Incremental learning strategies for credit cards fraud detection
INTERNATIONAL JOURNAL OF DATA SCIENCE AND ANALYTICS(2021)
摘要
Every second, thousands of credit or debit card transactions are processed in financial institutions. This extensive amount of data and its sequential nature make the problem of fraud detection particularly challenging. Most analytical strategies used in production are still based on batch learning, which is inadequate for two reasons: Models quickly become outdated and require sensitive data storage. The evolving nature of bank fraud enshrines the importance of having up-to-date models, and sensitive data retention makes companies vulnerable to infringements of the European General Data Protection Regulation. For these reasons, evaluating incremental learning strategies is recommended. This paper designs and evaluates incremental learning solutions for real-world fraud detection systems. The aim is to demonstrate the competitiveness of incremental learning over conventional batch approaches and, consequently, improve its accuracy employing ensemble learning, diversity and transfer learning. An experimental analysis is conducted on a full-scale case study including five months of e-commerce transactions and made available by our industry partner, Worldline.
更多查看译文
关键词
Fraud detection, Incremental learning, Transfer Learning, Fintech
AI 理解论文
溯源树
样例
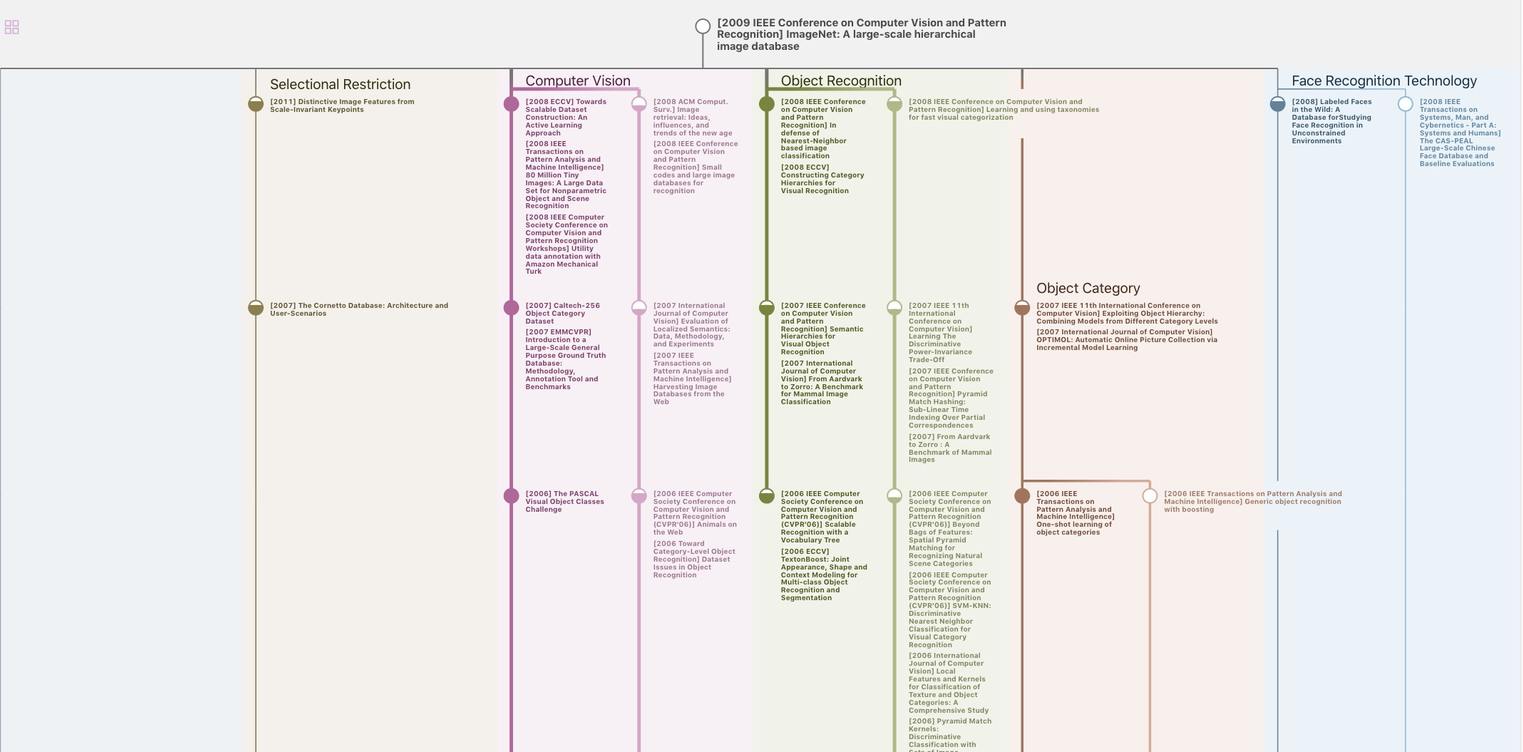
生成溯源树,研究论文发展脉络
Chat Paper
正在生成论文摘要