A Unified Representation Learning Strategy for Open Relation Extraction with Ranked List Loss
springer
Abstract
Open Relation Extraction (OpenRE), aiming to extract relational facts from open-domain corpora, is a sub-task of Relation Extraction and a crucial upstream process for many other NLP tasks. However, various previous clustering-based OpenRE strategies either confine themselves to unsupervised paradigms or can not directly build a unified relational semantic space, hence impacting down-stream clustering. In this paper, we propose a novel supervised learning framework named MORE-RLL (Metric learning-based Open Relation Extraction with Ranked List Loss) to construct a semantic metric space by utilizing Ranked List Loss to discover new relational facts. Experiments on real-world datasets show that MORE-RLL can achieve excellent performance compared with previous state-of-the-art methods, demonstrating the capability of MORE-RLL in unified semantic representation learning and novel relational fact detection.
MoreTranslated text
Key words
Open-domain,Relation extraction,Deep metric learning
AI Read Science
Must-Reading Tree
Example
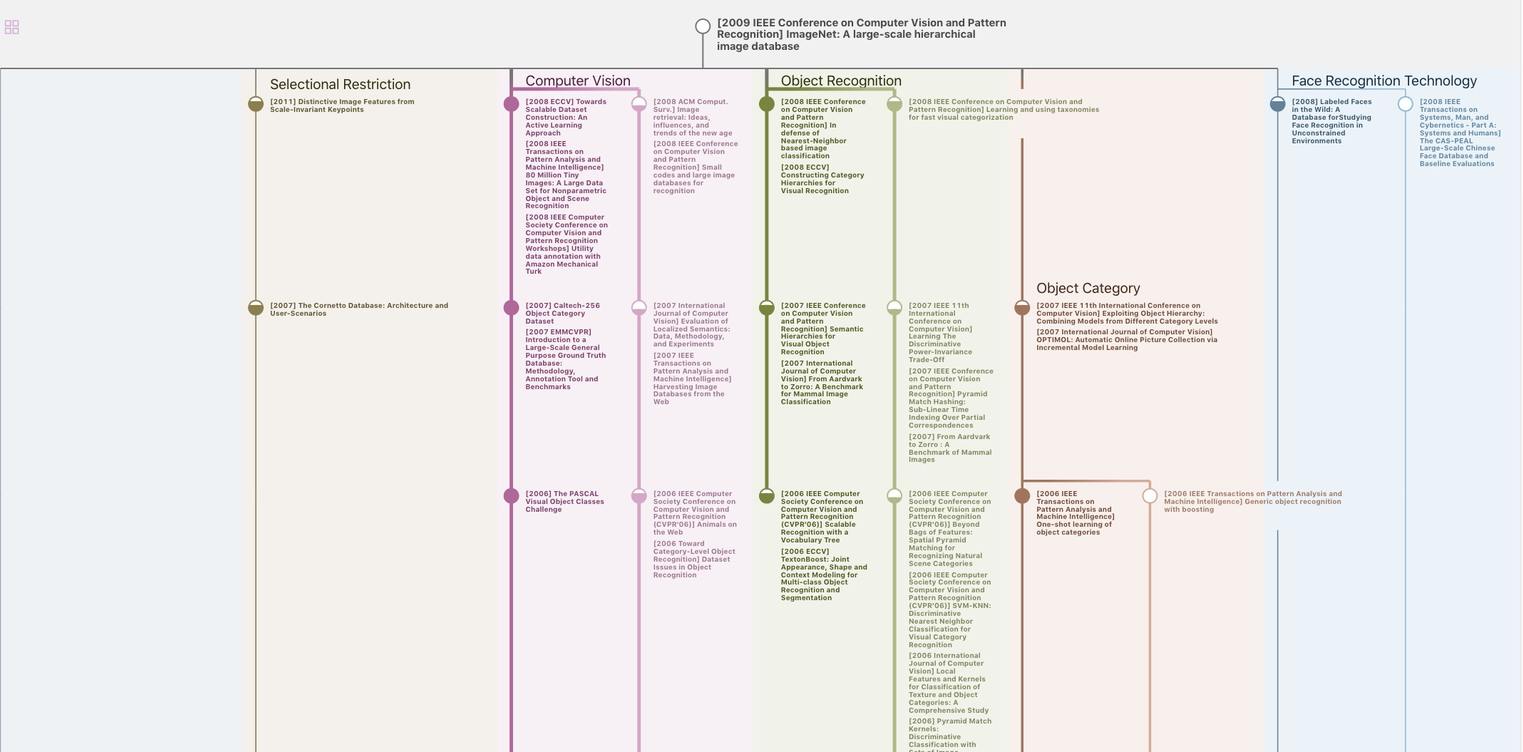
Generate MRT to find the research sequence of this paper
Chat Paper
Summary is being generated by the instructions you defined