GFINNs: GENERIC formalism informed neural networks for deterministic and stochastic dynamical systems
PHILOSOPHICAL TRANSACTIONS OF THE ROYAL SOCIETY A-MATHEMATICAL PHYSICAL AND ENGINEERING SCIENCES(2022)
摘要
We propose the GENERIC formalism informed neural networks (GFINNs) that obey the symmetric degeneracy conditions of the GENERIC formalism. GFINNs comprise two modules, each of which contains two components. We model each component using a neural network whose architecture is designed to satisfy the required conditions. The component-wise architecture design provides flexible ways of leveraging available physics information into neural networks. We prove theoretically that GFINNs are sufficiently expressive to learn the underlying equations, hence establishing the universal approximation theorem. We demonstrate the performance of GFINNs in three simulation problems: gas containers exchanging heat and volume, thermoelastic double pendulum and the Langevin dynamics. In all the examples, GFINNs outperform existing methods, hence demonstrating good accuracy in predictions for both deterministic and stochastic systems.This article is part of the theme issue 'Data-driven prediction in dynamical systems'.
更多查看译文
关键词
data-driven discovery, physics-informed neural networks, GENERIC formalism, interpretable scientific machine learning
AI 理解论文
溯源树
样例
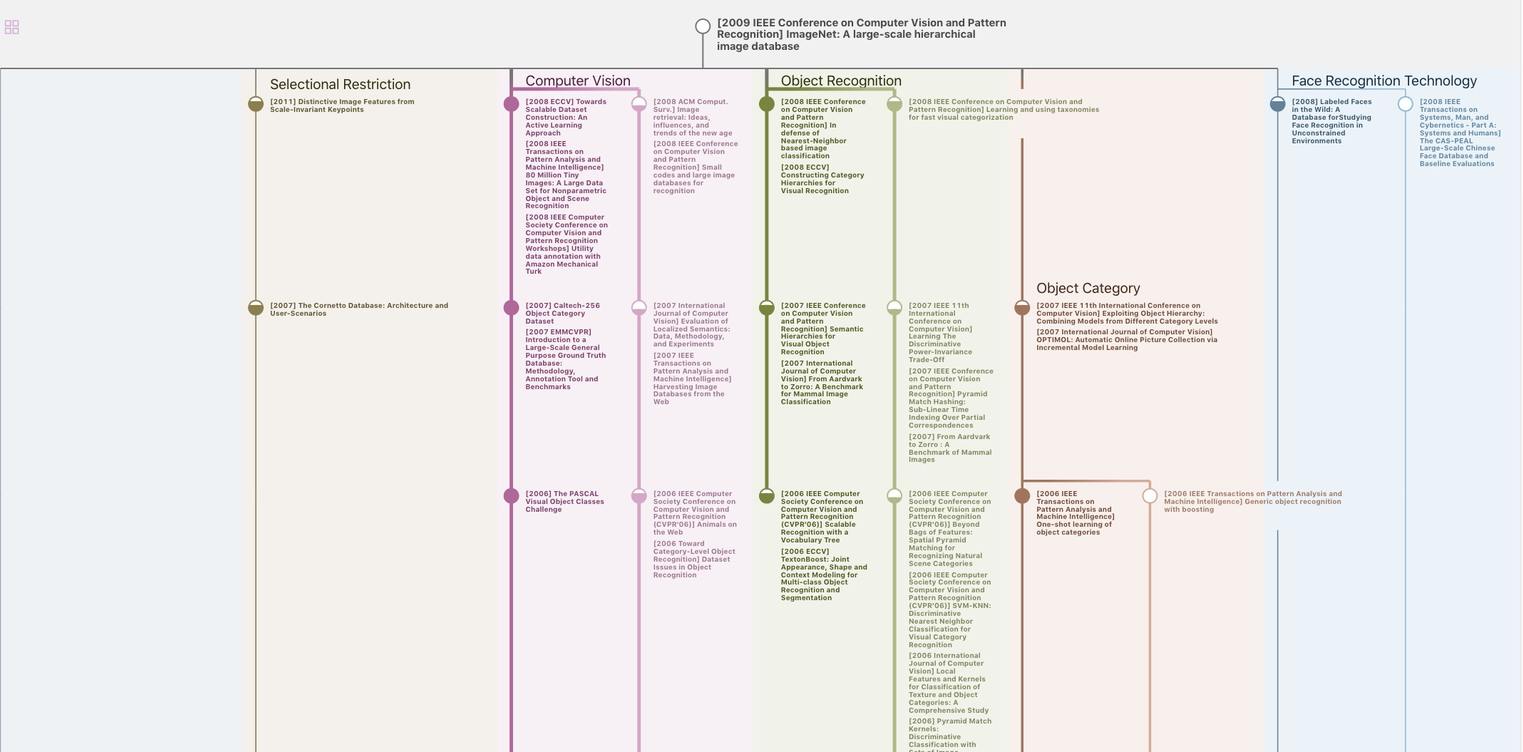
生成溯源树,研究论文发展脉络
Chat Paper
正在生成论文摘要