Distributed Mirror Descent Algorithm With Bregman Damping for Nonsmooth Constrained Optimization
IEEE TRANSACTIONS ON AUTOMATIC CONTROL(2023)
Abstract
To efficiently solve the nonsmooth distributed optimization with both local constraints and coupled constraints, we propose a distributed continuous-time algorithm based on the mirror descent (MD) method. In this article, we introduce the Bregman damping into distributed MD-based dynamics, which not only successfully applies the MD idea to the distributed primal-dual framework, but also ensures the boundedness of all variables and the convergence of the entire dynamics. Our approach generalizes the classic distributed projection-based dynamics, and establishes a connection between MD methods and distributed Euclidean-projected approaches. Also, we prove the convergence of the proposed distributed dynamics with an O(1/t) rate. For practical implementation, we further give a discrete-time algorithm based on the proposed dynamics with an O(1/root k) convergence rate.
MoreTranslated text
Key words
Constrained optimization,distributed algorithm,mirror descent,multi-agent system,nonsmooth.
AI Read Science
Must-Reading Tree
Example
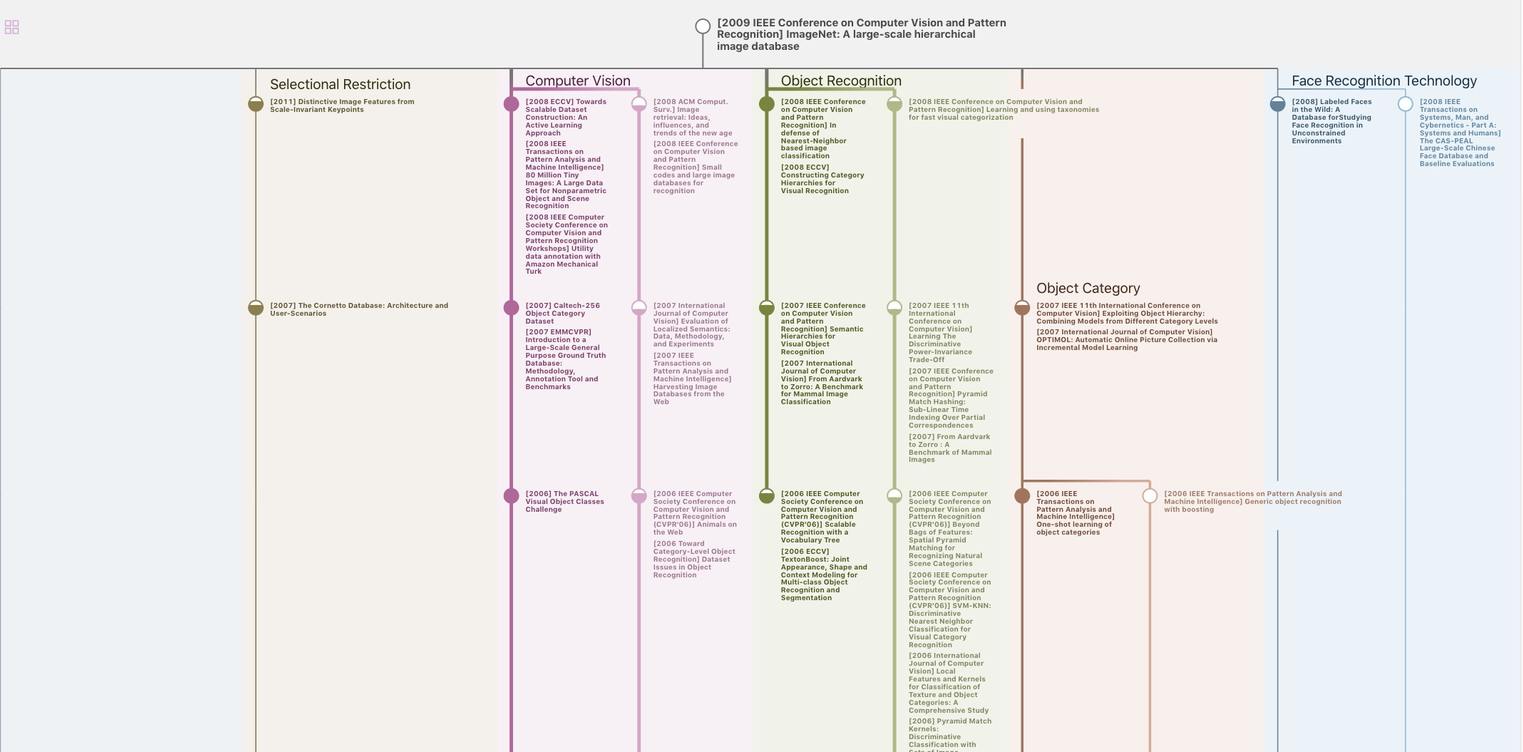
Generate MRT to find the research sequence of this paper
Chat Paper
Summary is being generated by the instructions you defined