Graph Robustness Benchmark: Rethinking and Benchmarking Adversarial Robustness of Graph Neural Networks
user-5f925d0d4c775ec6fa69c404(2021)
摘要
Recent studies have shown that Graph Neural Networks (GNNs) are vulnerable to 1 adversarial attacks. Previous attacks and defenses on GNNs face common prob2 lems like scalability or generality, which hinder the progress of this domain. By 3 rethinking limitations in previous works, we propose Graph Robustness Benchmark 4 (GRB), the first benchmark that aims to provide scalable, general, unified, and 5 reproducible evaluation on adversarial robustness of GNNs. GRB includes (1) 6 scalable datasets processed by a novel splitting scheme; (2) diverse set of baseline 7 methods covering GNNs, attacks, and defenses; (3) unified evaluation pipeline that 8 permits a fair comparison; (4) modular coding framework that facilitates imple9 mentation of various methods and ensures reproducibility; (5) leaderboards that 10 track the progress of the field. Besides, we propose two strong baseline defenses 11 that significantly outperform previous ones. With extensive experiments, we can 12 fairly compare all methods and investigate their pros and cons. GRB is open-source 13 and maintains all datasets, codes, leaderboards at https://cogdl.ai/grb/home, 14 which will be continuously updated to promote future research in this field. 15
更多查看译文
关键词
Graph (abstract data type),Robustness (computer science),Benchmark (computing),Benchmarking,Theoretical computer science,Computer science,Adversarial system,Graph neural networks
AI 理解论文
溯源树
样例
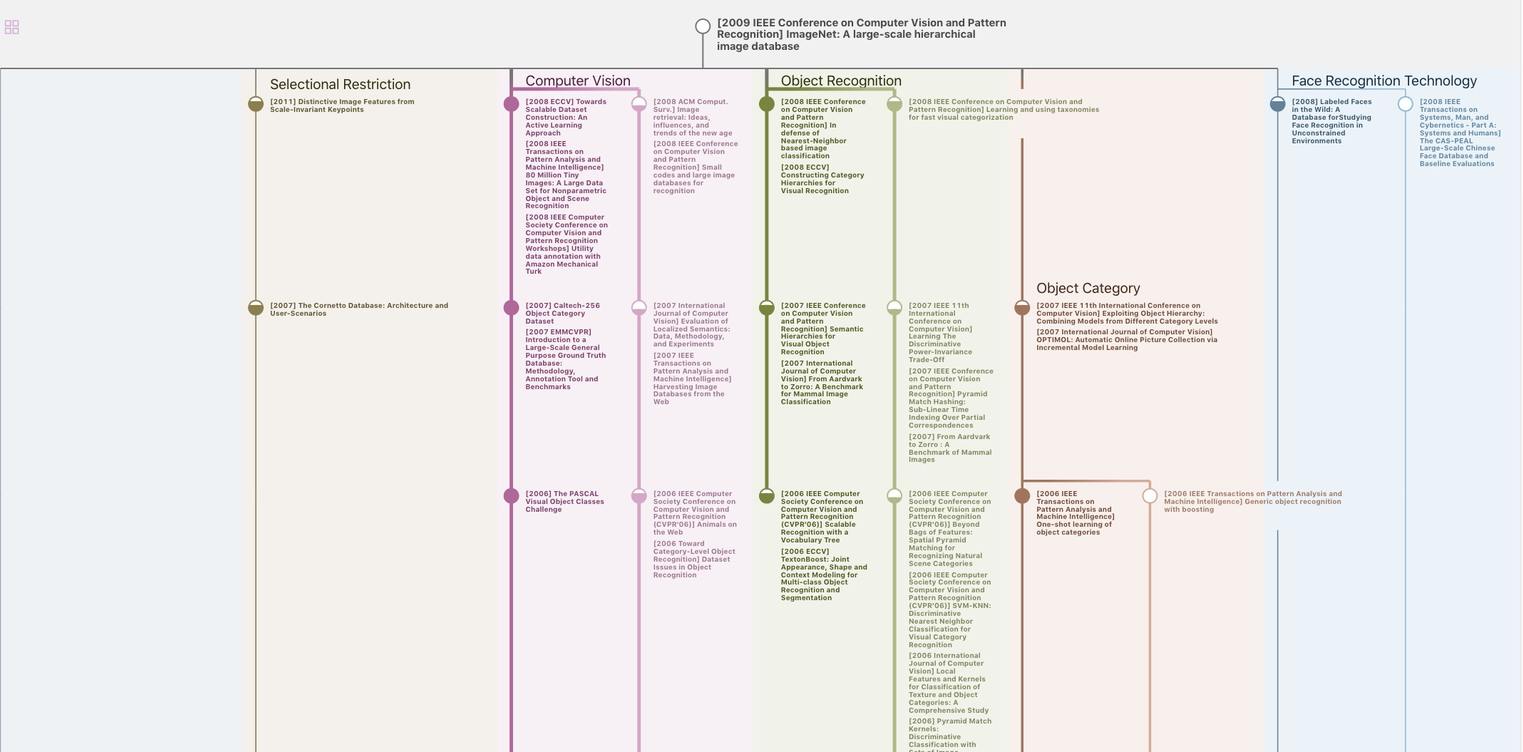
生成溯源树,研究论文发展脉络
Chat Paper
正在生成论文摘要