Rate distortion comparison of a few gradient quantizers
CoRR(2021)
摘要
This article is in the context of gradient compression. Gradient compression is a popular technique for mitigating the communication bottleneck observed when training large machine learning models in a distributed manner using gradient-based methods such as stochastic gradient descent. In this article, assuming a Gaussian distribution for the components in gradient, we find the rate distortion trade-off of gradient quantization schemes such as Scaled-sign and Top-K, and compare with the Shannon rate distortion limit. A similar comparison with vector quantizers also is presented.
更多查看译文
关键词
few gradient quantizers,rate
AI 理解论文
溯源树
样例
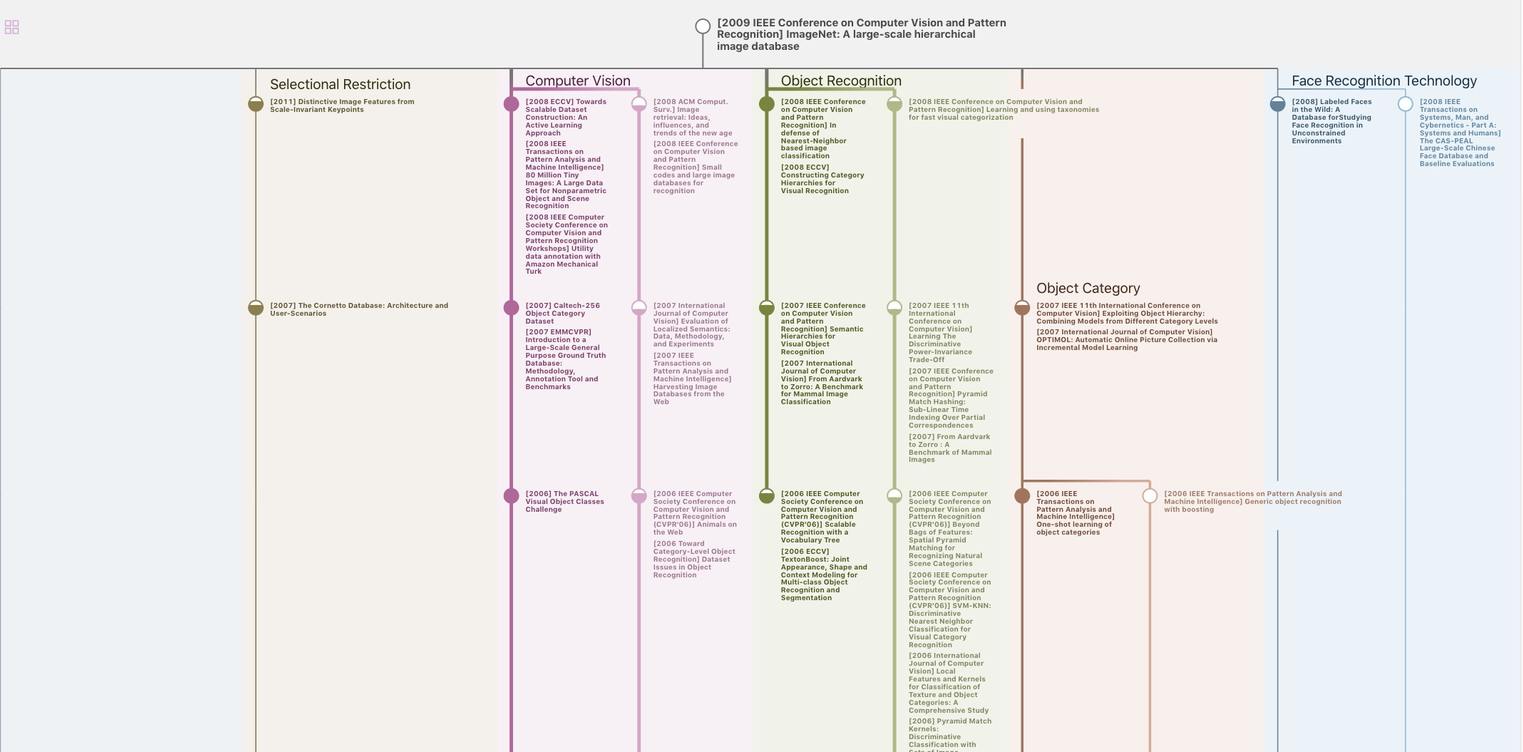
生成溯源树,研究论文发展脉络
Chat Paper
正在生成论文摘要