Unsupervised Local Discrimination for Medical Images
IEEE TRANSACTIONS ON PATTERN ANALYSIS AND MACHINE INTELLIGENCE(2023)
摘要
Contrastive learning, which aims to capture general representation from unlabeled images to initialize the medical analysis models, has been proven effective in alleviating the high demand for expensive annotations. Current methods mainly focus on instance-wise comparisons to learn the global discriminative features, however, pretermitting the local details to distinguish tiny anatomical structures, lesions, and tissues. To address this challenge, in this paper, we propose a general unsupervised representation learning framework, named local discrimination (LD), to learn local discriminative features for medical images by closely embedding semantically similar pixels and identifying regions of similar structures across different images. Specifically, this model is equipped with an embedding module for pixel-wise embedding and a clustering module for generating segmentation. And these two modules are unified by optimizing our novel region discrimination loss function in a mutually beneficial mechanism, which enables our model to reflect structure information as well as measure pixel-wise and region-wise similarity. Furthermore, based on LD, we propose a center-sensitive one-shot landmark localization algorithm and a shape-guided cross-modality segmentation model to foster the generalizability of our model. When transferred to downstream tasks, the learned representation by our method shows a better generalization, outperforming representation from 18 state-of-the-art (SOTA) methods and winning 9 out of all 12 downstream tasks. Especially for the challenging lesion segmentation tasks, the proposed method achieves significantly better performance.
更多查看译文
关键词
Contrastive learning,local discrimination,one-shot landmark localization,shape-guided segmentation
AI 理解论文
溯源树
样例
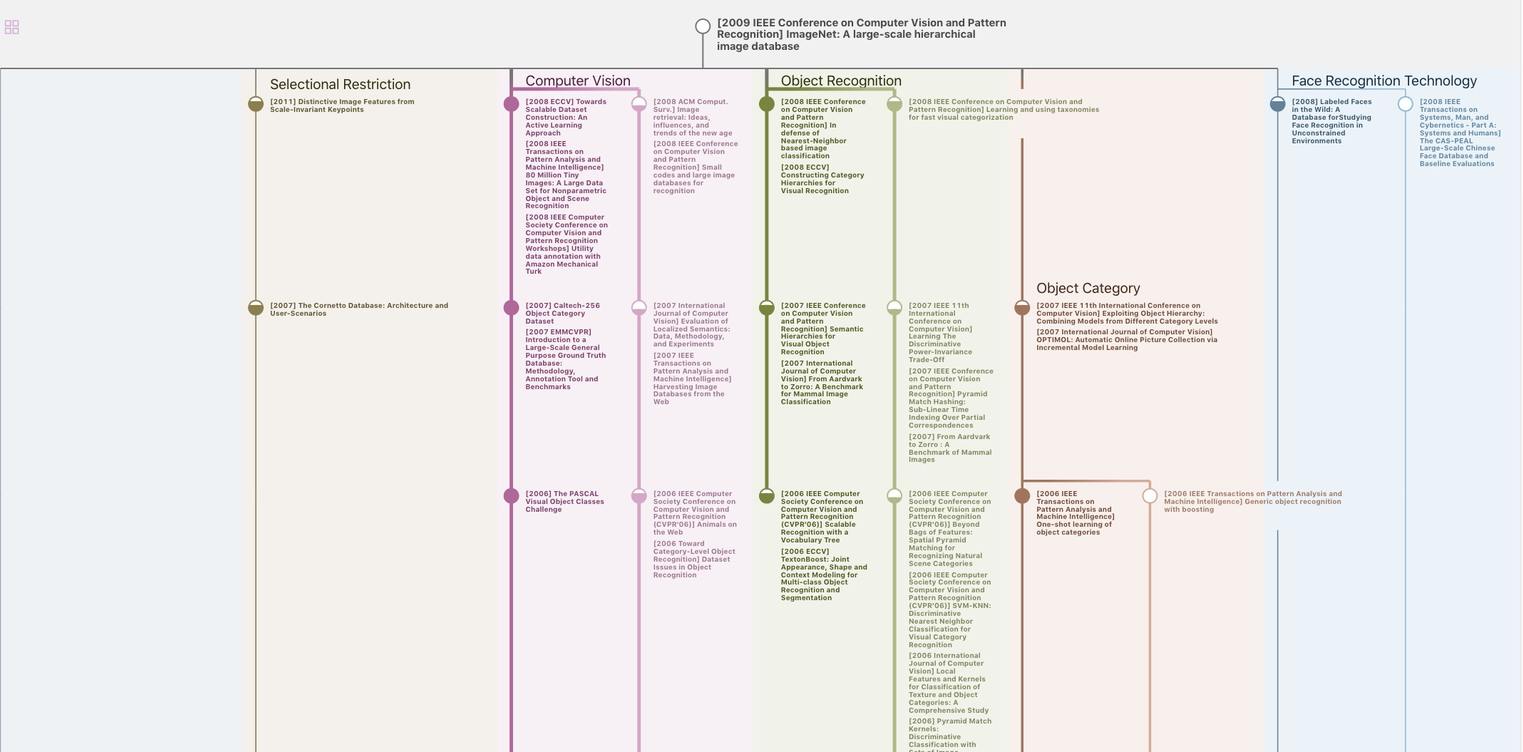
生成溯源树,研究论文发展脉络
Chat Paper
正在生成论文摘要