Meta Self-training for Few-shot Neural Sequence Labeling
Knowledge Discovery and Data Mining(2021)
摘要
ABSTRACTNeural sequence labeling is widely adopted for many Natural Language Processing (NLP) tasks, such as Named Entity Recognition (NER) and slot tagging for dialog systems and semantic parsing. Recent advances with large-scale pre-trained language models have shown remarkable success in these tasks when fine-tuned on large amounts of task-specific labeled data. However, obtaining such large-scale labeled training data is not only costly, but also may not be feasible in many sensitive user applications due to data access and privacy constraints. This is exacerbated for sequence labeling tasks requiring such annotations at token-level. In this work, we develop techniques to address the label scarcity challenge for neural sequence labeling models. Specifically, we propose a meta self-training framework which leverages very few manually annotated labels for training neural sequence models. While self-training serves as an effective mechanism to learn from large amounts of unlabeled data via iterative knowledge exchange -- meta-learning helps in adaptive sample re-weighting to mitigate error propagation from noisy pseudo-labels. Extensive experiments on six benchmark datasets including two for massive multilingual NER and four slot tagging datasets for task-oriented dialog systems demonstrate the effectiveness of our method. With only 10 labeled examples for each class in each task, the proposed method achieves 10% improvement over state-of-the-art methods demonstrating its effectiveness for limited training labels regime.
更多查看译文
关键词
meta-learning, self-training, natural language processing
AI 理解论文
溯源树
样例
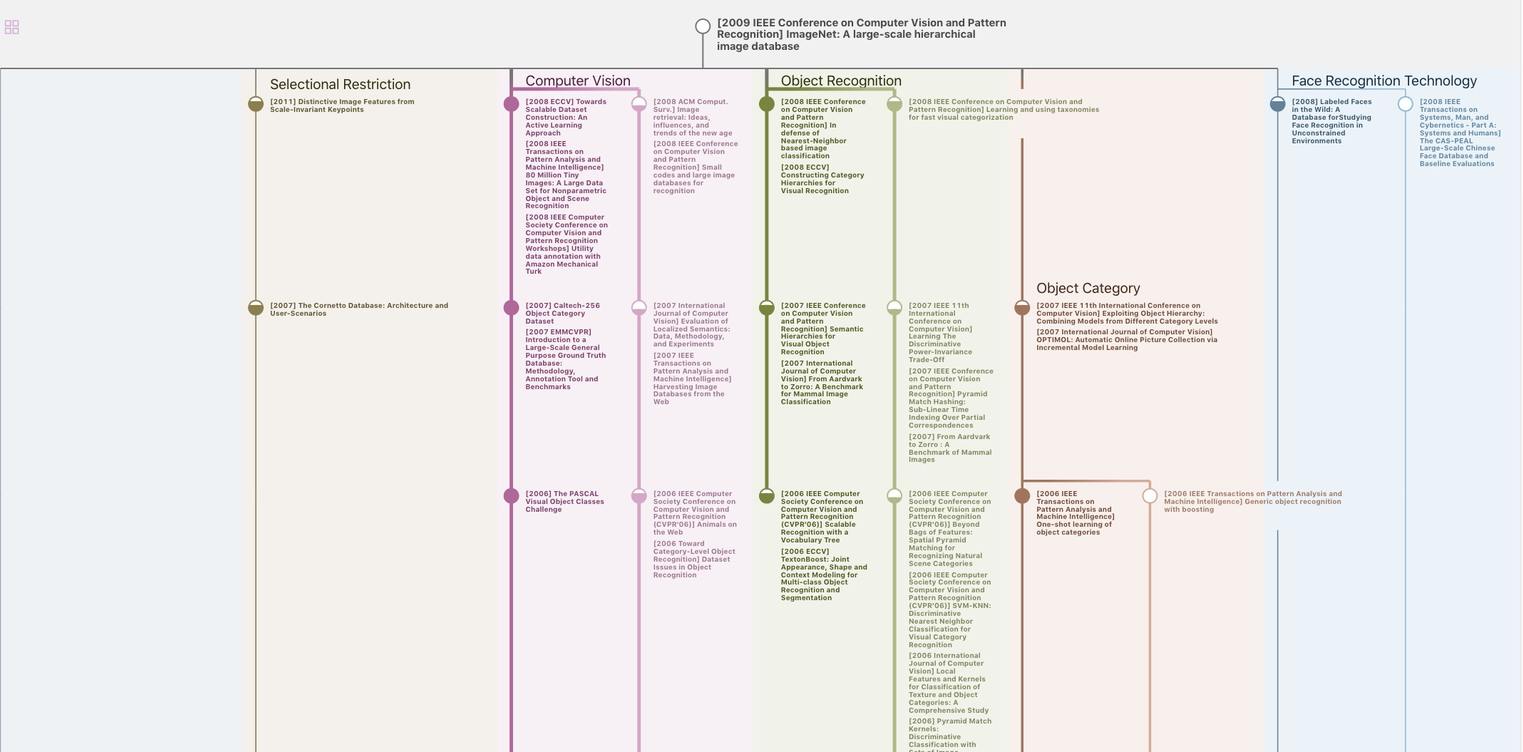
生成溯源树,研究论文发展脉络
Chat Paper
正在生成论文摘要