Field-Guide-Inspired Zero-Shot Learning.
ICCV(2021)
摘要
Modern recognition systems require large amounts of supervision to achieve accuracy. Adapting to new domains requires significant data from experts, which is onerous and can become too expensive. Zero-shot learning requires an annotated set of attributes for a novel category. Annotating the full set of attributes for a novel category proves to be a tedious and expensive task in deployment. This is especially the case when the recognition domain is an expert domain. We introduce a new field-guide-inspired approach to zero-shot annotation where the learner model interactively asks for the most useful attributes that define a class. We evaluate our method on classification benchmarks with attribute annotations like CUB, SUN, and AWA2 and show that our model achieves the performance of a model with full annotations at the cost of a significantly fewer number of annotations. Since the time of experts is precious, decreasing annotation cost can be very valuable for real-world deployment.
更多查看译文
关键词
Transfer/Low-shot/Semi/Unsupervised Learning,Recognition and classification,Vision + language
AI 理解论文
溯源树
样例
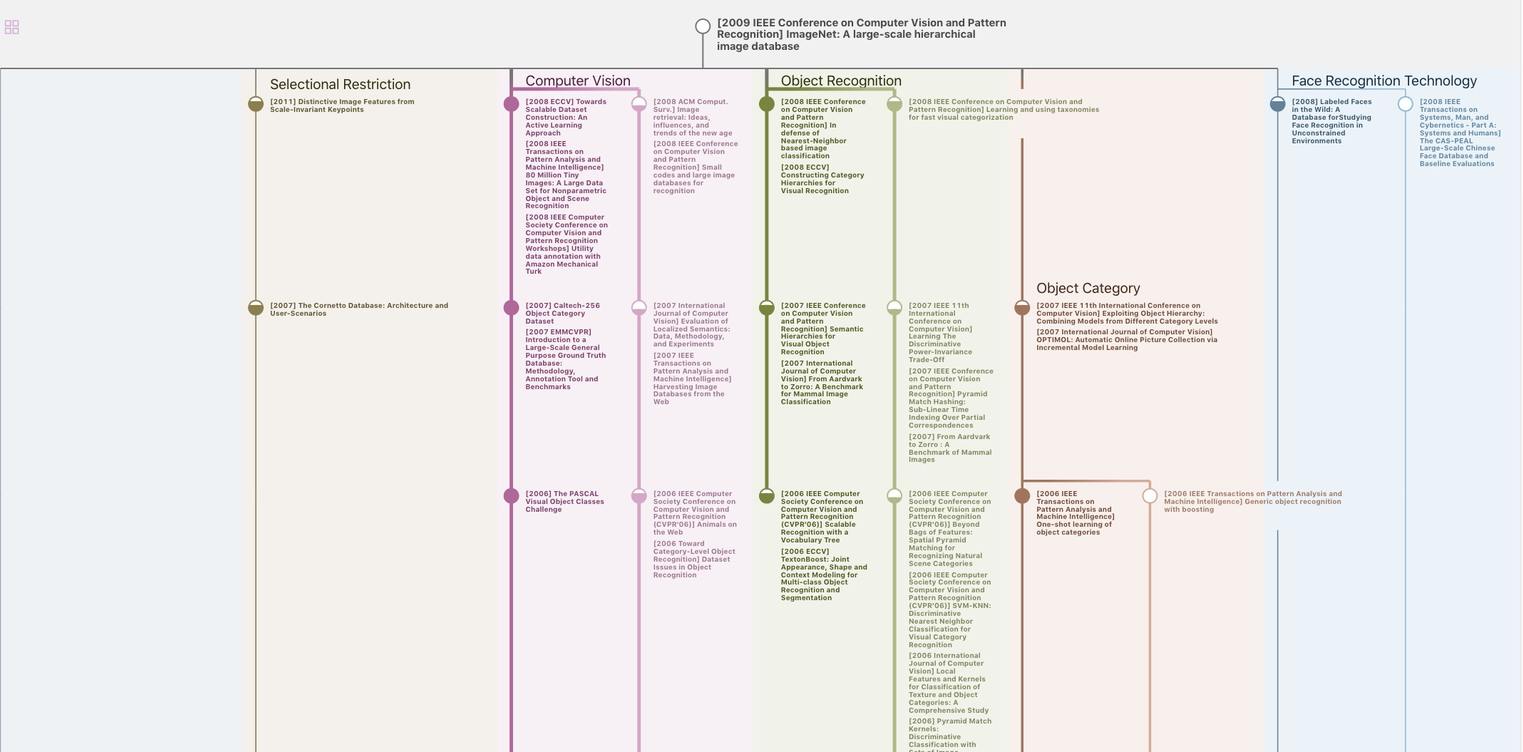
生成溯源树,研究论文发展脉络
Chat Paper
正在生成论文摘要