Using Autoregressive Integrated Moving Average (ARIMA) Modelling to Forecast Symptom Complexity in an Ambulatory Oncology Clinic: Harnessing Predictive Analytics and Patient-Reported Outcomes
International journal of environmental research and public health/International journal of environmental research and public health(2021)
摘要
An increasing incidence of cancer has led to high patient volumes and time challenges in ambulatory oncology clinics. By knowing how many patients are experiencing complex care needs in advance, clinic scheduling and staff allocation adjustments could be made to provide patients with longer or shorter timeslots to address symptom complexity. In this study, we used predictive analytics to forecast the percentage of patients with high symptom complexity in one clinic population in a given time period. Autoregressive integrated moving average (ARIMA) modelling was utilized with patient-reported outcome (PRO) data and patient demographic information collected over 24 weeks. Eight additional weeks of symptom complexity data were collected and compared to assess the accuracy of the forecasting model. The predicted symptom complexity levels were compared with observation data and a mean absolute predicting error of 5.9% was determined, indicating the model's satisfactory accuracy for forecasting symptom complexity levels among patients in this clinic population. By using a larger sample and additional predictors, this model could be applied to other clinics to allow for tailored scheduling and staff allocation based on symptom complexity forecasting and inform system level models of care to improve outcomes and provide higher quality patient care.
更多查看译文
关键词
patient-reported outcomes,symptom complexity levels,forecasting model,predictive analytics,ARIMA,clinic scheduling,staff allocation
AI 理解论文
溯源树
样例
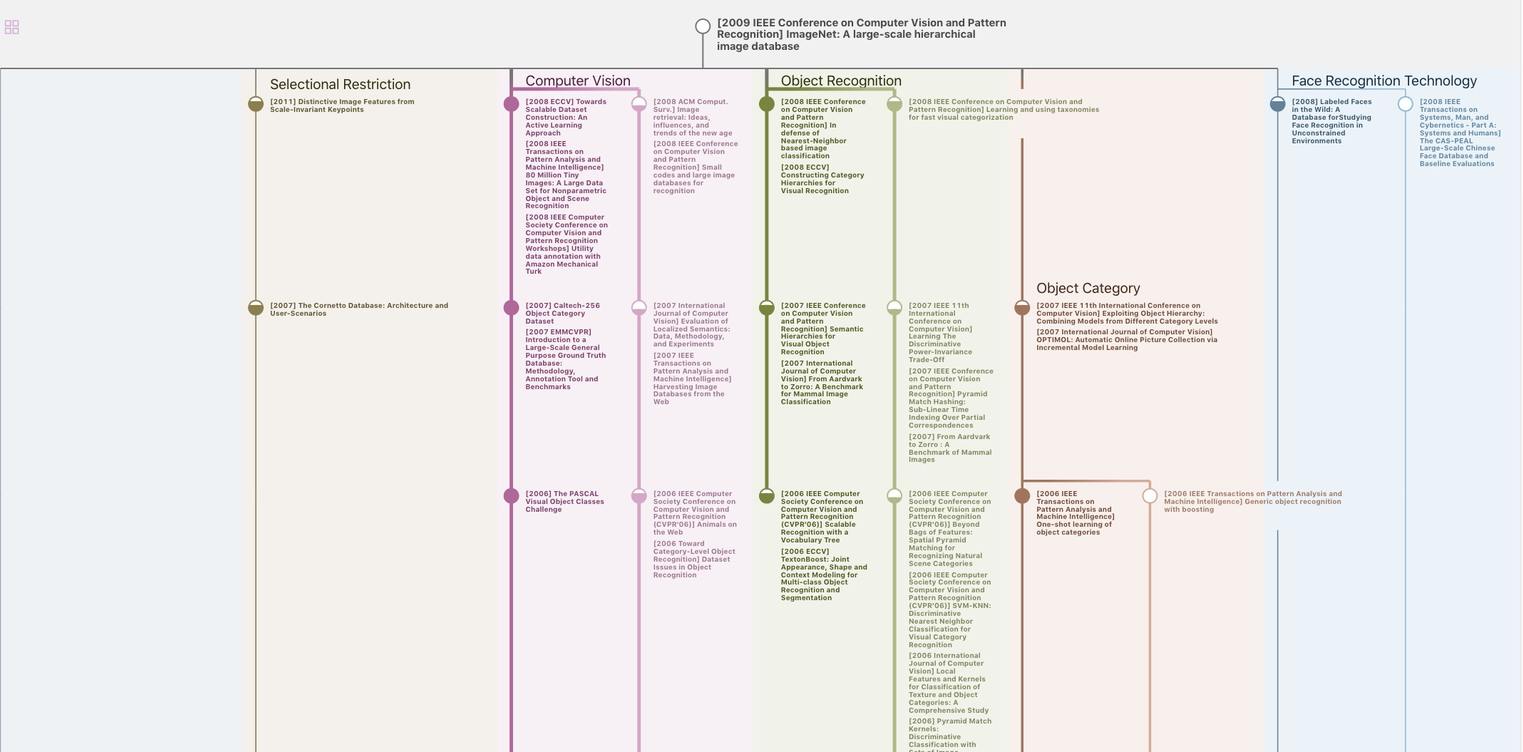
生成溯源树,研究论文发展脉络
Chat Paper
正在生成论文摘要