GRP-FED: Addressing Client Imbalance in Federated Learning via Global-Regularized Personalization
SIAM International Conference on Data Mining (SDM)(2022)
摘要
Since data is presented long-tailed in reality, it is challenging for Federated Learning (FL) to train across decentralized clients as practical applications. We present Global-Regularized Personalization (GRP-FED) to tackle the data imbalanced issue by considering a single global model and multiple local models for each client. With adaptive aggregation, the global model treats multiple clients fairly and mitigates the global long-tailed issue. Each local model is learned from the local data and aligns with its distribution for customization. To prevent the local model from just overfitting, GRP-FED applies an adversarial discriminator to regularize between the learned global-local features. Extensive results show that our GRP-FED improves under both global and local scenarios on real-world MIT-BIH and synthesis CIFAR-10 datasets, achieving comparable performance and addressing client imbalance.
更多查看译文
关键词
federated learning,addressing client imbalance,grp-fed,global-regularized
AI 理解论文
溯源树
样例
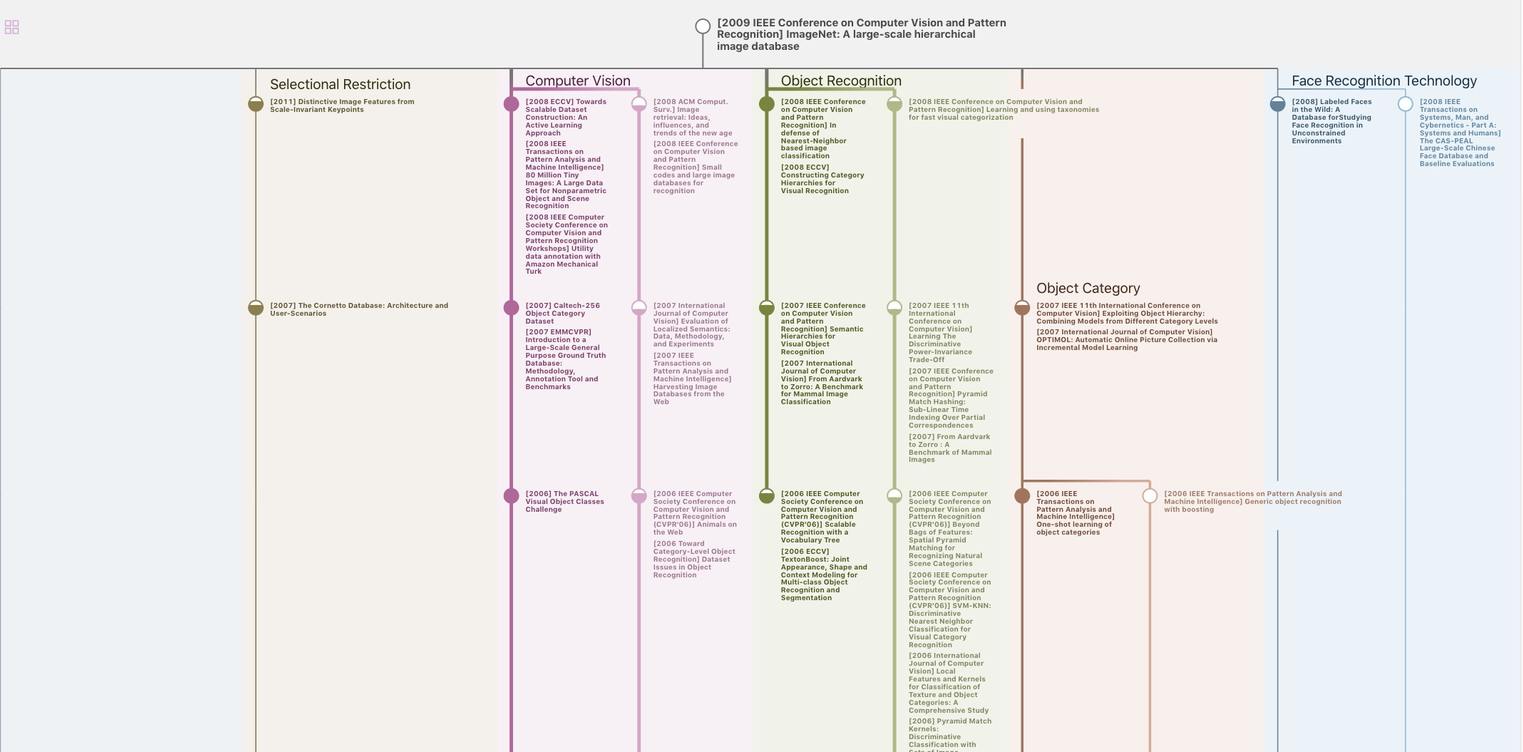
生成溯源树,研究论文发展脉络
Chat Paper
正在生成论文摘要