Memory-Augmented Non-Local Attention for Video Super-Resolution
IEEE Conference on Computer Vision and Pattern Recognition(2022)
摘要
In this paper, we propose a simple yet effective video super-resolution method that aims at generating highfidelity high-resolution (HR) videos from low-resolution (LR) ones. Previous methods predominantly leverage temporal neighbor frames to assist the super-resolution of the current frame. Those methods achieve limited performance as they suffer from the challenges in spatial frame alignment and the lack of useful information from similar LR neighbor frames. In contrast, we devise a cross-frame non-local attention mechanism that allows video superresolution without frame alignment, leading to being more robust to large motions in the video. In addition, to acquire general video prior information beyond neighbor frames, and to compensate for the information loss caused by large motions, we design a novel memory-augmented attention module to memorize general video details during the superresolution training. We have thoroughly evaluated our work on various challenging datasets. Compared to other recent video super-resolution approaches, our method not only achieves significant performance gains on large motion videos but also shows better generalization. Our source code and the new Parkour benchmark dataset is available at https://github.com/jiy173/MANA.
更多查看译文
关键词
Computational photography, Image and video synthesis and generation
AI 理解论文
溯源树
样例
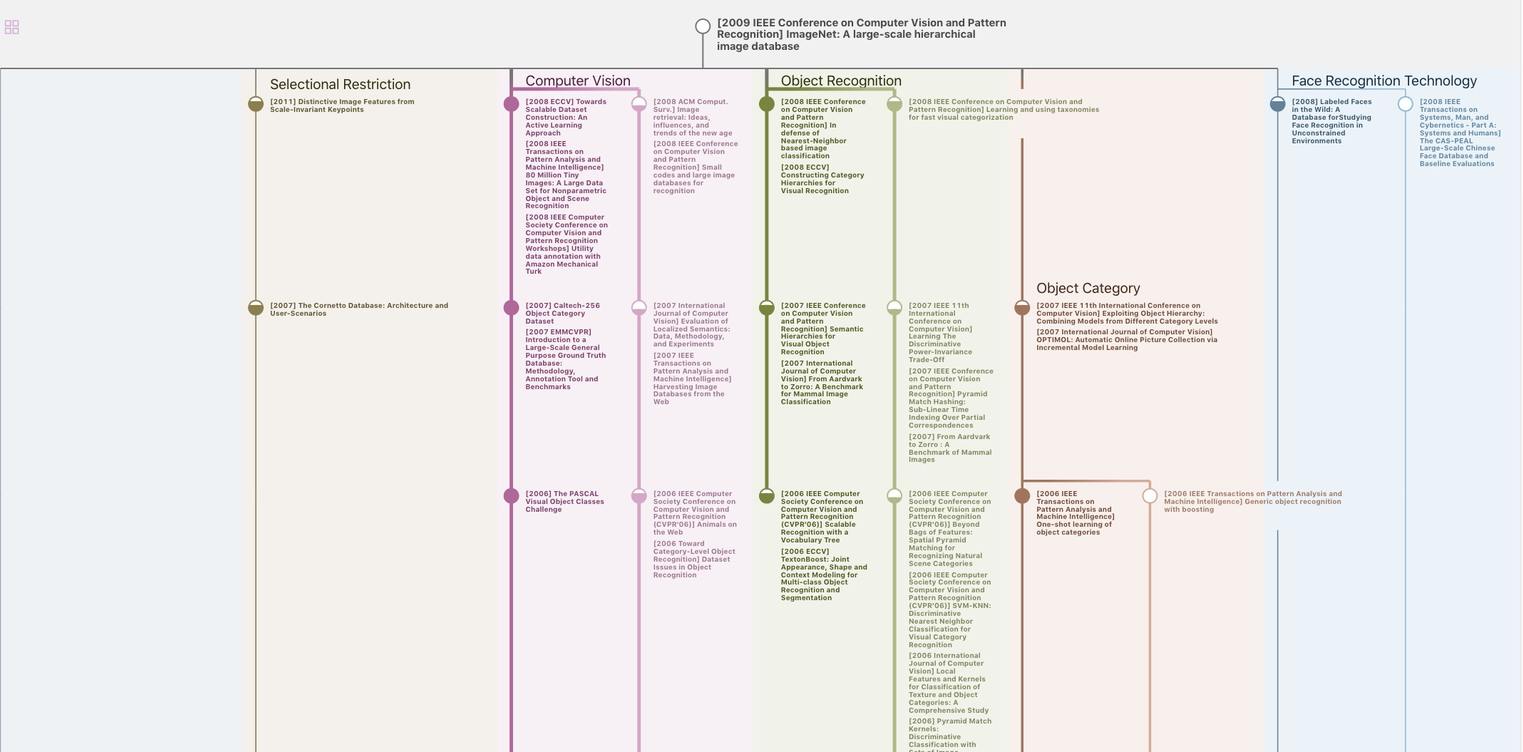
生成溯源树,研究论文发展脉络
Chat Paper
正在生成论文摘要