Robust Probabilistic Simplex Component Analysis
2021 IEEE Statistical Signal Processing Workshop (SSP)(2021)
摘要
Simplex component analysis (SCA) is an important problem that finds diverse applications from hyperspectral imaging to topic mining and community detection. This contribution revisits SCA from a probabilistic point of view, with the aim of dealing with outliers in a disciplined and effective way. Towards this end, a mixture distribution is used to model the data, and the parameters of the mixture model are sought via a maximum likelihood formulation. A variational inference approximation is used to tackle the resulting problem, and the solution enables simultaneous nominal model estimation and outlier detection. Careful simulations with synthetic and semi-real data reveal that the proposed approach offers significantly improved robustness and outlier detection capabilities.
更多查看译文
关键词
Simplex component analysis,outlier,maximum likelihood,variational inference approximation,hyperspectral unmixing
AI 理解论文
溯源树
样例
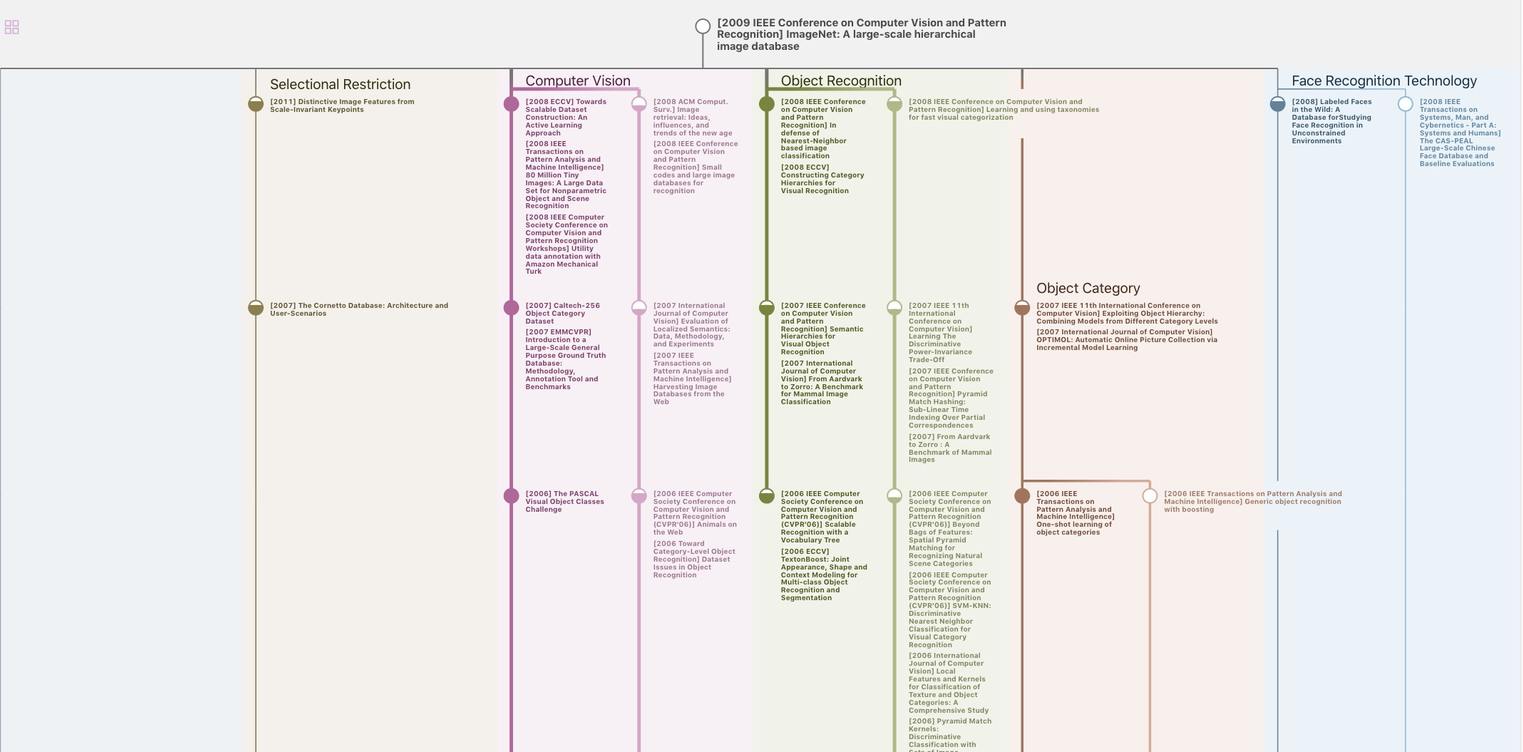
生成溯源树,研究论文发展脉络
Chat Paper
正在生成论文摘要