Federated Learning for Energy-balanced Client Selection in Mobile Edge Computing
IWCMC 2021: 2021 17TH INTERNATIONAL WIRELESS COMMUNICATIONS & MOBILE COMPUTING CONFERENCE (IWCMC)(2021)
摘要
Mobile edge computing (MEC) has been considered as a promising technology to provide seamless integration of multiple application services. Federated learning (FL) is carried out at edge clients in MEC for privacy-preserving training of data processing models. Despite that the edge clients with small data payloads consume less energy on FL training, the small data payload gives rise to a low learning accuracy due to insufficient input to the FL training. Inadequate selection of the edge clients can result in a large energy consumption at the edge clients, or a low learning accuracy of the FL training. In this paper, a new FL-based client selection optimization is proposed to balance the trade-off between energy consumption of the edge clients and the learning accuracy of FL. We first show that this optimization problem is NP-complete. Next, we propose a FL-based energy-accuracy balancing heuristic algorithm to approximate the optimal client selection in polynomial time. The numerical results show the advantage of our proposed algorithm.
更多查看译文
关键词
client selection, mobile edge computing, federated learning, heuristic algorithm
AI 理解论文
溯源树
样例
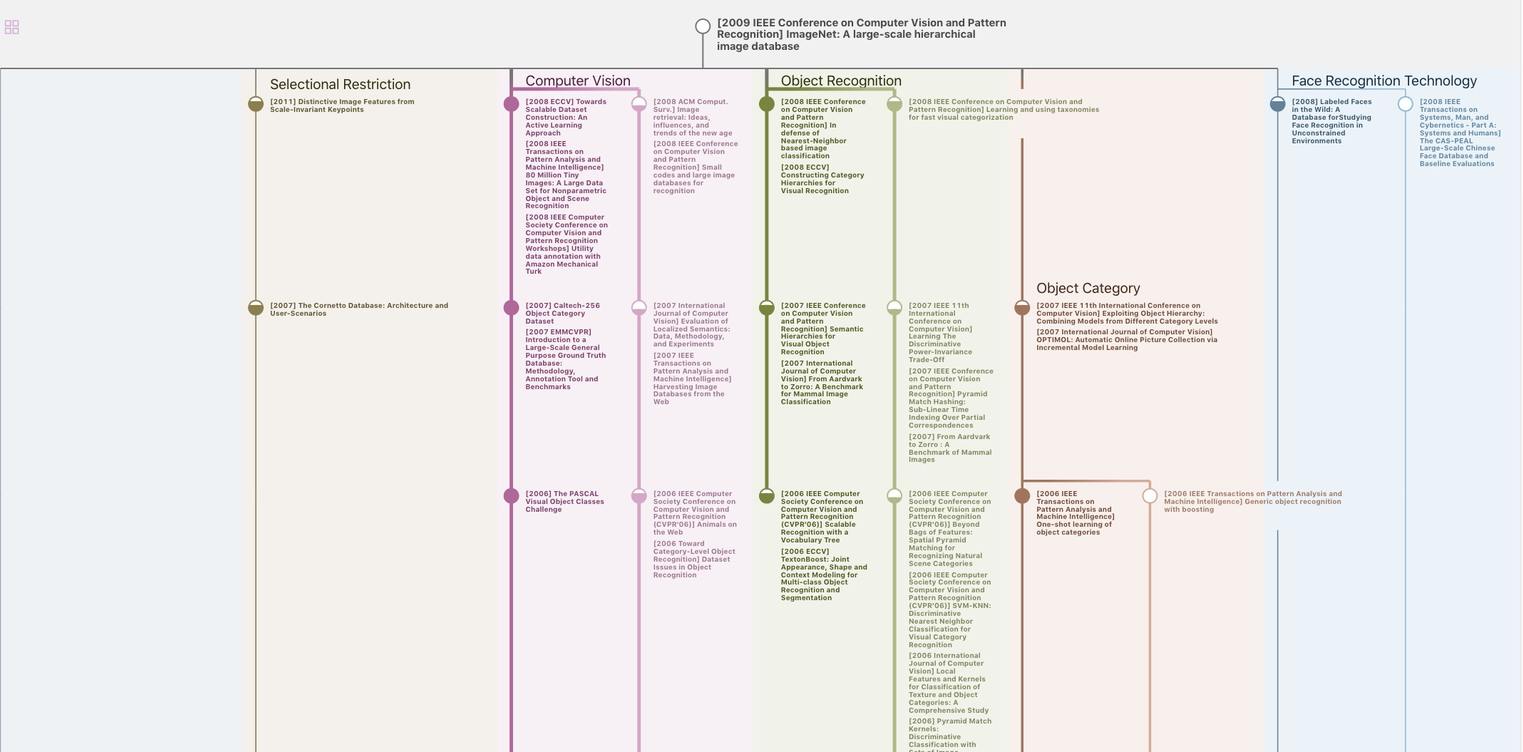
生成溯源树,研究论文发展脉络
Chat Paper
正在生成论文摘要