Explainable machine-learning predictions for complications after pediatric congenital heart surgery
SCIENTIFIC REPORTS(2021)
摘要
The quality of treatment and prognosis after pediatric congenital heart surgery remains unsatisfactory. A reliable prediction model for postoperative complications of congenital heart surgery patients is essential to enable prompt initiation of therapy and improve the quality of prognosis. Here, we develop an interpretable machine-learning-based model that integrates patient demographics, surgery-specific features and intraoperative blood pressure data for accurately predicting complications after pediatric congenital heart surgery. We used blood pressure variability and the k-means algorithm combined with a smoothed formulation of dynamic time wrapping to extract features from time-series data. In addition, SHAP framework was used to provide explanations of the prediction. Our model achieved the best performance both in binary and multi-label classification compared with other consensus-based risk models. In addition, this explainable model explains why a prediction was made to help improve the clinical understanding of complication risk and generate actionable knowledge in practice. The combination of model performance and interpretability is easy for clinicians to trust and provide insight into how they should respond before the condition worsens after pediatric congenital heart surgery.
更多查看译文
关键词
pediatric congenital heart surgery,machine-learning machine-learning,complications
AI 理解论文
溯源树
样例
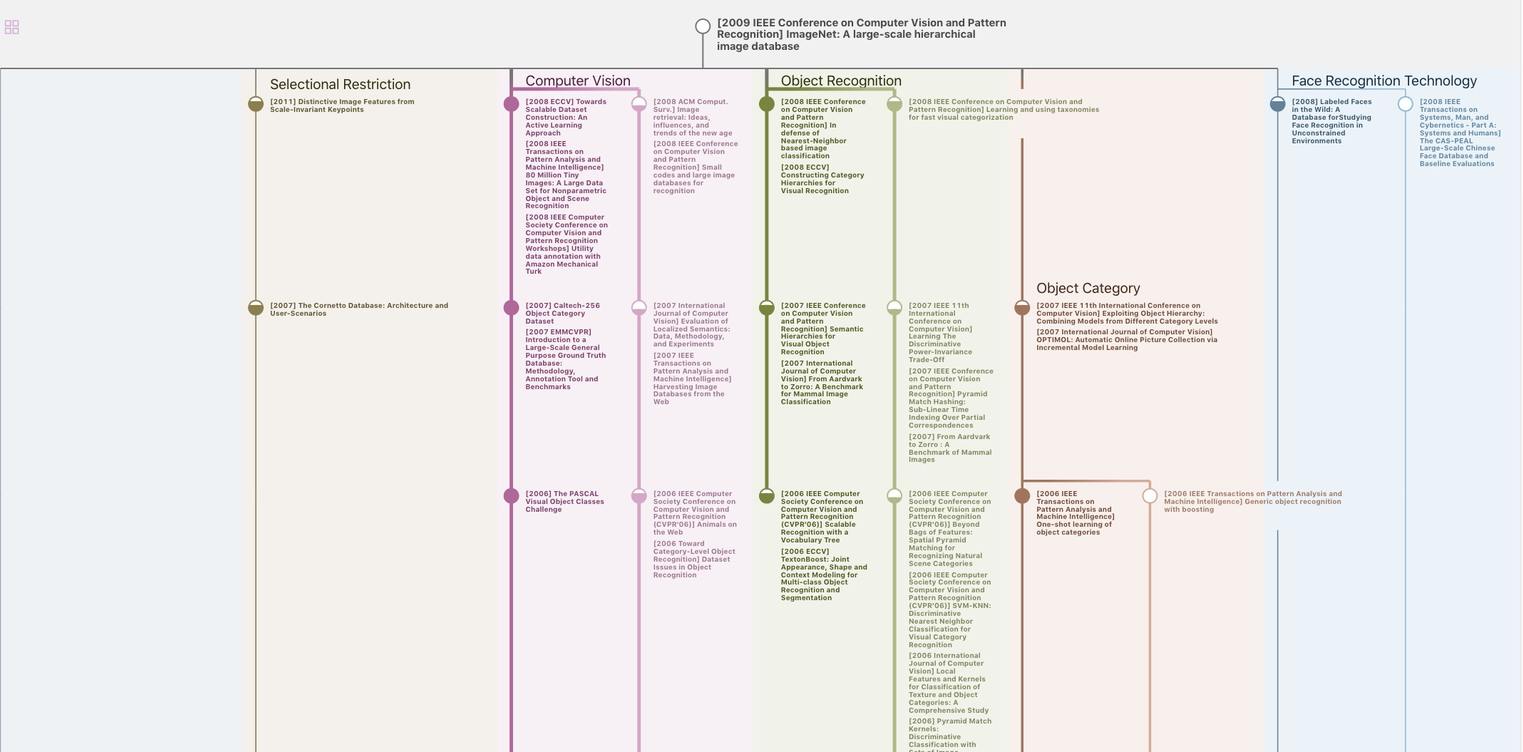
生成溯源树,研究论文发展脉络
Chat Paper
正在生成论文摘要