Weighed ℓ1 on the Simplex: Compressive Sensing Meets Locality
2021 IEEE Statistical Signal Processing Workshop (SSP)(2021)
摘要
Sparse manifold learning algorithms combine techniques in manifold learning and sparse optimization to learn features that could be utilized for downstream tasks. The standard setting of compressive sensing can not be immediately applied to this setup. Due to the intrinsic geometric structure of data, dictionary atoms might be redundant and do not satisfy the restricted isometry property or coherence condition. In addition, manifold learning emphasizes learning local geometry which is not reflected in a standard ℓ
1
minimization problem. We propose weighted ℓ
0
and weighted ℓ
1
regularizations that encourage representation via neighborhood atoms suited for dictionary based manifold learning. Assuming that the data is generated from Delaunay triangulation, we show the equivalence of weighted ℓ
1
and weighted ℓ
0
. We discuss an optimization program that learns the dictionaries and sparse co-efficients and demonstrate the utility of our regularization on synthetic and real datasets.
更多查看译文
关键词
Weighted ℓ1 minimization,compressive sensing,manifold learning,dictionary learning,algorithm un-rolling
AI 理解论文
溯源树
样例
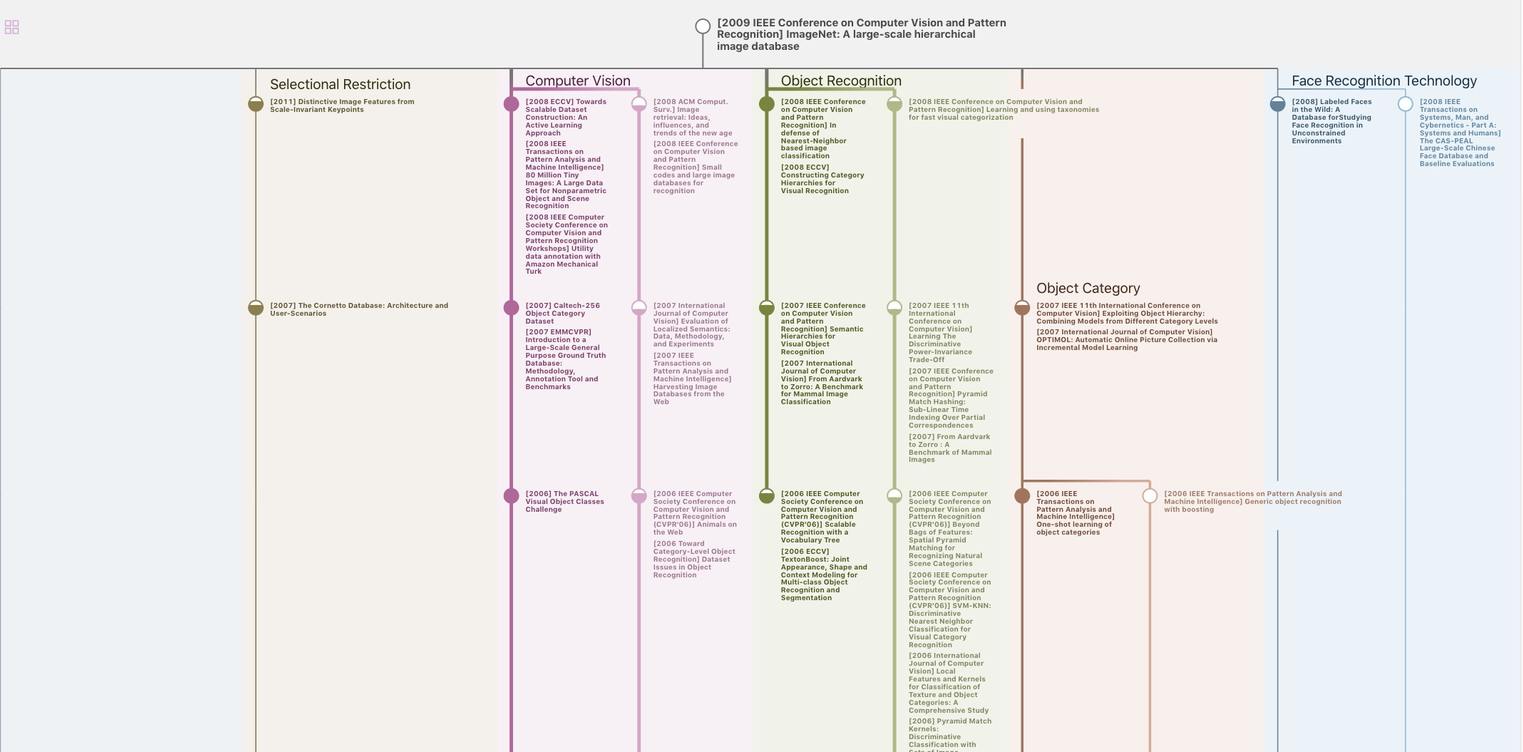
生成溯源树,研究论文发展脉络
Chat Paper
正在生成论文摘要