An adaptive Gaussian mixture method for nonlinear uncertainty propagation in neural networks
Neurocomputing(2021)
摘要
Using neural networks to address data-driven problems often entails dealing with uncertainties. However, the propagation of uncertainty through a network's nonlinear layers is usually a bottleneck, since the existing techniques designed to transmit Gaussian distributions via moment estimation are not capable of predicting non-Gaussian distributions. In this study, a Gaussian-mixture-based uncertainty propagation scheme is proposed for neural networks. Given that any input uncertainty can be characterized as a Gaussian mixture with a finite number of components, the developed scheme actively examines each mixture component and adaptively split those whose fidelity in representing uncertainty is deteriorated by the network's nonlinear activation layers. A Kullback-Leibler criterion that directly measures the nonlinearity-induced non-Gaussianity in post-activation distributions is derived to trigger splitting and a set of high-precision Gaussian splitting libraries is established. Four uncertainty propagation examples on dynamic systems and data-driven applications are demonstrated, in all of which the developed scheme exhibited exemplary fidelity and efficiency in predicting the evolution of non Gaussian distributions through both recurrent and multi-layer neural networks. (c) 2021 Elsevier B.V. All rights reserved.
更多查看译文
关键词
Neural networks,Nonlinear uncertainty propagation,Gaussian mixture model,Dynamic systems
AI 理解论文
溯源树
样例
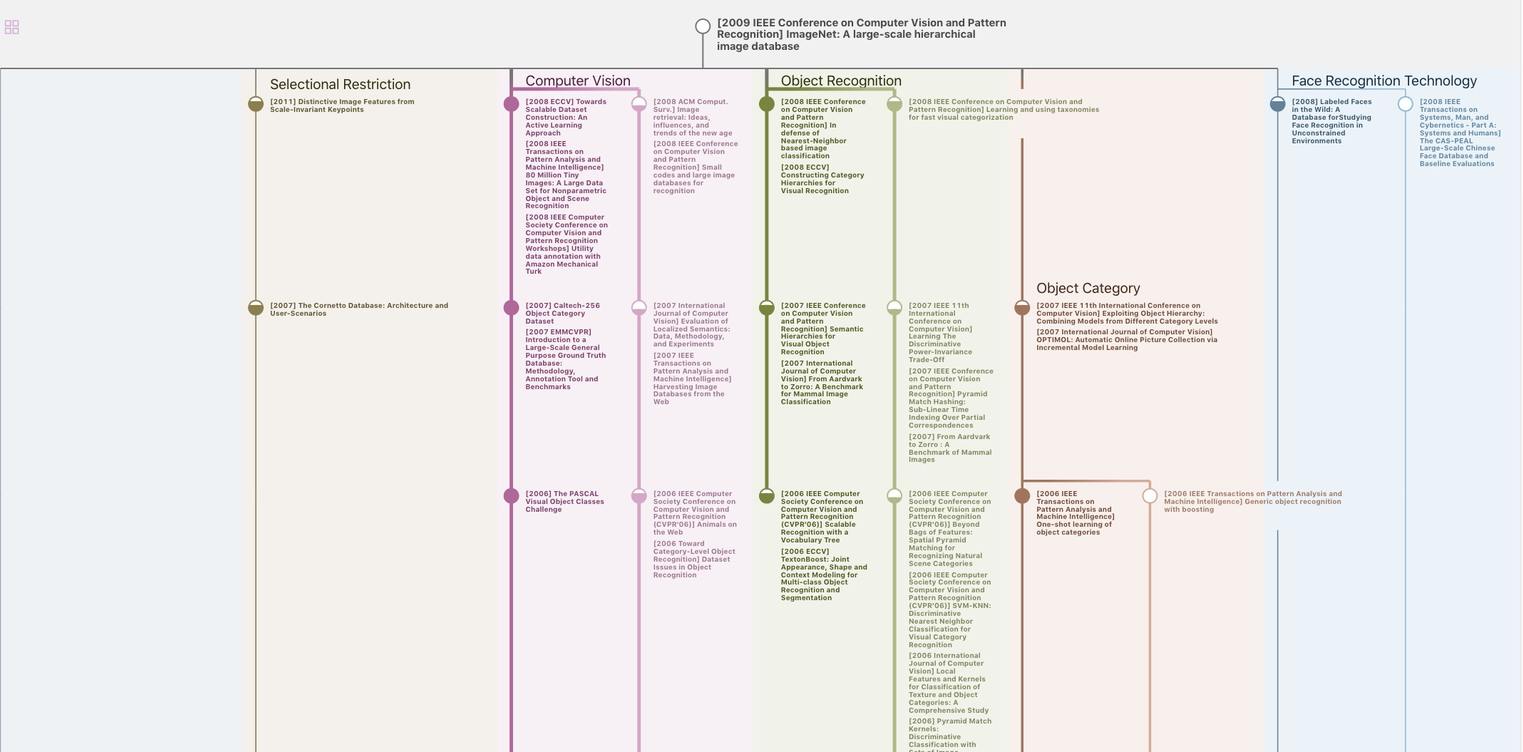
生成溯源树,研究论文发展脉络
Chat Paper
正在生成论文摘要