A Sensitivity Matrix Approach Using Two-Stage Optimization for Voltage Regulation of LV Networks with High PV Penetration
Energies(2021)SCI 4区
Abstract
The occurrence of voltage violations is a major deterrent for absorbing more rooftop solar power into smart Low-Voltage Distribution Grids (LVDGs). Recent studies have focused on decentralized control methods to solve this problem due to the high computational time in performing load flows in centralized control techniques. To address this issue, a novel sensitivity matrix was developed to estimate the voltages of the network by replacing load flow simulations. In this paper, a Centralized Active, Reactive Power Management System (CARPMS) is proposed to optimally utilize the reactive power capability of smart Photovoltaic (PV) inverters with minimal active power curtailment to mitigate the voltage violation problem. The developed sensitivity matrix is able to reduce the time consumed by 55.1% compared to load flow simulations, enabling near-real-time control optimization. Given the large solution space of power systems, a novel two-stage optimization is proposed, where the solution space is narrowed down by a Feasible Region Search (FRS) step, followed by Particle Swarm Optimization (PSO). The failure of standalone PSO to converge to a feasible solution for 34% of the scenarios evaluated further validates the necessity of the two-stage optimization using FRS. The performance of the proposed methodology was analysed in comparison to the load flow method to demonstrate the accuracy and the capability of the optimization algorithm to mitigate voltage violations in near-real time. The deviations of the mean voltages of the proposed methodology from the load flow method were: 6.5×10−3 p.u for reactive power control using Q-injection, 1.02×10−2 p.u for reactive power control using Q-absorption, and 0 p.u for active power curtailment case.
MoreTranslated text
Key words
smart grid,renewable energy integration,rooftop solar PV,PV inverter control,voltage violation
PDF
View via Publisher
AI Read Science
AI Summary
AI Summary is the key point extracted automatically understanding the full text of the paper, including the background, methods, results, conclusions, icons and other key content, so that you can get the outline of the paper at a glance.
Example
Background
Key content
Introduction
Methods
Results
Related work
Fund
Key content
- Pretraining has recently greatly promoted the development of natural language processing (NLP)
- We show that M6 outperforms the baselines in multimodal downstream tasks, and the large M6 with 10 parameters can reach a better performance
- We propose a method called M6 that is able to process information of multiple modalities and perform both single-modal and cross-modal understanding and generation
- The model is scaled to large model with 10 billion parameters with sophisticated deployment, and the 10 -parameter M6-large is the largest pretrained model in Chinese
- Experimental results show that our proposed M6 outperforms the baseline in a number of downstream tasks concerning both single modality and multiple modalities We will continue the pretraining of extremely large models by increasing data to explore the limit of its performance
Try using models to generate summary,it takes about 60s
Must-Reading Tree
Example
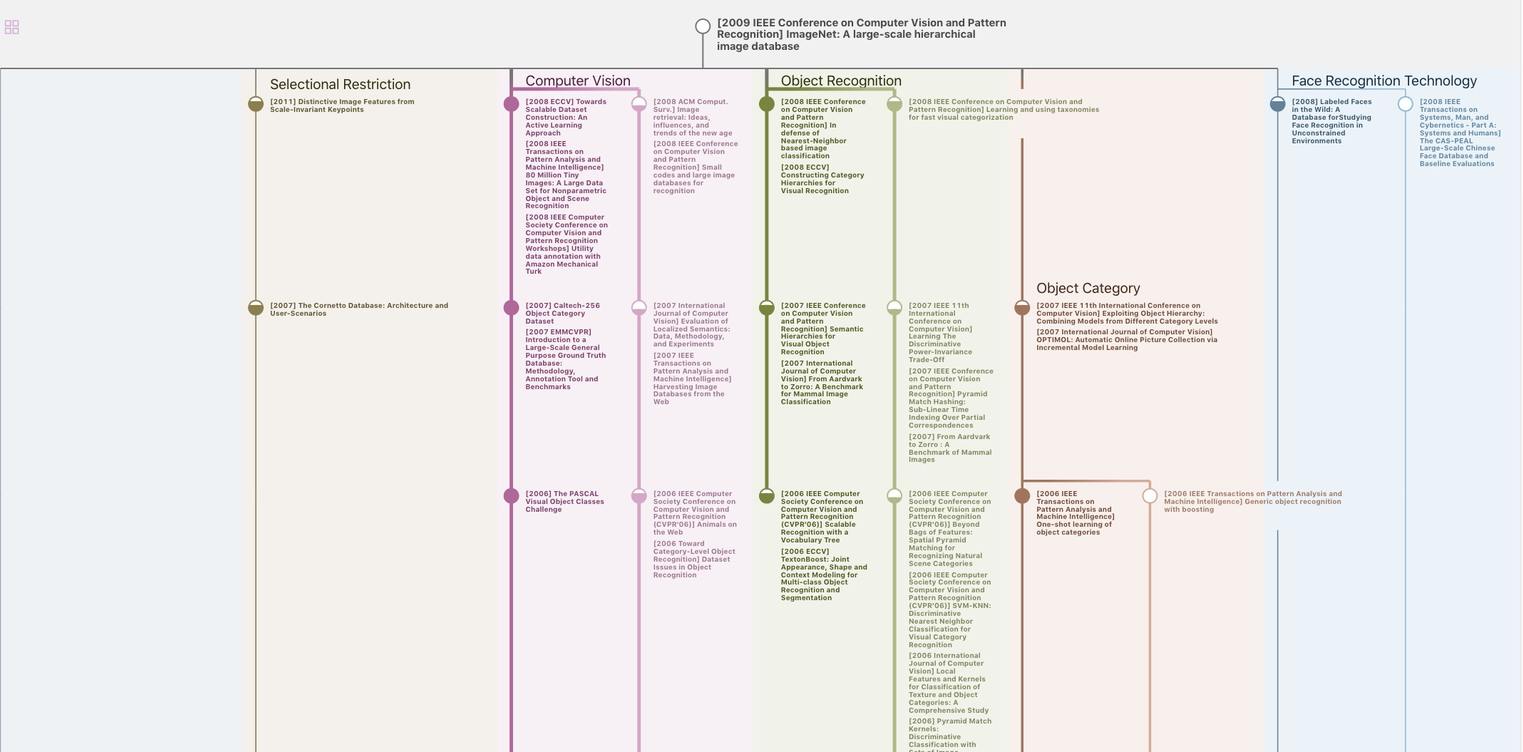
Generate MRT to find the research sequence of this paper
Related Papers
Data Disclaimer
The page data are from open Internet sources, cooperative publishers and automatic analysis results through AI technology. We do not make any commitments and guarantees for the validity, accuracy, correctness, reliability, completeness and timeliness of the page data. If you have any questions, please contact us by email: report@aminer.cn
Chat Paper
去 AI 文献库 对话