Multi-Scale Trend Analysis Of Water Quality Using Error Propagation Of Generalized Additive Models
SCIENCE OF THE TOTAL ENVIRONMENT(2022)
摘要
Effective stewardship of ecosystems to sustain current ecological status or mitigate impacts requires nuanced understanding of how conditions have changed over time in response to anthropogenic pressures and natural variability. Detecting and appropriately characterizing changes requires accurate and flexible trend assessment methods that can be readily applied to environmental monitoring datasets. A key requirement is complete propagation of uncertainty through the analysis. However, this is difficult when there are mismatches between sampling frequency, period of record, and trends of interest. Here, we propose a novel application of generalized additive models (GAMs) for characterizing multi-decadal changes in water quality indicators and demonstrate its utility by analyzing a 30-year record of biweekly-to-monthly chlorophyll-a concentrations in the San Francisco Estuary. GAMs have shown promise in water quality trend analysis to separate long-term (i.e., annual or decadal) trends from seasonal variation. Our proposed methods estimate seasonal averages in a response variable with GAMs, extract uncertainty measures for the seasonal estimates, and then use the uncertainty measures with mixed-effects meta-analysis regression to quantify inter-annual trends that account for full propagation of error across methods. We first demonstrate that nearly identical descriptions of temporal changes can be obtained using different smoothing spline formulations of the original time series. We then extract seasonal averages and their standard errors for an a priori time period within each year from the GAM results. Finally, we demonstrate how across-year trends in seasonal averages can be modeled with mixed-effects metaanalysis regression that propagates uncertainties from the GAM fits to the across-year analysis. Overall, this
更多查看译文
关键词
Chlorophyll, Generalized additive models, meta-analysis, San Francisco estuary, Trend analysis
AI 理解论文
溯源树
样例
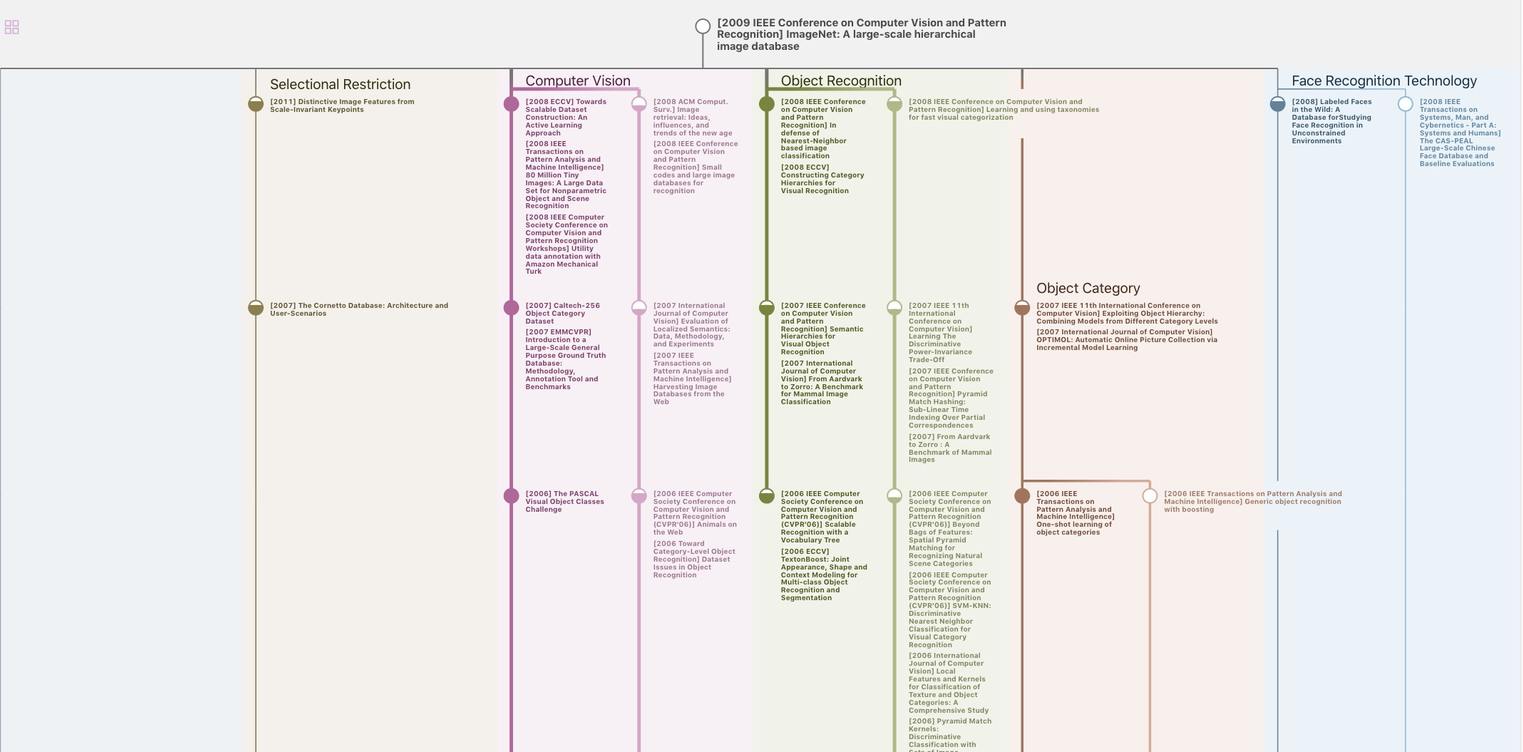
生成溯源树,研究论文发展脉络
Chat Paper
正在生成论文摘要