Variational inference with NoFAS: Normalizing flow with adaptive surrogate for computationally expensive models
Journal of Computational Physics(2022)
摘要
Fast inference of numerical model parameters from data is an important prerequisite to generate predictive models for a wide range of applications. Use of sampling-based approaches such as Markov chain Monte Carlo may become intractable when each likelihood evaluation is computationally expensive. New approaches combining variational inference with normalizing flow are characterized by a computational cost that grows only linearly with the dimensionality of the latent variable space, and rely on gradient-based optimization instead of sampling, providing a more efficient approach for Bayesian inference about the model parameters. Moreover, the cost of frequently evaluating an expensive likelihood can be mitigated by replacing the true model with an offline trained surrogate model, such as neural networks. However, this approach might generate significant bias when the surrogate is insufficiently accurate around the posterior modes. To reduce the computational cost without sacrificing inferential accuracy, we propose Normalizing Flow with Adaptive Surrogate (NoFAS), an optimization strategy that alternatively updates the normalizing flow parameters and surrogate model parameters. We also propose an efficient sample weighting scheme for surrogate model training that preserves global accuracy while effectively capturing high posterior density regions. We demonstrate the inferential and computational superiority of NoFAS against various benchmarks, including cases where the underlying model lacks identifiability. The source code and numerical experiments used for this study are available at https://github.com/cedricwangyu/NoFAS.
更多查看译文
关键词
Variational inference,Normalizing flow,Adaptive surrogate modeling,Memory-aware loss function,Alternated gradient-based optimization
AI 理解论文
溯源树
样例
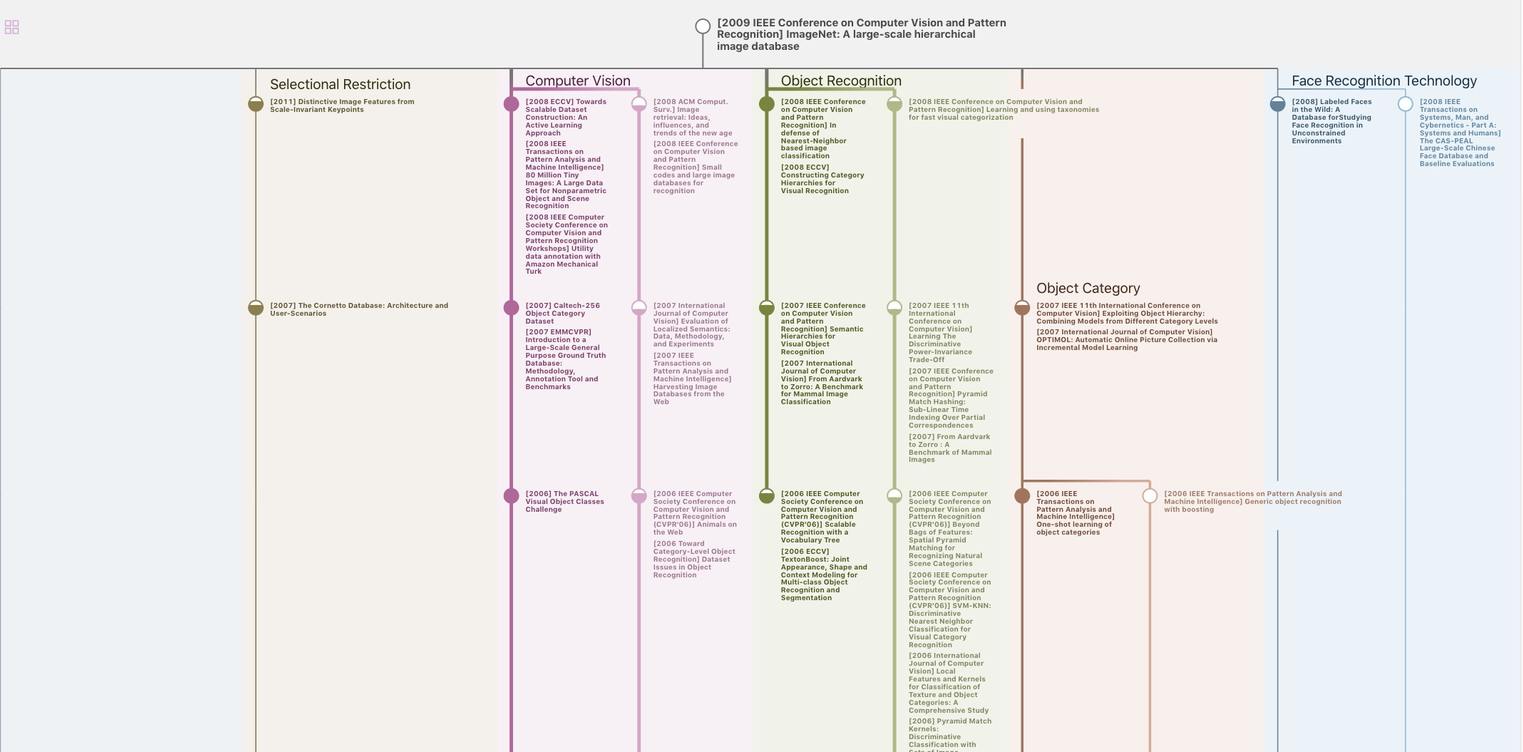
生成溯源树,研究论文发展脉络
Chat Paper
正在生成论文摘要