Learning Spatiotemporal Occupancy Grid Maps for Lifelong Navigation in Dynamic Scenes
2022 International Conference on Robotics and Automation (ICRA)(2022)
摘要
We present a novel method for generating, predicting, and using Spatiotemporal Occupancy Grid Maps (SOGM), which embed future information of dynamic scenes. Our au-tomated generation process creates groundtruth SOGMs from previous navigation data. We build on prior work to annotate lidar points based on their dynamic properties, which are then projected on time-stamped 2D grids: SOGMs. We design a 3D-2D feedforward architecture, trained to predict the future time steps of SOGMs, given 3D lidar frames as input. Our pipeline is entirely self-supervised, thus enabling lifelong learning for robots. The network is composed of a 3D back-end that extracts rich features and enables the semantic segmentation of the lidar frames, and a 2D front-end that predicts the future information embedded in the SOGMs within planning. We also design a navigation pipeline that uses these predicted SOGMs. We provide both quantitative and qualitative insights into the predictions and validate our choices of network design with a comparison to the state of the art and ablation studies.
更多查看译文
关键词
spatiotemporal occupancy grid maps,lifelong navigation
AI 理解论文
溯源树
样例
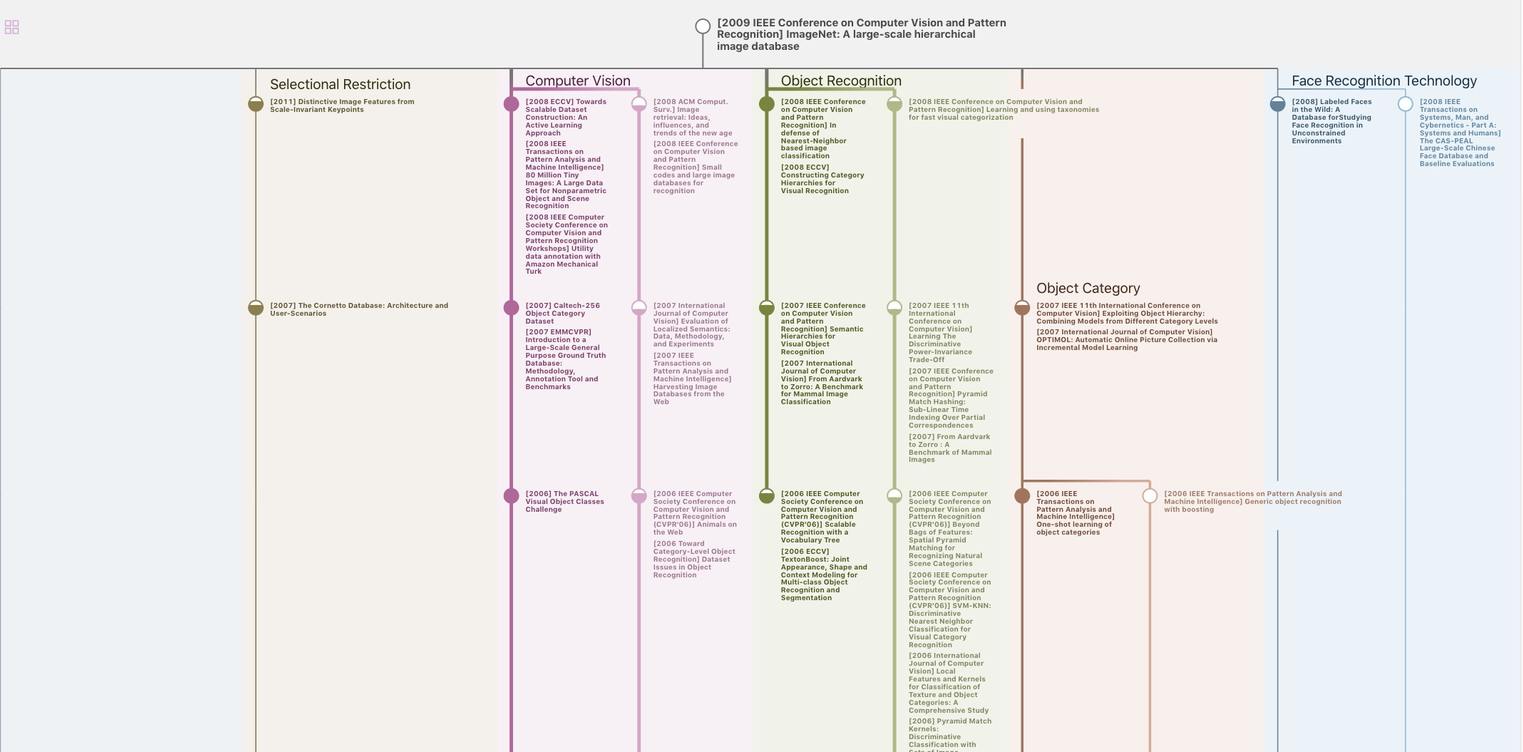
生成溯源树,研究论文发展脉络
Chat Paper
正在生成论文摘要