Evolutionary optimization assisted delayed deep cycle reservoir modeling method with its application to ship heave motion prediction
ISA Transactions(2022)
摘要
As one emerging reservoir modeling method, cycle reservoir with regular jumps (CRJ) provides one effective tool for many time series analysis tasks such as ship heave motion prediction. However, the shallow learning structure of single CRJ model limits its memory capacity and leads to unsatisfactory prediction performance. In order to pursue the stronger dynamic characteristic description of time series data, a delayed deep CRJ model is presented in this paper by integrating the deep learning framework with delay links and the evolutionary optimization for mixed-integer problem. Different from the basic CRJ model with only one reservoir, delayed deep CRJ builds multiple serial reservoirs with inserting the delay links between adjacent reservoirs. Due to the design of dynamic deep learning structure, the memory capacity is enlarged to improve ship heave motion prediction. Aiming at the mix-integer optimization problem in delayed deep CRJ model, a heuristic evolutionary optimization scheme based on the stepwise differential evolution algorithm is applied to determine the delayed deep CRJ parameters automatically. The stepwise differential evolution assisted delayed deep CRJ model can avoid the non-optimal solution resulted from the manual parameter setting effectively. Finally, one numerical example and the real experiment data are utilized to validate the methods and the results demonstrate that delayed deep CRJ model has better prediction performance in contrast to the basic CRJ method.
更多查看译文
关键词
Time series prediction,Cycle reservoir with regular jumps,Deep learning,Differential evolution
AI 理解论文
溯源树
样例
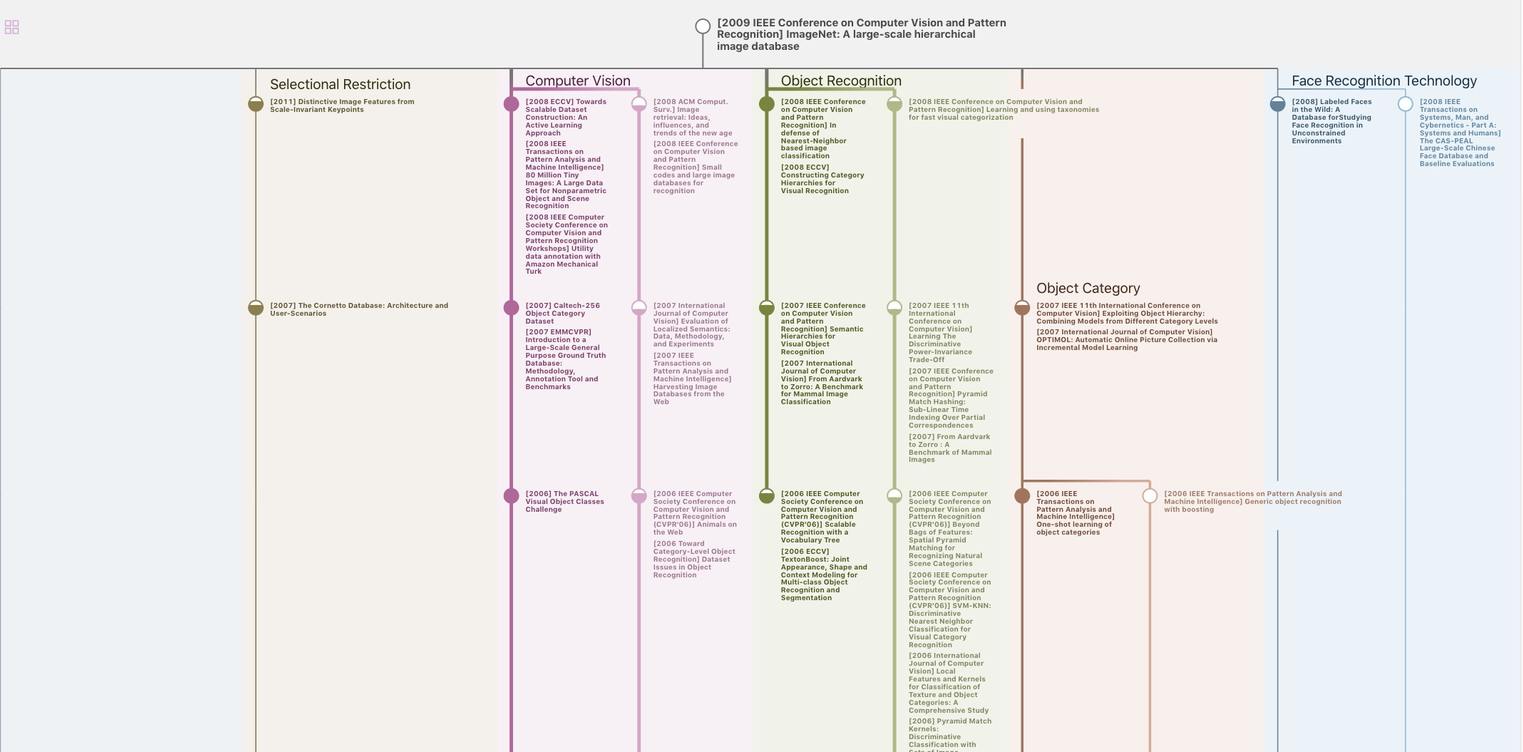
生成溯源树,研究论文发展脉络
Chat Paper
正在生成论文摘要