Iatbp-Hyb-Enc: Prediction Of Antitubercular Peptides Via Heterogeneous Feature Representation And Genetic Algorithm Based Ensemble Learning Model
COMPUTERS IN BIOLOGY AND MEDICINE(2021)
摘要
Tuberculosis (TB) is a worldwide illness caused by the bacteria Mycobacterium tuberculosis. Owing to the high prevalence of multidrug-resistant tuberculosis, numerous traditional strategies for developing novel alternative therapies have been presented. The effectiveness and dependability of these procedures are not always consistent. Peptide-based therapy has recently been regarded as a preferable alternative due to its excellent selectivity in targeting specific cells without affecting the normal cells. However, due to the rapid growth of the peptide samples, predicting TB accurately has become a challenging task. To effectively identify antitubercular peptides, an intelligent and reliable prediction model is indispensable. An ensemble learning approach was used in this study to improve expected results by compensating for the shortcomings of individual classification algorithms. Initially, three distinct representation approaches were used to formulate the training samples: k-space amino acid composition, composite physiochemical properties, and one-hot encoding. The feature vectors of the applied feature extraction methods are then combined to generate a heterogeneous vector. Finally, utilizing individual and heterogeneous vectors, five distinct nature classification models were used to evaluate prediction rates. In addition, a genetic algorithm-based ensemble model was used to improve the suggested model's prediction and training capabilities. Using Training and independent datasets, the proposed ensemble model achieved an accuracy of 94.47% and 92.68%, respectively. It was observed that our proposed "iAtbP-Hyb-EnC" model outperformed and reported similar to 10% highest training accuracy than existing predictors. The "iAtbP-Hyb-EnC" model is suggested to be a reliable tool for scientists and might play a valuable role in academic research and drug discovery. The source code and all datasets are publicly available at https://github.com/Farman335/iAtbP-Hyb-EnC.
更多查看译文
关键词
Antitubercular peptides, Ensemble classification, Genetic algorithm, One-hot encoding, Composite physiochemical properties, k-fold cross-validation test
AI 理解论文
溯源树
样例
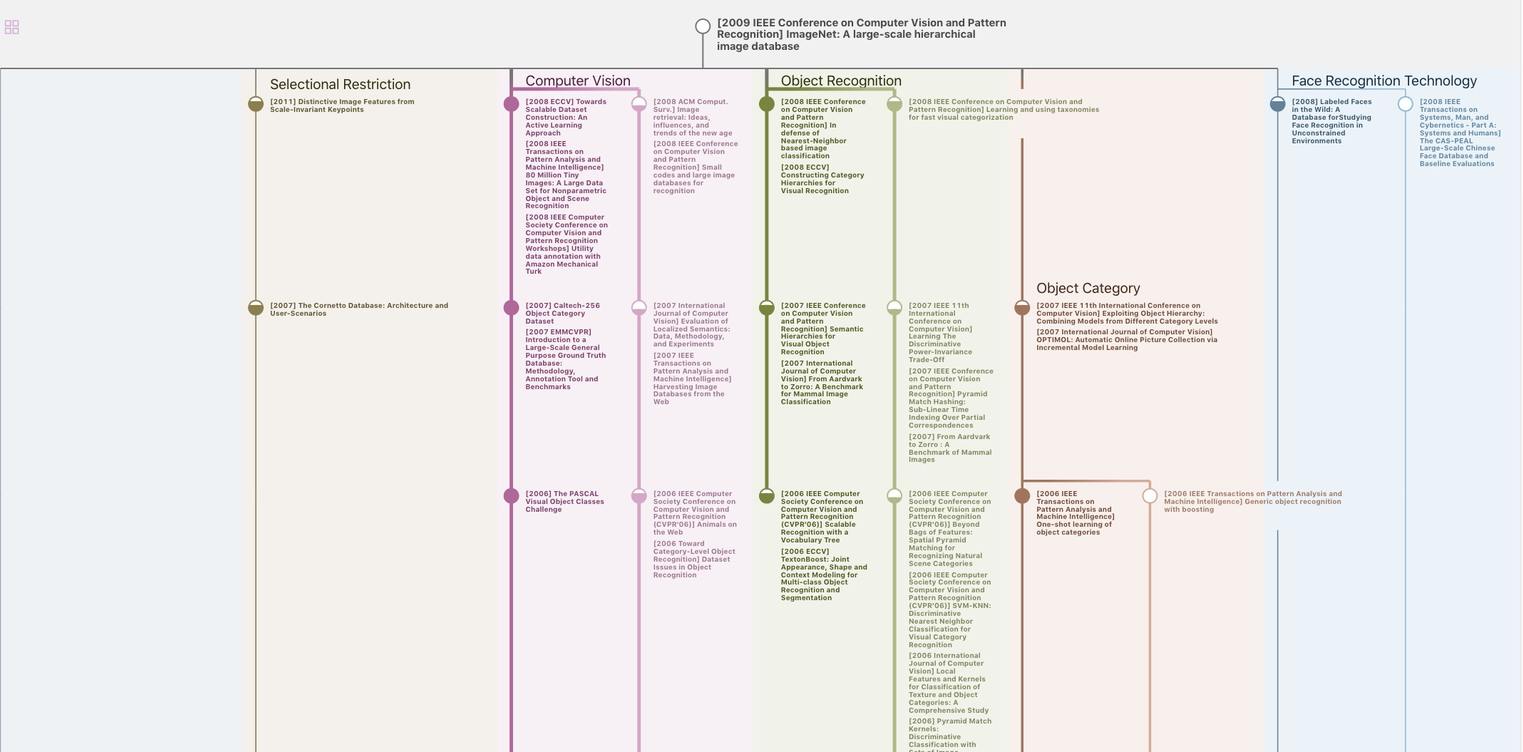
生成溯源树,研究论文发展脉络
Chat Paper
正在生成论文摘要