Task-dependent neural representations of visual object categories
EUROPEAN JOURNAL OF NEUROSCIENCE(2021)
摘要
What do we perceive in a glance of an object? If we are questioned about it, will our perception be affected? How does the task demand influence visual processing in the brain and, consequently, our behaviour? To address these questions, we conducted an object categorisation experiment with three tasks, one at the superordinate level ('animate/inanimate') and two at the basic levels ('face/body' and 'animal/human face') along with a passive task in which participants were not required to categorise objects. To control bottom-up information and eliminate the effect of sensory-driven dissimilarity, we used a particular set of animal face images as the identical target stimuli across all tasks. We then investigated the impact of top-down task demands on behaviour and brain representations. Behavioural results demonstrated a superordinate advantage in the reaction time, while the accuracy was similar for all categorisation levels. The event-related potentials (ERPs) for all categorisation levels were highly similar except for about 170 ms and after 300 ms from stimulus onset. In these time windows, the animal/human face categorisation, which required fine-scale discrimination, elicited a differential ERP response. Similarly, decoding analysis over all electrodes showed the highest peak value of task decoding around 170 ms, followed by a few significant timepoints, generally after 300 ms. Moreover, brain responses revealed task-related neural modulation during categorisation tasks compared with the passive task. Overall, these findings demonstrate different task-related effects on the behavioural response and brain representations. The early and late components of neural modulation could be linked to perceptual and top-down processing of object categories, respectively.
更多查看译文
关键词
behavioural responses, event-related potentials (ERPs), levels of object categorisation, N170, task-related information
AI 理解论文
溯源树
样例
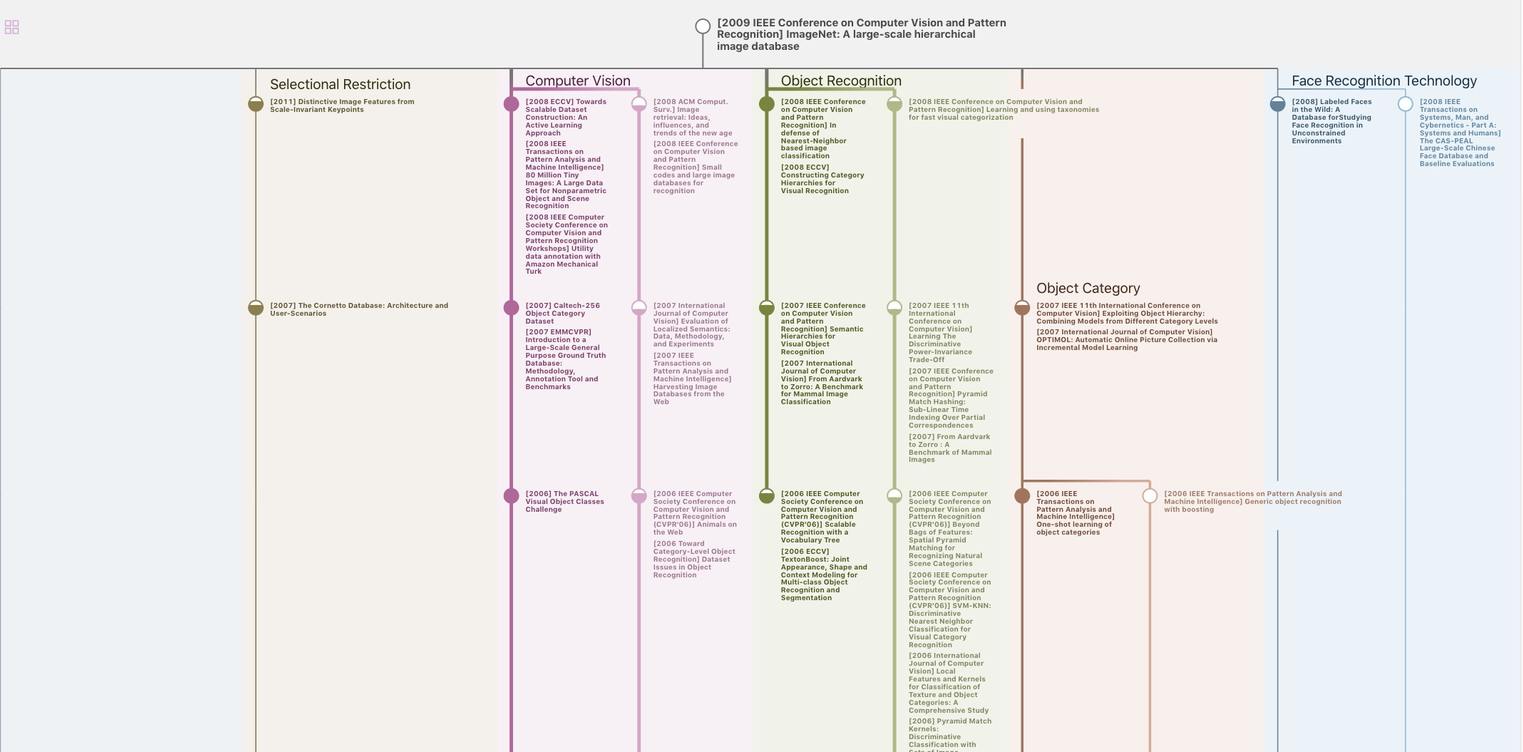
生成溯源树,研究论文发展脉络
Chat Paper
正在生成论文摘要