SMAC-Seg: LiDAR Panoptic Segmentation via Sparse Multi-directional Attention Clustering
IEEE International Conference on Robotics and Automation(2022)
摘要
Panoptic segmentation aims to address semantic and instance segmentation simultaneously in a unified framework. However, an efficient solution of panoptic segmentation in applications like autonomous driving is still an open research problem. In this work, we propose a novel LiDAR-based panoptic system, called SMAC-Seg. We present a learnable sparse multi-directional attention clustering to segment multi-scale foreground instances. SMAC-Seg is a real-time clustering-based approach, which removes the complex proposal network to segment instances. Most existing clustering-based methods use the difference of the predicted and ground truth center offset as the only loss to supervise the instance centroid regression. However, this loss function only considers the centroid of the current object, but its relative position with respect to the neighbouring objects is not considered when learning to cluster. Thus, we propose to use a novel centroid-aware repel loss as an additional term to effectively supervise the network in order to differentiate each object cluster with its neighbours. Our experimental results show that SMAC-Seg achieves state-of-the-art performance among all real-time deployable networks on both large-scale public SemanticKITTI and nuScenes panoptic segmentation datasets.
更多查看译文
关键词
lidar panoptic segmentation,smac-seg,multi-directional
AI 理解论文
溯源树
样例
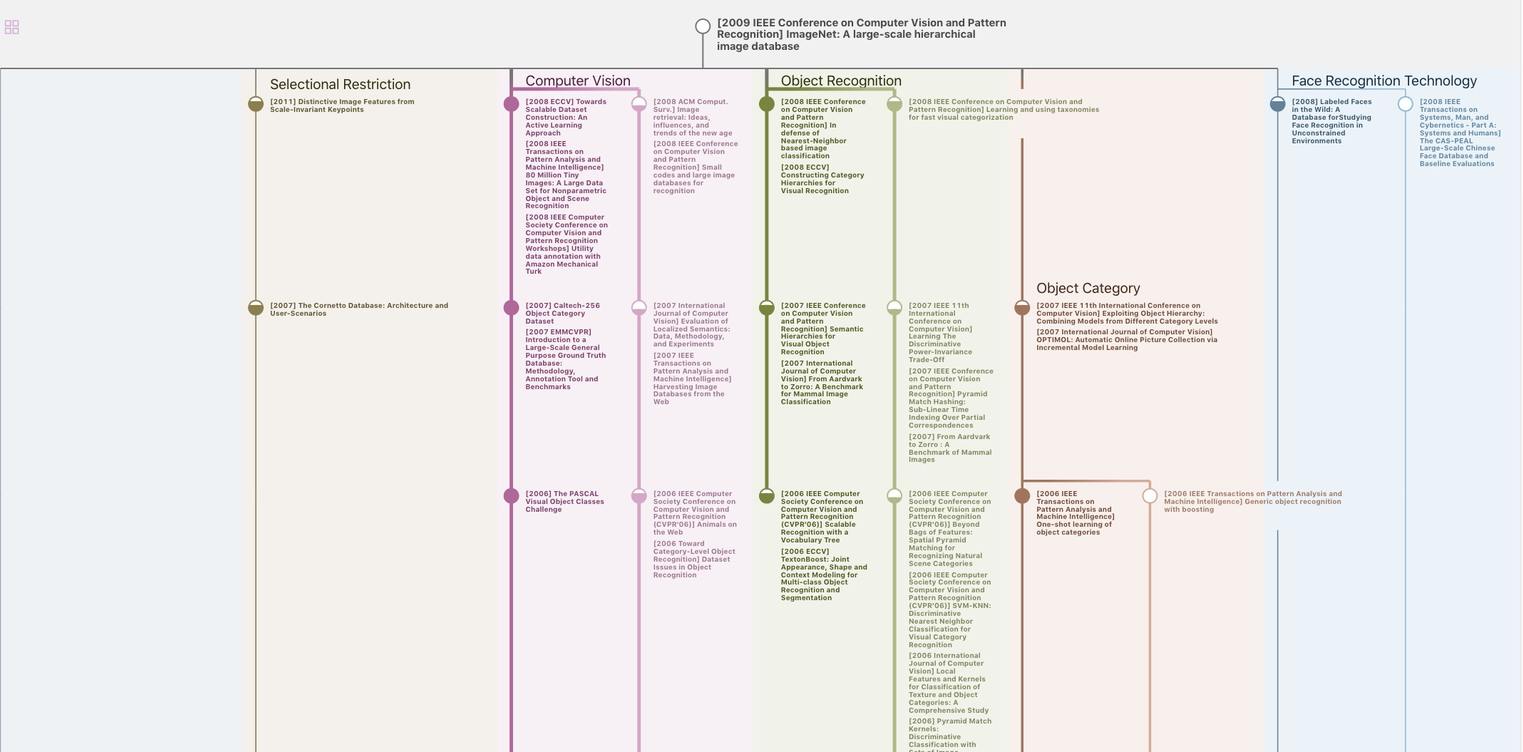
生成溯源树,研究论文发展脉络
Chat Paper
正在生成论文摘要