An Annotated Graph Model with Differential Degree Heterogeneity for Directed Networks
arxiv(2023)
摘要
Directed networks are conveniently represented as graphs in which ordered edges encode interactions between vertices. Despite their wide availability, there is a shortage of statistical models amenable for inference, specially when contextual information and degree heterogeneity are present. This paper presents an annotated graph model with parameters explicitly accounting for these features. To overcome the curse of dimensionality due to modelling degree heterogeneity, we introduce a sparsity assumption and propose a penalized likelihood approach with $\ell_1$-regularization for parameter estimation. We study the estimation and selection consistency of this approach under a sparse network assumption, and show that inference on the covariate parameter is straightforward, thus bypassing the need for the kind of debiasing commonly employed in $\ell_1$-penalized likelihood estimation. Simulation and data analysis corroborate our theoretical findings.
更多查看译文
关键词
annotated graph model,directed networks,differential degree heterogeneity
AI 理解论文
溯源树
样例
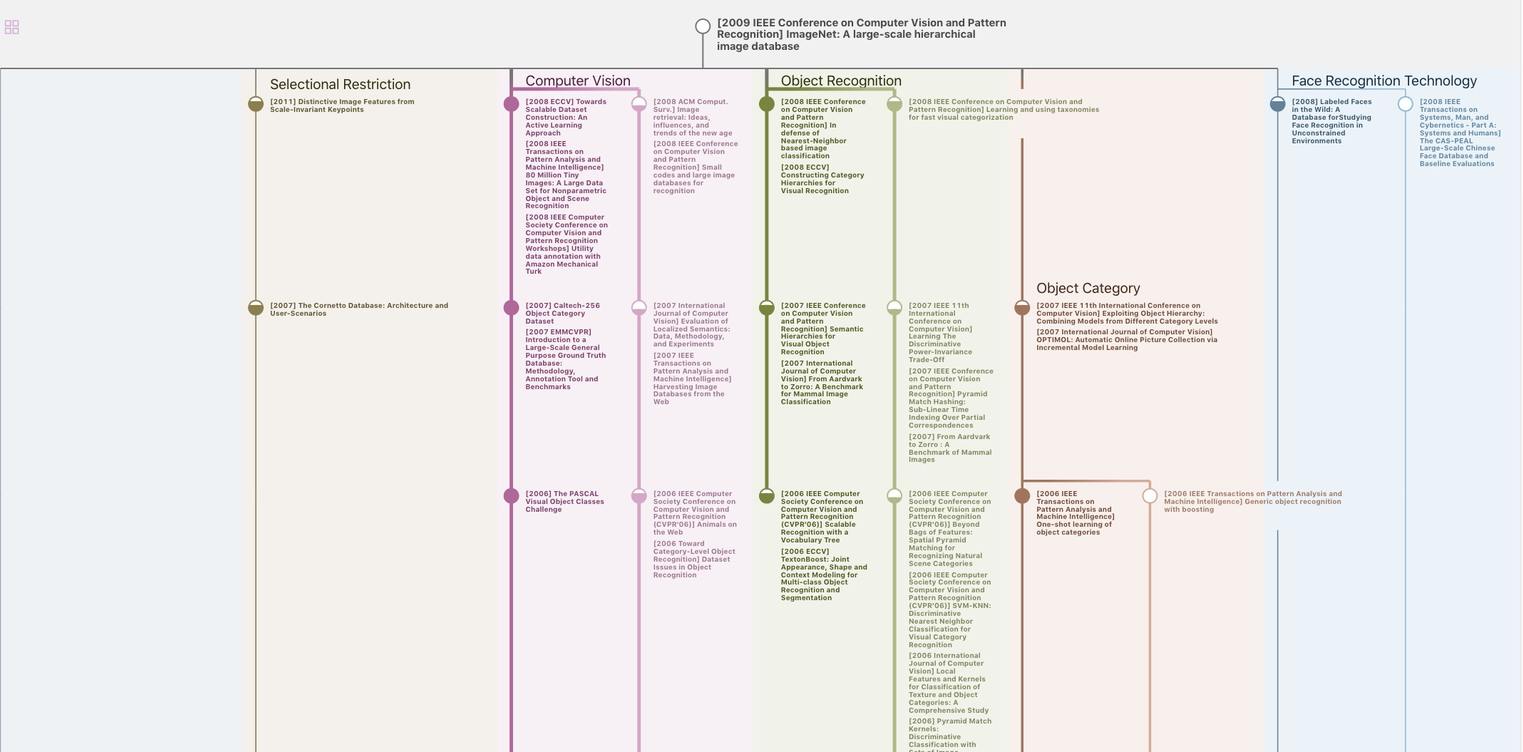
生成溯源树,研究论文发展脉络
Chat Paper
正在生成论文摘要