems, it is essential to automate the tuning. This paper presents Amazon SageMaker Automatic Model Tuning (AMT), a fully managed system for gradient-free optimization at scale. AMT finds the best version of a trained machine learning model by repeatedly evaluating it with different hyperparameter configurations. It leverages either random search or Bayesian optimization to choose the hyperparameter values resulting in the best model, as measured by the metric chosen by the user. AMT can be used with built-in algorithms, custom algorithms, and Amazon SageMaker pre-built containers for machine learning frameworks. We discuss the core functionality, system architecture, our design principles, and lessons learned. We also describe more advanced features of AMT, such as automated early stopping and warm-starting, showing in experiments their benefits to users.
更多查看译文
关键词
AutoML, hyperparameter tuning, scalable systems
AI 理解论文
溯源树
样例
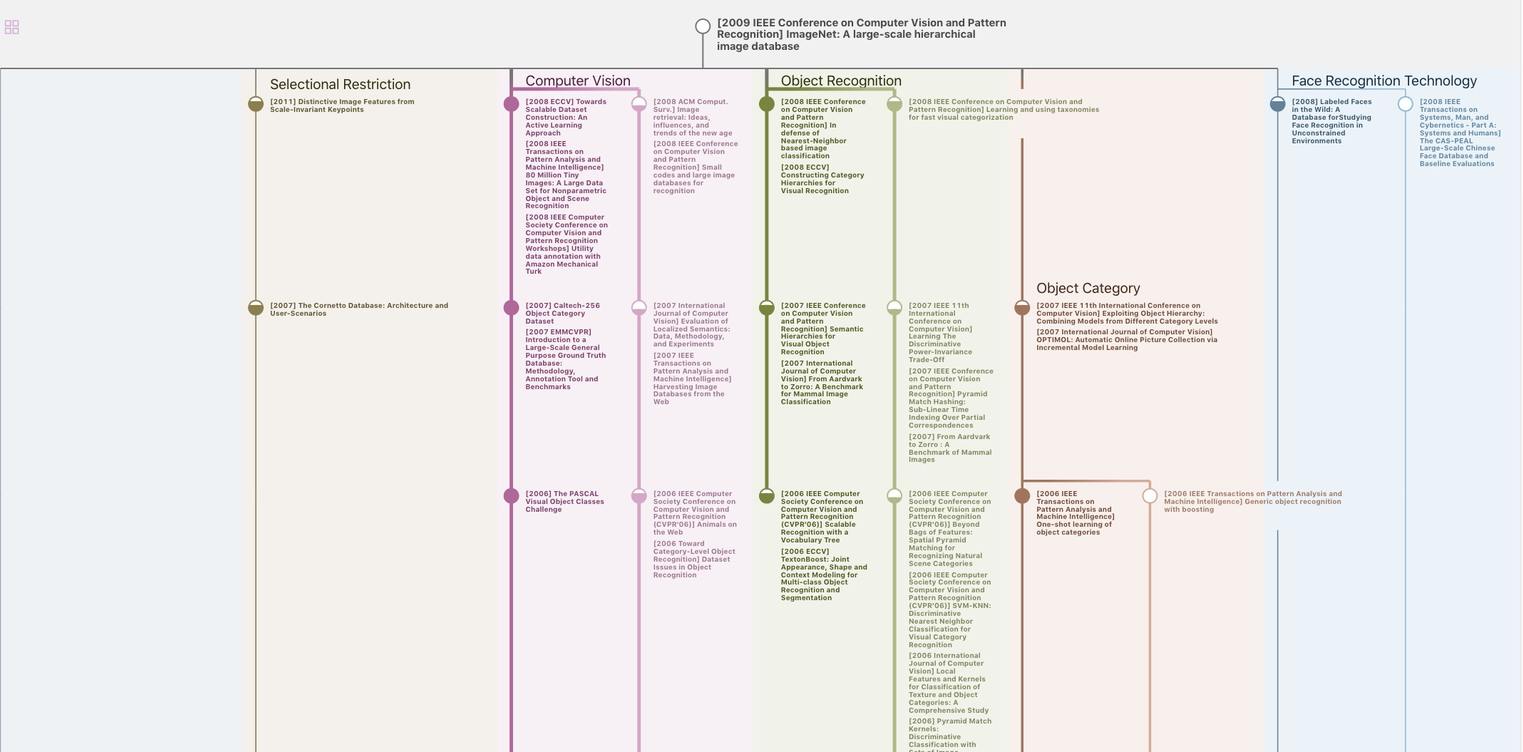
生成溯源树,研究论文发展脉络
Chat Paper
正在生成论文摘要