Joint Learning of Object Detection and Pose Estimation using Augmented Autoencoder
2021 17th International Conference on Machine Vision and Applications (MVA)(2021)
摘要
This paper proposes a method for estimating the pose of a rigid object. While an appearance-based pose estimator requires a bounding box of each target object, an object detector is in general trained independently of the pose estimator. Recent pose estimators are robust to occlusion and image deviation, if the object region is correctly located by the detector. In reality, however, it is difficult to detect correct bounding-boxes, and such erroneous bounding-boxes make pose estimation inaccurate. Our proposed method integrates the object detector and the pose estimator so that they share feature maps and support to each other for improving the pose estimation accuracy. Experimental results demonstrate that the performance of our method is 7.54 times better than the SoTA pose estimation method.
更多查看译文
关键词
object detection,rigid object,appearance-based pose estimator,target object,object detector,object region,SoTA pose estimation method,joint learning,pose estimation,augmented autoencoder,bounding box,feature maps
AI 理解论文
溯源树
样例
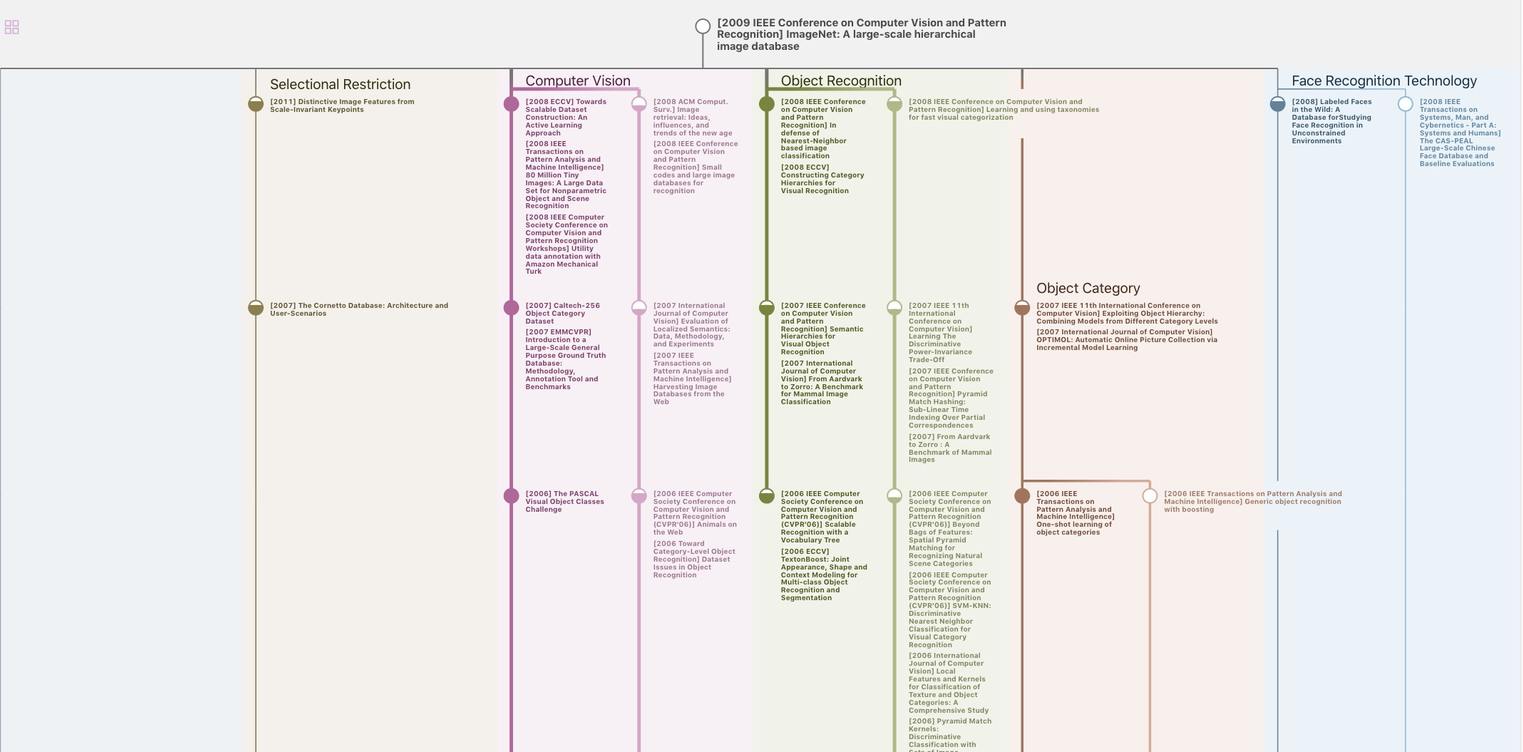
生成溯源树,研究论文发展脉络
Chat Paper
正在生成论文摘要