Inductive and irregular dynamic network representation based on ordinary differential equations
Knowledge-Based Systems(2021)
摘要
Dynamic network representation has aroused increasing attention from researchers since it reflects the characteristics of real-world networks and has been widely used in various applications. Enormous efforts have been made to this problem. Existing studies often use fixed-time steps. However, in fact, the dynamics in real-world networks are usually irregular over time. To address this problem, in this paper, we employ ordinary differential equations and propose a novel graph representation approach for dynamic networks, namely Graph-ODE. The core idea lies in that it leverages ordinary differential equations to model the continuous latent dynamics of the network and therefore can naturally capture the evolution pattern. Specifically, to deal with the irregularity of node interactions, we first propose a differential form of GRU aggregator to aggregate the chronological neighbors. In addition, we employ neighbor sampling strategies for efficiency. Besides, our inductive algorithm owns the ability to deal with new emerging nodes. Experimental results on various real-world data sets demonstrate that our method significantly outperforms several state-of-the-art algorithms on downstream tasks.
更多查看译文
关键词
Dynamic network representation,Inductive learning,Irregular dynamics
AI 理解论文
溯源树
样例
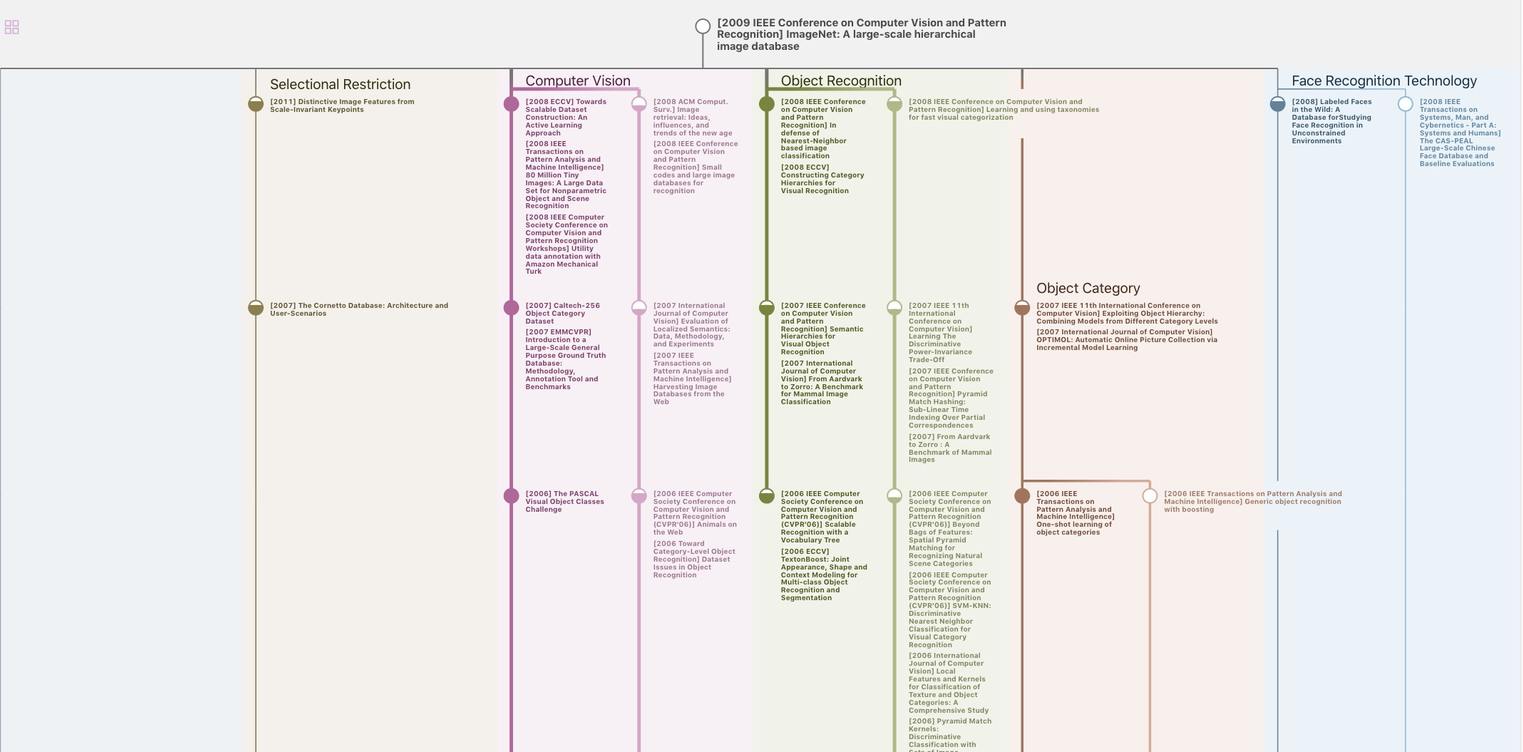
生成溯源树,研究论文发展脉络
Chat Paper
正在生成论文摘要