Active label cleaning for improved dataset quality under resource constraints
NATURE COMMUNICATIONS(2022)
摘要
Imperfections in data annotation, known as label noise, are detrimental to the training of machine learning models and have a confounding effect on the assessment of model performance. Nevertheless, employing experts to remove label noise by fully re-annotating large datasets is infeasible in resource-constrained settings, such as healthcare. This work advocates for a data-driven approach to prioritising samples for re-annotation—which we term “active label cleaning". We propose to rank instances according to estimated label correctness and labelling difficulty of each sample, and introduce a simulation framework to evaluate relabelling efficacy. Our experiments on natural images and on a specifically-devised medical imaging benchmark show that cleaning noisy labels mitigates their negative impact on model training, evaluation, and selection. Crucially, the proposed approach enables correcting labels up to 4 × more effectively than typical random selection in realistic conditions, making better use of experts’ valuable time for improving dataset quality.
更多查看译文
关键词
active label cleaning,dataset quality
AI 理解论文
溯源树
样例
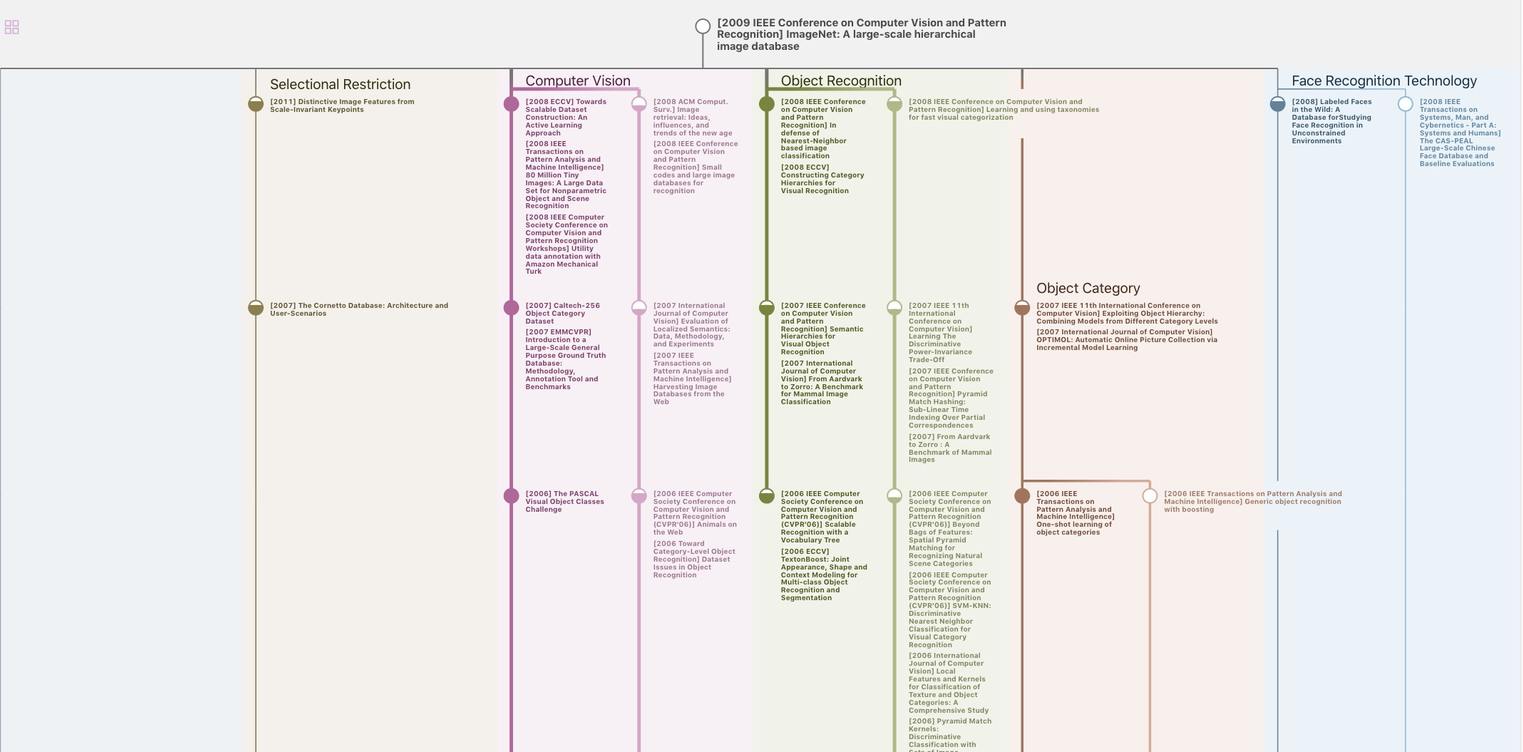
生成溯源树,研究论文发展脉络
Chat Paper
正在生成论文摘要