Deep Learning based Spatial-Temporal In-loop filtering for Versatile Video Coding
2021 IEEE/CVF CONFERENCE ON COMPUTER VISION AND PATTERN RECOGITION WORKSHOPS (CVPRW 2021)(2021)
摘要
The existing deep learning-based Versatile Video Coding (VVC) in-loop filtering (ILF) enhancement works mainly focus on learning the one-to-one mapping between the reconstructed and the original video frame, ignoring the potential resources at encoder and decoder. This work proposes a deep learning-based Spatial-Temporal In-Loop filtering (STILF) that takes advantage of the coding information to improve VVC in-loop filtering. Each CTU is filtered by VVC default in-loop filtering, self-enhancement Convolutional neural network (CNN) with CU map (SEC), and the reference-based enhancement CNN with the optical flow (REO). Bits indicating ILF mode are encoded under CABAC regular mode. Experimental results show that 3.78%, 6.34%, 6%, and 4.64% BD-rate reductions are obtained under All Intra, Low Delay P, Low Delay B, and Random Access configurations, respectively.
更多查看译文
关键词
VVC default in-loop filtering,reference-based enhancement CNN,original video frame,coding information,deep learning-based versatile video coding in-loop filtering,self-enhancement convolutional neural network,ILF,CU map,SEC,REO,optical flow,CABAC regular mode,random access configurations
AI 理解论文
溯源树
样例
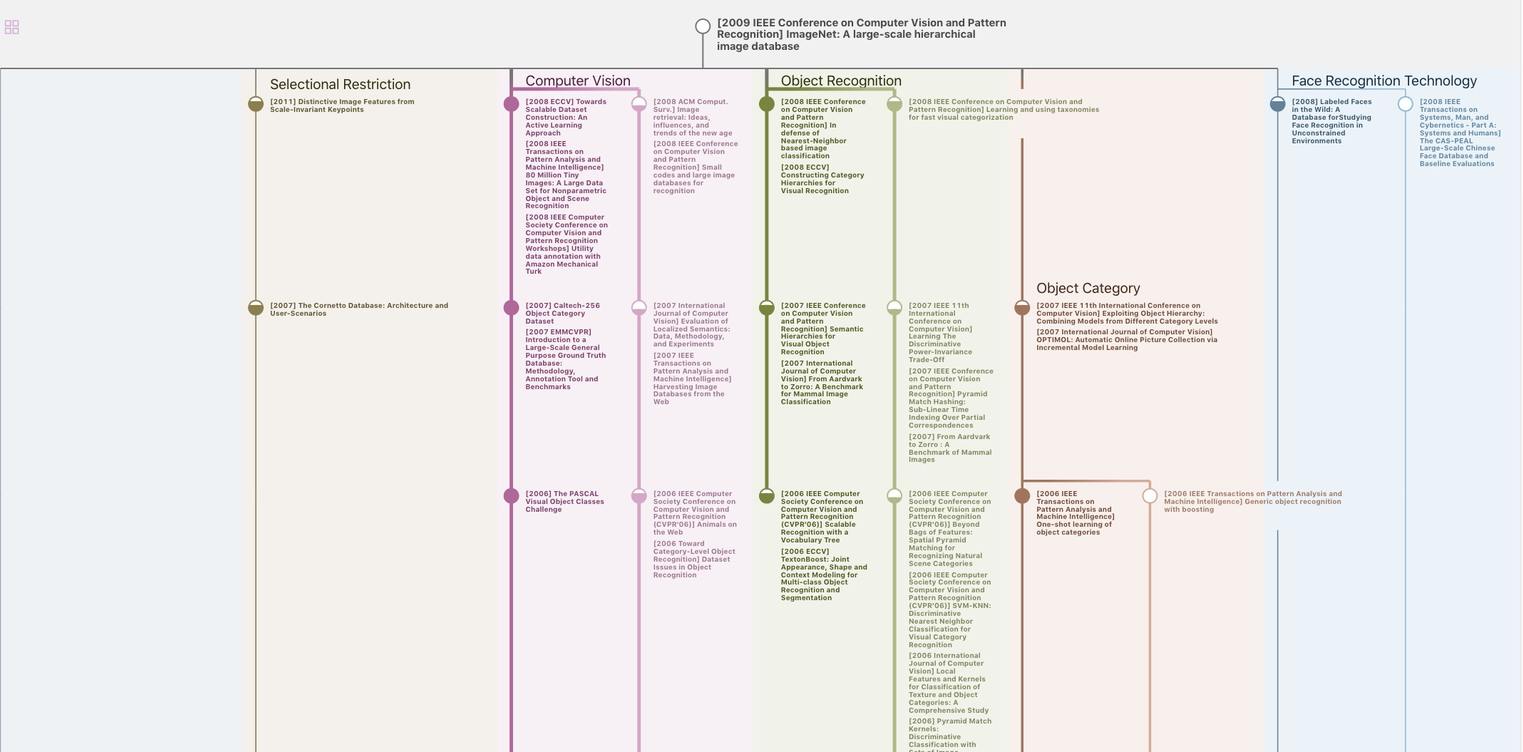
生成溯源树,研究论文发展脉络
Chat Paper
正在生成论文摘要