Few-Shot Table-to-Text Generation with Prototype Memory.
EMNLP(2021)
摘要
Neural table-to-text generation models have achieved remarkable progress on an array of tasks. However, due to the data-hungry nature of neural models, their performances strongly rely on large-scale training examples, limiting their applicability in real-world applications. To address this, we propose a new framework: Prototype-to-Generate (P2G), for table-to-text generation under the few-shot scenario. The proposed framework utilizes the retrieved prototypes, which are jointly selected by an IR system and a novel prototype selector to help the model bridging the structural gap between tables and texts. Experimental results on three benchmark datasets with three state-of-the-art models demonstrate that the proposed framework significantly improves the model performance across various evaluation metrics.
更多查看译文
关键词
memory,generation,few-shot,table-to-text
AI 理解论文
溯源树
样例
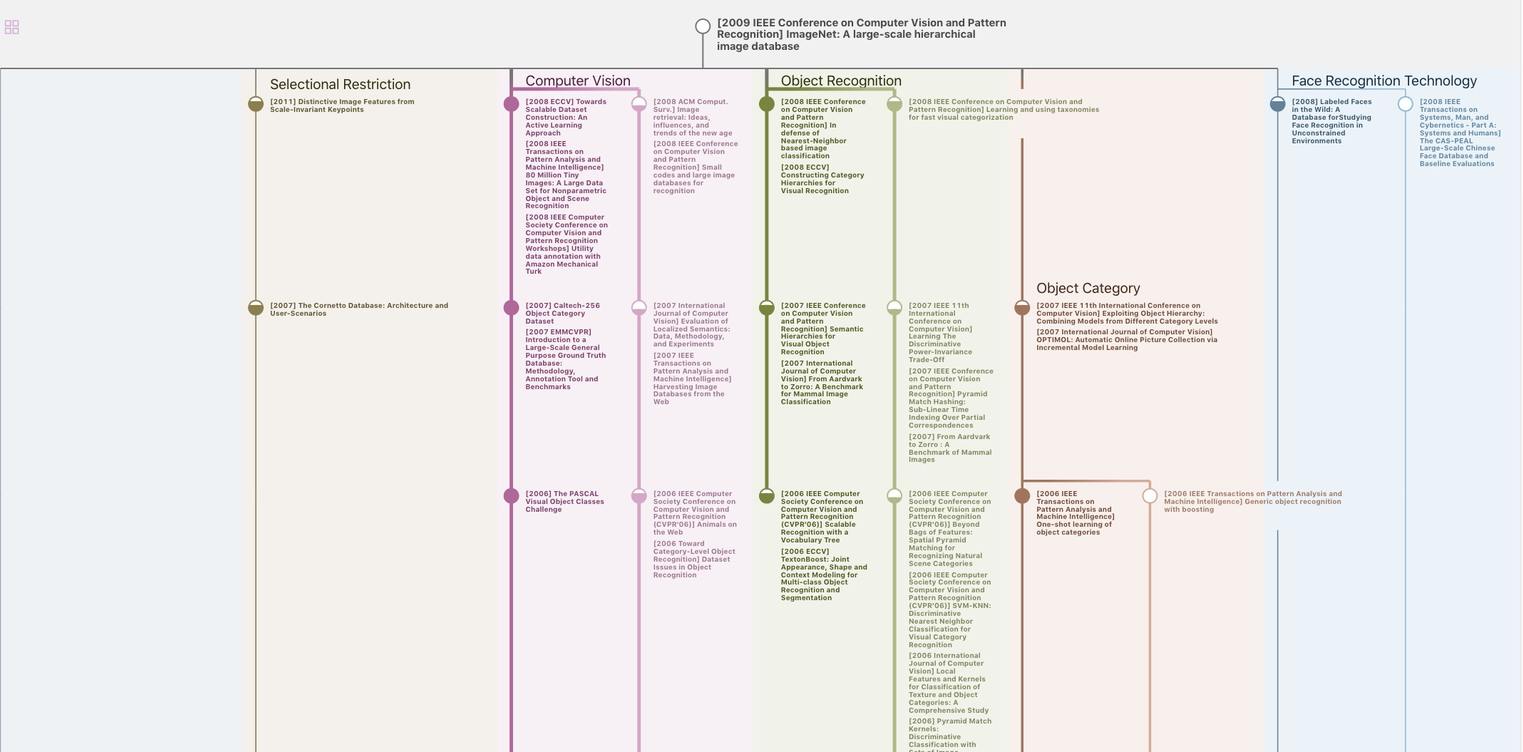
生成溯源树,研究论文发展脉络
Chat Paper
正在生成论文摘要