A Bayesian approach to (online) transfer learning: Theory and algorithms?
arxiv(2023)
摘要
Transfer learning is a machine learning paradigm where knowledge from one problem is utilized to solve a new but related problem. While conceivable that knowledge from one task could help solve a related task, if not executed properly, transfer learning algorithms can impair the learning performance instead of improving it - commonly known as negative transfer. In this paper, we use a parametric statistical model to study transfer learning from a Bayesian perspective. Specifically, we study three variants of transfer learning problems, instantaneous, online, and time-variant transfer learning. We define an appropriate objective function for each problem and provide either exact expressions or upper bounds on the learning performance using information-theoretic quantities, which allow simple and explicit characterizations when the sample size becomes large. Furthermore, examples show that the derived bounds are accurate even for small sample sizes. The obtained bounds give valuable insights into the effect of prior knowledge on transfer learning, at least with respect to our Bayesian formulation of the transfer learning problem. In particular, we formally characterize the conditions under which negative transfer occurs. Lastly, we devise several (online) transfer learning algorithms that are amenable to practical implementations, some of which do not require the parametric assumption. We demonstrate the effectiveness of our algorithms with real data sets, focusing primarily on when the source and target data have strong similarities.& COPY; 2023 The Author(s). Published by Elsevier B.V. This is an open access article under the CC BY-NC license (http://creativecommons .org /licenses /by-nc /4 .0/).
更多查看译文
关键词
Transfer learning,Conditional mutual information,Prior information,Negative transfer
AI 理解论文
溯源树
样例
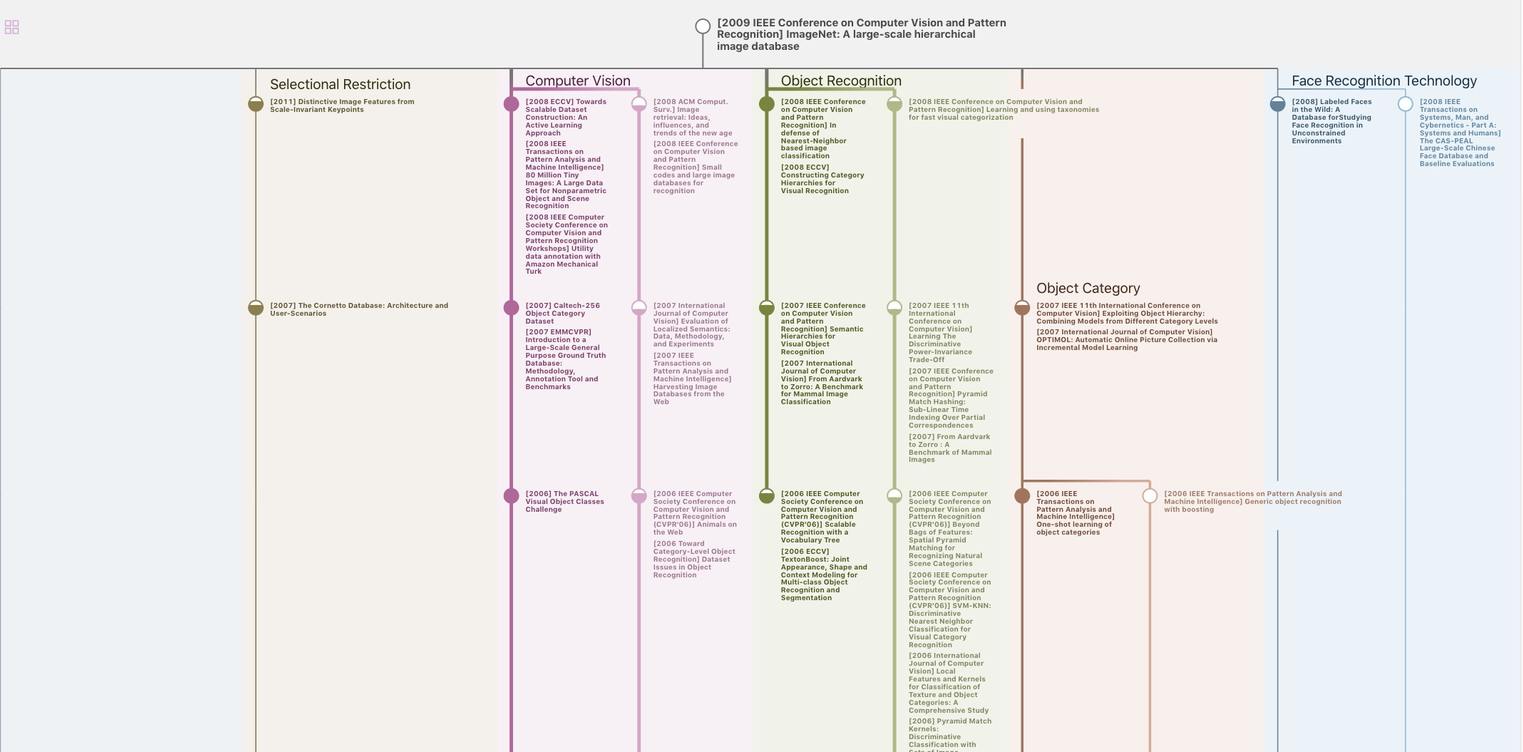
生成溯源树,研究论文发展脉络
Chat Paper
正在生成论文摘要