MM-ViT: Multi-Modal Video Transformer for Compressed Video Action Recognition
2022 IEEE/CVF Winter Conference on Applications of Computer Vision (WACV)(2022)
摘要
This paper presents a pure transformer-based approach, dubbed the Multi-Modal Video Transformer (MM-ViT), for video action recognition. Different from other schemes which solely utilize the decoded RGB frames, MM-ViT operates exclusively in the compressed video domain and exploits all readily available modalities, i.e., I-frames, motion vectors, residuals and audio waveform. In order to handle the large number of spatiotemporal tokens extracted from multiple modalities, we develop several scalable model variants which factorize self-attention across the space, time and modality dimensions. In addition, to further explore the rich inter-modal interactions and their effects, we develop and compare three distinct cross-modal attention mechanisms that can be seamlessly integrated into the transformer building block. Extensive experiments on three public action recognition benchmarks (UCF-101, Something-Something-v2, Kinetics-600) demonstrate that MM-ViT outperforms the state-of-the-art video transformers in both efficiency and accuracy, and performs better or equally well to the state-of-the-art CNN counterparts with computationally-heavy optical flow.
更多查看译文
关键词
Action and Behavior Recognition Visual Reasoning, Analysis and Understanding
AI 理解论文
溯源树
样例
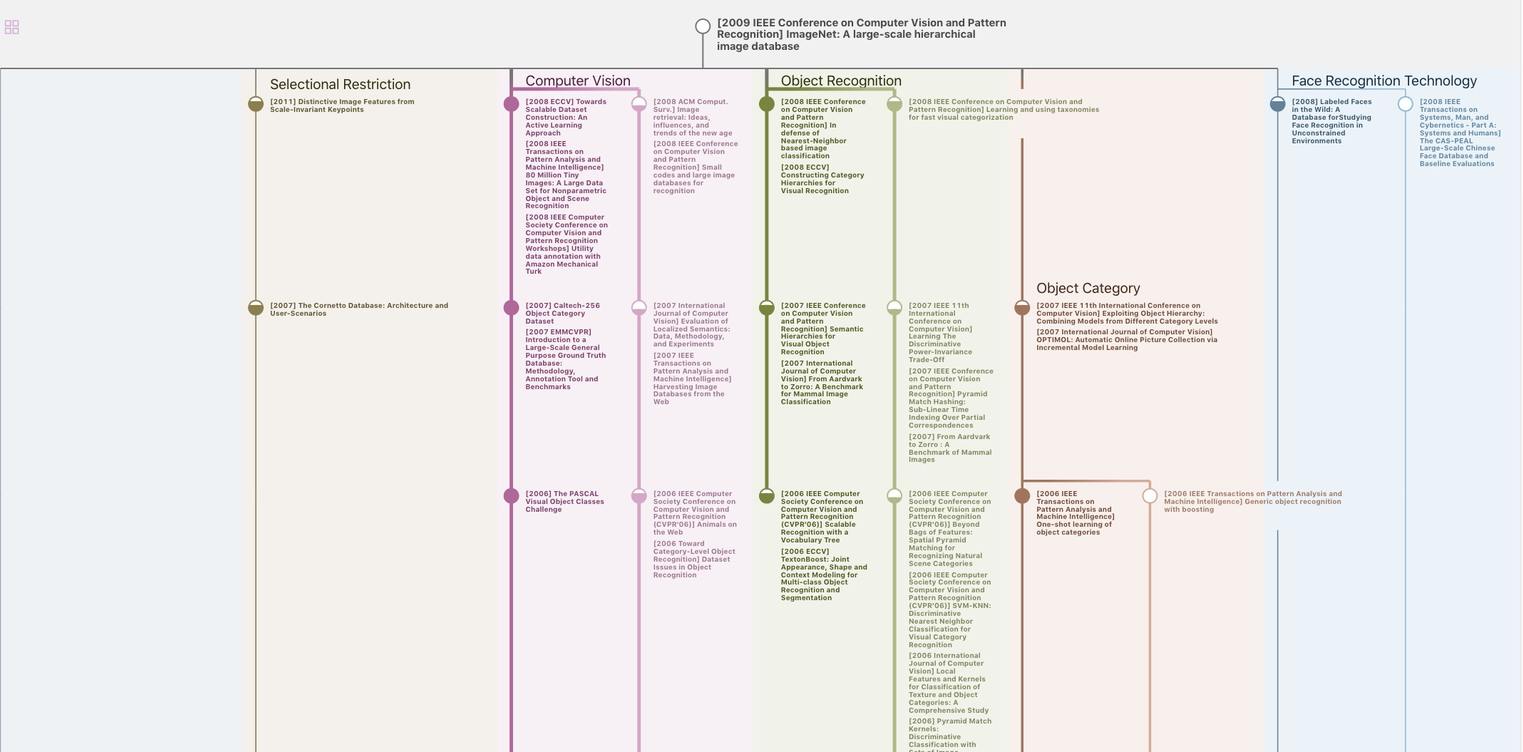
生成溯源树,研究论文发展脉络
Chat Paper
正在生成论文摘要